We use Apache Airflow for the automation and orchestration of model deployment, training, and feature engineering steps. It is a model lifecycle management tool.
Data Engineer Team Lead at Unibank
Can be used with multiple systems and servers, Kubernetes systems, and dashboard systems
Pros and Cons
- "The product is stable."
- "There is a need for more features on experimental evolution steps."
What is our primary use case?
How has it helped my organization?
We have an integration with Apache Airflow in our portal for messaging. We use group and transformation data from Redshift to Tesco, and then create a call flow to the router. This is a source of data leakage, such as data engineering and machine learning, especially in a HIPAA environment. We need to check the evolution steps in the pipeline. In production, we only have two cases. Sometimes, we need customer data not in the database, which we get from object storage. The call flow from Redshift to Tesco involves transforming the data and then generating it with the router or Kibana router for the policy. The data is then transformed and sent to the dashboard or data warehouse.
What needs improvement?
Airflow is a pipeline for transferring code by clients, but for experimental model experiments, Apache Airflow does not have any solution. There is a need for more features on experimental evolution steps.
For how long have I used the solution?
I have been using Apache Airflow for one and a half years.
Buyer's Guide
Apache Airflow
April 2025
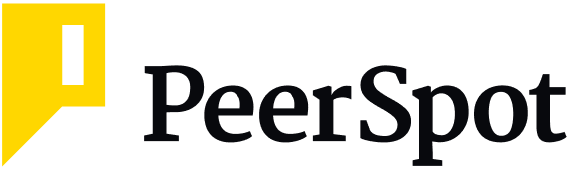
Learn what your peers think about Apache Airflow. Get advice and tips from experienced pros sharing their opinions. Updated: April 2025.
849,190 professionals have used our research since 2012.
What do I think about the stability of the solution?
The product is stable. I rate the solution’s stability an eight out of ten.
What do I think about the scalability of the solution?
20 users are using this solution in our organization. I rate the solution’s scalability an eight out of ten.
How was the initial setup?
The initial setup is not complex and can be done by two people. However, open-source prime solutions have some difficulties. We can schedule Apache Airflow on Kubernetes. Space limitations and installation issues may arise, as we do not have full control over Kubernetes cluster resources, and our administration is limited. I rate the initial setup a six out of ten, where one is difficult, and ten is easy.
What other advice do I have?
I recommend Apache Airflow because it is still profitable and can be used with multiple systems and servers, Kubernetes systems, and dashboard systems. You can use it to get social media and other data, but it can be expensive. Overall, I rate the solution a nine out of ten.
Which deployment model are you using for this solution?
On-premises
Disclosure: I am a real user, and this review is based on my own experience and opinions.
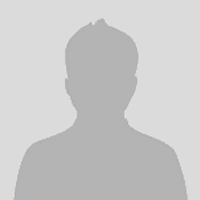
IT Professional at Freelance
Equips users with a comprehensive feature set for managing complex workflows and has a responsive technical support team
Pros and Cons
- "Airflow integrates well with Cloudera and effectively supports complex operations."
- "One area for improvement would be to address specific functionalities removed in recent updates that were previously useful for our operations."
What is our primary use case?
We use the product for scheduling and defining workflows. It helps us extensively to manage complex workflows within Cloudera's ecosystem, particularly for handling and processing data.
How has it helped my organization?
The solution has been beneficial in automating and managing our data workflows efficiently. It has integrated well with our Cloudera environment, enabling us to handle complex workflows with greater ease and reliability.
What is most valuable?
The solution's most valuable feature is its ability to run workflows without saving changes. It allows us to execute tasks without permanently altering our configurations, which is useful for temporary adjustments and testing.
What needs improvement?
One area for improvement would be to address specific functionalities removed in recent updates that were previously useful for our operations.
Additional features that could enhance the product include more flexibility in parameterization and improved tools for managing and debugging workflows.
For how long have I used the solution?
I have been working with Airflow for approximately a year and a half, focusing on the current version for the past eight months.
What do I think about the stability of the solution?
The product has been stable in our environment.
What do I think about the scalability of the solution?
The product is scalable.
How are customer service and support?
The technical support team has been responsive and helpful. They addressed issues related to removed functionalities and ensured critical features were restored in subsequent updates.
How would you rate customer service and support?
Positive
Which solution did I use previously and why did I switch?
We previously used Hortonworks but switched to Cloudera CDP. We also used other Cloudera tools but found Airflow to be a better fit for our current needs due to its capabilities in workflow management.
How was the initial setup?
The initial setup was complex due to the integration with various data sources and configuration requirements, but once properly set up, it has proven effective.
What about the implementation team?
The implementation was carried out with guidance from Cloudera's support team, who provided valuable assistance in configuring the solution to meet our requirements.
Which other solutions did I evaluate?
We evaluated other data workflow solutions but found Airflow the most suitable due to its integration with Cloudera and comprehensive feature set for managing complex workflows.
What other advice do I have?
Airflow integrates well with Cloudera and effectively supports complex operations. However, users should be aware of changes in functionality between versions and plan accordingly.
Overall, I rate it a nine out of ten.
Disclosure: My company has a business relationship with this vendor other than being a customer: Partner
Last updated: Sep 19, 2024
Flag as inappropriateBuyer's Guide
Apache Airflow
April 2025
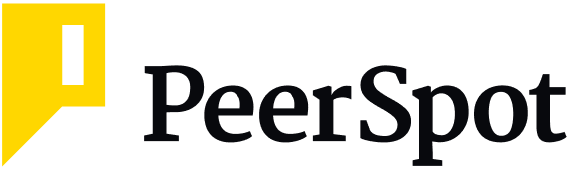
Learn what your peers think about Apache Airflow. Get advice and tips from experienced pros sharing their opinions. Updated: April 2025.
849,190 professionals have used our research since 2012.
Enterprise Architect at kosakya
An open-source solution that has limitations in processing too many jobs
Pros and Cons
- "I worked on a project at a leading German bank for two years, successfully migrating large applications with hundreds of jobs."
- "Apache Airflow improved workflow efficiency, but we had to find solutions for large workflows. For instance, a monthly workflow with 1200 jobs had to be split into three to four pieces as it struggled with large job numbers. Loading a workflow with 500 jobs could take 10 minutes, which wasn't acceptable."
What needs improvement?
Apache Airflow improved workflow efficiency, but we had to find solutions for large workflows. For instance, a monthly workflow with 1200 jobs had to be split into three to four pieces as it struggled with large job numbers. Loading a workflow with 500 jobs could take 10 minutes, which wasn't acceptable.
The most important feature Apache Airflow lacks is support for external configuration files. All classical schedulers like Control-M or Automic allow you to load workflow definitions from YAML, XML, or JSON files, but the tool requires you to write Python programs. Airflow only supports external configuration for variables, not for workflows. To address this, I created a YAML configuration file that I converted into Python programs, but this functionality is missing from Apache Airflow itself.
All of its competitors have this feature. In Control-M, Automic, and IBM's scheduler, you can load workflows from XML, JSON, or YAML files.
For how long have I used the solution?
I've been familiar with Apache Airflow for about three to four years. I worked on a project at a leading German bank for two years, successfully migrating large applications with hundreds of jobs. However, the leading German bank paused its migration strategy due to issues with the team in India. They're likely waiting for version 3, which is expected next year.
What do I think about the stability of the solution?
I rate the tool's stability a nine out of ten.
What do I think about the scalability of the solution?
I rate the product's scalability a seven out of ten.
How are customer service and support?
Apache Airflow doesn't have its own technical support.
How was the initial setup?
I've been involved in all aspects of Airflow deployment, including building infrastructure using Kubernetes and containers. We faced challenges migrating from enterprise schedulers like Control-M and IBM's scheduler to Airflow, as it lacked some functionality. I had to implement extra features and extensions to support things like individual calendars.
What's my experience with pricing, setup cost, and licensing?
Apache Airflow is open-source and free. Hyperscalers like Google (with Composer), Azure, and AWS offer managed Airflow services.
What other advice do I have?
I recommend Apache Airflow because it's open-source, but you must accept its limitations. However, I wouldn't recommend it to companies in biomedical, chemistry, or oil and gas industries with large workflows and thousands of jobs. For example, genomic analysis at an American multinational pharmaceutical and biotechnology corporation involved workflows with around twenty thousand jobs, which Airflow can't handle. Special schedulers are needed for such cases, as even classical schedulers like Control-M and Automic aren't suitable.
I rate the overall solution a seven out of ten.
Disclosure: I am a real user, and this review is based on my own experience and opinions.
Last updated: Sep 6, 2024
Flag as inappropriateHead of Big Data Department at IBA Group
Used for the orchestration of data pipelines, but it should have better integration with cloud platforms
Pros and Cons
- "Since it's widely adopted by the community, Apache Airflow is a user-friendly solution."
- "Apache Airflow should have better integration with cloud platforms."
What is our primary use case?
We use Apache Airflow for the orchestration of data pipelines.
What is most valuable?
Since it's widely adopted by the community, Apache Airflow is a user-friendly solution.
What needs improvement?
Apache Airflow should have better integration with cloud platforms.
For how long have I used the solution?
I have been using Apache Airflow for a couple of years.
What do I think about the stability of the solution?
Apache Airflow is not a stable solution.
What do I think about the scalability of the solution?
Around ten people are using the solution in our organization.
How was the initial setup?
The solution's initial setup is difficult and should be done by an experienced person.
What's my experience with pricing, setup cost, and licensing?
Apache Airflow is a cheap solution.
What other advice do I have?
The solution is deployed on the cloud in our organization. Before choosing Apache Airflow, users should try cloud-native services first.
Overall, I rate the solution a seven out of ten.
Disclosure: I am a real user, and this review is based on my own experience and opinions.
Senior Data Engineer at a photography company with 11-50 employees
A tool that needs to improve its complex initial setup and limited integration capabilities but can be useful in workflow automation
Pros and Cons
- "Apache Airflow is useful for workflow automation, making it capable of automating pipelines, data pipelines, and data warehouse processes."
- "The problem with Apache Airflow is that it is an open-source tool. You have to build it into a Kubernetes container, which is not easy to maintain, and I find it to be very clunky."
What is our primary use case?
Apache Airflow is useful for workflow automation, making it capable of automating pipelines, data pipelines, and data warehouse processes. I don't have a strong need for Apache Airflow because I do everything with a dbt or data build tool since it has its own integrated workflow process.
I use Fivetran to synchronize my data. I don't need to do any automation on that and don't have any need for workflow automation. I have everything I need.
How has it helped my organization?
We were experimenting with the solution. We never reached the point where we would deploy the solution in the production capacity.
What needs improvement?
The problem with Apache Airflow is that it is an open-source tool. You have to build it into a Kubernetes container, which is not easy to maintain, and I find it to be very clunky.
Additionally, there is room for improvement with DAGs. I had a very hard time building DAGs in Apache Airflow. I decided to use Astronomer, which is on top of Apache Airflow and is supposed to make your life easier. The best part of the solution is the third-party add-on which is Astronomer.
It would be a very nice tool if it could have been an entirely cloud-based solution. Apache Airflow is not so nice when you have a hybrid setup, such as half is on-premises and half of it is on a cloud environment. It should integrate better with the outside world.
For how long have I used the solution?
I have been using Apache Airflow for a couple of months.
What do I think about the stability of the solution?
I have no opinion on the solution's stability. The solution did not get to a production capacity. I couldn't even do file processing with Apache Airflow. None of the engineers could actually help me set up Apache Airflow. I had to give up on the product. Just buy a product that works, and you will be done with it.
How was the initial setup?
The initial setup was complex to deploy on the cloud. Installing the software is very difficult. The documentation is very bad. There is no installer where you can press a button, and it does everything for you. One may need a couple of engineers to install the solution, which is an issue with open-source tools. Price-wise, the software falls on the cheaper side. With Apache Airflow, one may spend much more on engineers.
The solution is deployed purely on the cloud.
What was our ROI?
I didn't experience any ROI using the solution. I could do everything without Apache Airflow since it would have been just a money pit.
What other advice do I have?
I suggest others not use Apache Airflow. If you use Apache Airflow, you will waste your time unless you have a bunch of engineers who already know about the solution.
If you cannot write a DAG within two hours of starting the process, then forget about the tool, and it would be better if you tried to find something else.
Overall, if the tool was working properly, it would be very good, but unfortunately, it is not.
Overall, I rate the solution a five out of ten.
Disclosure: I am a real user, and this review is based on my own experience and opinions.
Software engineer at Naver Corp
Convenient, easy to learn, has a simple UI, and has a huge user base
Pros and Cons
- "The UI is very simple and easy to learn."
- "The documentation must be improved."
What is our primary use case?
My team works on commerce services. We use Airflow to synchronize user information or product information from other services. We use the tool for automating data pipelines. We store user history about API calls and show it on a statistics page, like daily or real-time statistics. We use the solution to aggregate API user's data.
What is most valuable?
Kubernetes from the batch application is the most useful to my team. It uses Python. It is simple. There are not many learning costs. We're using the scheduler. We don't need to care about the batch job every day. We just need to notice when the alerts are firing. It is convenient for us. The product supports many other services, like Kubernetes. I saw some custom applications and programs. The solution integrates very well with other products.
What needs improvement?
The documents do not precisely define the function of the operators. I had to do some experiments to understand the function of the operators. The documentation must be improved. Some parts of the documentation do not precisely explain the parameters and functions. We often need to do experiments to understand how they work.
For how long have I used the solution?
I have been using the solution for one and a half years.
What do I think about the stability of the solution?
I rate the tool’s stability a nine out of ten.
What do I think about the scalability of the solution?
I rate the tool’s scalability a six or seven out of ten. We haven’t horizontally scaled the solution. At least 20% of the teams in my organization are using Airflow to do some batch jobs. There are around 300 users.
How was the initial setup?
I rate the ease of setup an eight out of ten. The product is deployed on the cloud. We release Airflow on Kubernetes. The deployment takes less than five minutes. We use a deployment tool made by our company to deploy the solution.
Which other solutions did I evaluate?
I am also using Apache Kafka.
What other advice do I have?
I will recommend the product to others. The UI is very simple and easy to learn. There are a lot of users of the product. We can find information easily on Google. Overall, I rate the tool an eight out of ten.
Disclosure: I am a real user, and this review is based on my own experience and opinions.
Lead Data Scientist at MVola
An easy to implement and flexible solution
Pros and Cons
- "The solution is flexible for all programming languages for all frameworks."
- "Apache Airflow could be improved by integrating some versioning principles."
What is our primary use case?
Currently, I am a lead data scientist. Our primary use cases for Apache Airflow are for all orchestrations, from the basic big data lake to machine learning predictions. It is used for all the MLS processes. It is also used for some ELT, to transform, load, and export all big data from restricted, unrestricted, and all phase processes.
What is most valuable?
The user experience of Apache Airflow is good. The solution is flexible for all programming languages for all frameworks. I also value that it is used for monitoring. Apache Airflow helps to easily integrate data sources with other products.
What needs improvement?
Apache Airflow could be improved by integrating some versioning principles. Currently, we have to swap some tags in our flow. It would be interesting if we can check the product and version all of the product at the same time comparing what scripts have changed from last year to this year, or last month to this month.
For example, we have a flow for one project, to version it we need to check it one by one to identify which tags changed and which scripts changed. All of these need to be done manually.
For how long have I used the solution?
I have been using Apache Airflow for four months.
What do I think about the stability of the solution?
We have experienced some bugs in Airflow. For example, the solution did not mention all the errors regarding why a process did not work. We had to investigate to try and understand why it was not working.
What do I think about the scalability of the solution?
The solution is easy to scale. We have four people in our organization that use Airflow. One is dedicated to the solution, while the others can use it to adjust the flow of their jobs on their own.
How are customer service and support?
We do not use technical support. We are trained to resolve concerns on our own. If a problem is significant we could call support, however, there is a good developer community that uses Airflow that can help resolve the issue with us.
How would you rate customer service and support?
Positive
Which solution did I use previously and why did I switch?
Prior to using Airflow, I used Windows SSIS for three years. We made the switch because Windows SSIS uses the drag-and-drop concept, where Airflow requires coding. Also, Windows is orientated to Microsoft products and is not very flexible.
How was the initial setup?
I am a technician, so the initial setup is instinctive. Without experience, it would not be as simple. Experience with configurations with parameters is required. The documentation is good, however, it does not mention some features explicitly requiring some research.
I would rate the ease of implementation a three out of five.
What about the implementation team?
We have dedicated machine learning ops, so we manage all product deployment ourselves. The deployment takes about four days, including two days of administration.
Apache Airflow requires maintenance. It is very important to maintain all the source codes and all the data. We are looking for a platform that would facilitate the maintenance of the project.
What's my experience with pricing, setup cost, and licensing?
We use a community edition of Apache Airflow. It is open-source and free.
What other advice do I have?
Anyone considering Apache Airflow should make sure that they have a good team with experience, including some administration. A strong background will help to understand and exploit the strengths of the platform.
I would rate this solution a nine out of 10 overall.
Which deployment model are you using for this solution?
On-premises
Disclosure: I am a real user, and this review is based on my own experience and opinions.
Senior Software Engineer at a pharma/biotech company with 1,001-5,000 employees
Feature rich, open-source, and good for building data pipelines
Pros and Cons
- "I like the UI rework, it's much easier."
- "I would like to see it more friendly for other use cases."
What is our primary use case?
I'm a data engineer. In the past, I used Airflow for building data pipelines and to populate data warehouses. With my current company, it's a data product or datasets that we sell to biopharma companies.
We are using those pipelines to generate those datasets.
What is most valuable?
I like the UI rework, it's much easier.
I use XCom for derived variables that need to pass between tasks. I don't really tend to use it for passing data, but only for a derived variable. For example, I don't have to re-query something every time, with one-task uses. I use the JSON comp for overwriting certain parameters.
In our use cases, some of the inputs of the dataset are files that we pulled out of S3. Sometimes they need to re-do those files, but we don't need to change any logic, we just need to redo the bills. Rather than redeploying the code to point to a new S3 bucket, we overwrite it to point to a different S3 key.
I have read that there are many different workflow pipelining tools in the biotech space, such as Snakemake and Nextflow.
There is also a CWL plugin that we may look into at some point.
Eventually, we might have a use case where a researcher has a pipeline they run locally, and then we want to convert that to a DAG.
The CWL-Airflow plugin would be useful for that. This might be something to look into later. But that would be like months, or maybe a year from now.
What needs improvement?
I am using a Celery Executor and I find that it crashes and I can't see any logs. I can only assume that it's a memory issue and have to blindly restart until eventually, it starts up again.
One of the use cases is triggered by input rather than a batch process. For example, we receive a batch of data, it goes through tasks one, two, and three, and a new batch comes in, each subsequent task should be operating on just that data from the prior task.
I am used to working on it as the output gets written to a table and then the next task selects all from that upstream table. It could be coded where you are only writing the data for that portion of the task. It could handle state machines and state changes as opposed to the batch proxy.
I would like to see it more friendly for other use cases.
For how long have I used the solution?
In my current company, I just introduced it within the last couple of months. But I've used it at my prior two jobs as well.
We are using Version 2.0.1.
What's my experience with pricing, setup cost, and licensing?
We are using the open-source version of Apache Airflow.
What other advice do I have?
I usually create my own custom operators every time. We upgraded to 2.0, but I am not using any of the new features.
I haven't yet used DAG of DAGs or the new way of using Python functions in the Python operator yet. But we might use DAG of DAGs eventually.
I Love this solution and I would rate it a nine out of ten.
Which deployment model are you using for this solution?
Private Cloud
If public cloud, private cloud, or hybrid cloud, which cloud provider do you use?
Amazon Web Services (AWS)
Disclosure: I am a real user, and this review is based on my own experience and opinions.
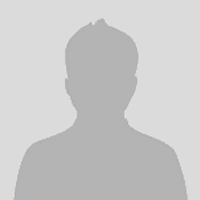
Buyer's Guide
Download our free Apache Airflow Report and get advice and tips from experienced pros
sharing their opinions.
Updated: April 2025
Product Categories
Business Process Management (BPM)Popular Comparisons
Informatica Intelligent Data Management Cloud (IDMC)
Camunda
Appian
Pega Platform
SAP Signavio Process Manager
Bizagi
IBM BPM
ARIS BPA
Bonita
Nintex Process Platform
AWS Step Functions
IBM Business Automation Workflow
Oracle BPM
KiSSFLOW
Boomi AtomSphere Flow
Buyer's Guide
Download our free Apache Airflow Report and get advice and tips from experienced pros
sharing their opinions.
Quick Links
Learn More: Questions:
- Which would you choose - Camunda Platform or Apache Airflow?
- When evaluating Business Process Management, what aspect do you think is the most important to look for?
- Camunda or Bonitasoft?
- Do you know of a solution which fulfills the requirements listed below?
- Looking for a BPMN tool that is easy to use and reasonably priced
- Which tool do you recommend for business process modeling only?
- Which is the best Workflow Automation Platform with microservices?
- RPA vs BPM: do they complement each other?
- What is the ROI of BPM solutions for a company which currently isn't using one?
- BPM Tools: What is the best alternative to Signavio?