The product handles reporting, data collection, and sharing, and serves as a database for applications.
Senior Data Architect at a pharma/biotech company with 1,001-5,000 employees
It's a scale out, MPP shared nothing architecture where there are multiple physical nodes.
Originally published at https://www.linkedin.com/pulse/microsoft-parallel-data-warehouse-pdw-stephen-c-folkerts
What’s the Difference Between Microsoft’s Parallel Data Warehouse (PDW) and SQL Server?
In this post, I’ll provide an in-depth view of Microsoft SQL Server Parallel Data Warehouse (PDW) and differentiate PDW with SQL Server SMP.
SQL Server is a scale up, Symmetric Multi-Processing (SMP) architecture. It doesn’t scale out to a Massively Parallel Processing (MPP) design. SQL Server SMP runs queries sequentially on a shared everything architecture. This means everything is processed on a single server and shares CPU, memory, and disk. In order to get more horse power out of your SMP box, or as your data grows, you need to buy a brand new larger more expensive server that has faster processors, more memory, and more storage, and find some other use for the old machine.
SQL Server PDW is designed for parallel processing. PDW is a scale out, MPP shared nothing architecture where there are multiple physical nodes. MPP architectures allow multiple servers to cooperate as one thus enabling distributed and parallel processing of large scan-based queries against large data volume tables. Most all data warehouse workload centric appliances leverage MPP architecture in some form of fashion. Each PDW node runs its own instance of SQL Server with dedicated CPU, memory, and storage. As queries go through the system, they are broken up to run simultaneously over each physical node. The primary benefits include the breath-taking query performance gains MPP provides, and the ability to add additional hardware to your deployment to linearly scale out to petabytes of data, without the diminishing returns of an SMP architecture.
The Grey Zone, When to use SQL Server PDW & What About Netezza, Teradata, Exasol, & a Hundred Others?
Often we’re not dealing with petabyte-scale data. Not even close, just in the terabytes, and we’re in a ‘grey zone’, where SQL Server SMP overlaps with PDW. Or the data is all relational and well structured, and there’s no Big Data business need like social media analysis, or fraud detection or combine structured and unstructured data from internal and external sources.
The capabilities of xVelocity Columnstore indexes and other in-memory capabilities and performance enhancements in SQL Server SMP should first be explored before recommending a PDW appliance. A combination of technologies, all native to SQL Server SMP may be the answer if you’re just dealing with relational data problems. The distinction between these sister products will always be blurry. And the underlying question of when to use SQL Server SMP versus PDW will persist, especially since SQL Server capabilities will keep clawing up into PDW territory, while PDW keeps growing. It is wise to understand the important differences for decision making.
Organizations demand results in near real time, and they expect their internal systems to match the speed of an Internet search engine to analyze virtually all data, regardless of its size or type. Once non-relational, unstructured or semi-structured data is thrown into the mix, you suddenly have a very different story. Business analysts struggle to figure out how to add the value of non-relational Hadoop data into their analysis. As a result, they’re held back from making faster more accurate data-driven decisions that are needed to compete in the marketplace. This is the current data challenge.
If you want to get into Netezza and Teradata, see my article Should I Choose Netezza, Teradata or Something Else?
Microsoft Parallel Data Warehouse (PDW)
Microsoft PDW is the rebuilt DATAllegro appliance with hardware and software designed together to achieve maximum performance and scalability. If you’d like to know more, see my article Microsoft PDW History, DATAllegro.
Query processing in PDW is highly parallelized. Data is distributed across processing and storage units called Compute Nodes. Each Compute Node has its own direct attached storage, processors, and memory that run as an independent processing unit. The Control Node is the brains of PDW and figures out how to run each T-SQL query in parallel across all of the Compute Nodes. As a result, queries run fast!
Microsoft SQL Server is foundational to PDW and runs on each Compute Node. PDW uses updateable in-memory clustered columnstore indexes for high compression rates and fast performance on the individual Compute Nodes.
The first rack of the PDW appliance is called the base rack. Every appliance has at least one base rack with 2 or 3 SQL Server Compute Nodes, depending on vendor hardware. As your business requirements change, you can expand PDW by adding scale units to the base rack. When the base rack is full, PDW expands by adding additional racks, called expansion racks, and adding scale units to them.
The base rack has two InfiniBand and two Ethernet switches for redundant network connectivity. A dedicated server runs the Control Node and the Management Node. A spare server ships in the rack for failover clustering. Optionally, you can add a second spare server.
With PDW’s MPP design, you don’t need to buy a new system to add capacity. Instead, PDW grows by adding to the existing system. PDW is designed to expand processing, memory, and storage by adding scale units consisting of SQL Server Compute nodes. By scaling out, you can easily expand capacity to handle a few terabytes to over 6 petabytes in a single appliance.
You don’t need to over-buy and waste storage that you don’t need, and if you under-buy you can quickly add more capacity if your data growth is faster than projected. When one rack is full, you can purchase another rack and start filling it with Compute nodes.
You also don’t need to migrate your data to a new system in order to add capacity. You can scale out without having to redesign your application or re-engineer the distribution mechanism. There is no need to migrate your data to a new system, and no need to restructure your database files to accommodate more nodes. PDW takes care of redistributing your data across more Compute nodes.
In-Memory xVelocity Clustered Columnstore Indexes Improve Query Performance
If MPP provides the computing power for high-end data warehousing, columnar has emerged as one of the most powerful architectures. For certain kinds of applications, columnar provides both accelerated performance and much better compressibility. Teradata, picked up columnar capabilities with its acquisition of Aster Data. HP acquired Vertica, which gave it a columnar MPP database.
PDW uses in-memory clustered columnstore indexes to improve query performance and to store data more efficiently. These indexes are updateable, and are applied to the data after it is distributed. A clustered columnstore index stores, retrieves and manages data by using a columnar data format, called a columnstore. The data is compressed, stored, and managed as a collection of partial columns, called column segments. Columns often have similar data, which results in high compression rates. In turn, higher compression rates further improve query performance because SQL Server PDW can perform more query and data operations in-memory. Most queries select only a few columns from a table, which reduces total I/O to and from the physical media. The I/O is reduced because columnstore tables are stored and retrieved by column segments, and not by B-tree pages.
SQL Server PDW provides xVelocity columnstores that are both clustered and updateable which saves roughly 70% on overall storage use by eliminating the row store copy of the data entirely. The hundreds or thousands of terabytes of information in your EDW can be built entirely on xVelocity columnstores. Updates and direct bulk load are fully supported on xVelocity columnstores, simplifying and speeding up data loading, and enabling real-time data warehousing and trickle loading; all while maintaining interactive query responsiveness.
Combining xVelocity and PDW integrates fast, in-memory technology on top of a massively parallel processing (MPP) architecture. xVelocity technology was originally introduced with PowerPivot for Excel. The core technology provides an in-memory columnar storage engine designed for analytics. Storing data in xVelocity provides extremely high compression ratios and enables in-memory query processing. The combination yields query performance orders of magnitude faster than conventional database engines. Both SQL Server SMP and PDW provide xVelocity columnstores.
Fast Parallel Data Loads
Loads are significantly faster with PDW than SMP because the data is loaded, in parallel, into multiple instances of SQL Server. For example, if you have 10 Compute Nodes and you load 1 Terabyte of data, you will have 10 independent SQL Server databases that are each compressing and bulk inserting 100 GB of data at the same time. This 10 times faster than loading 1 TB into one instance of SQL Server.
PDW uses a data loading tool called dwloader which is the fastest way to load data into PDW and does in-database set-based transformation of data. You can also use SQL Server Integration Services (SSIS) to bulk load data into PDW. The data is loaded from an off-appliance loading staging server into PDW Compute nodes. Informatica also works with PDW.
Scalable, Fast, and Reliable
With PDW’s Massively Parallel Processing (MPP) design, queries run in minutes instead of hours, and in seconds instead of minutes in comparison to Symmetric Multi-Processing (SMP) databases. PDW is not only fast and scalable, it is designed with high redundancy and high availability, making it a reliable platform you can trust with your most business critical data. PDW is designed for simplicity which makes it easy to learn and to manage. PDW’s PolyBase technology for analyzing Hadoop HDInsight data, and its deep integration with Business Intelligence tools make it a comprehensive platform for building end-to-end solutions.
Fast & Expected Query Performance Gains
With PDW, complex queries can complete 5-100 times faster than data warehouses built on symmetric multi-processing (SMP) systems. 50 times faster means that queries complete in minutes instead of hours, or seconds instead of minutes. With this performance, your business analysts can perform ad-hoc queries or drill down into the details faster. As a result, your business can make better decisions, faster.
Why Queries Run Fast in PDW
Queries Run in PDW on Distributed and Highly Parallelized data. To support parallel query processing, PDW distributes fact table rows across the Compute Nodes and stores the table as many smaller physical tables. Within each SQL Server Compute Node, the distributed data is stored into 8 physical tables that are each stored on independent disk-pairs. Each independent storage location is called a distribution. PDW runs queries in parallel on each distribution. Since every Compute Node has 8 distributions, the degree of parallelism for a query is determined by the number of Compute Nodes. For example, if your appliance has 8 Compute Nodes your queries will run in parallel on 64 distributions across the appliance.
When PDW distributes a fact table, it uses one of the columns as the key for determining the distribution to which the row belongs. A hash function assigns each row to a distribution according to the key value in the distribution column. Every row in a table belongs to one and only one distribution. If you don’t choose the best distribution column, it’s easy to re-create the table using a different distribution column.
PDW doesn’t require that all tables get distributed. Small dimension tables are usually replicated to each SQL Server Compute Node. Replicating small tables speeds query processing since the data is always available on each Compute Node and there is no need to waste time transferring the data among the SQL Server Compute Nodes in order to satisfy a query.
PDW’s Cost-Based Query Optimizer
PDW’s cost-based query optimizer is the brain that makes parallel queries run fast and return accurate results. A result of Microsoft’s extensive research and development efforts, the query optimizer uses proprietary algorithms to successfully choose a high performing parallel query plan. The parallel query plan, contains all of the operations necessary to run the query in parallel. As a result, PDW handles all the complexity that comes with parallel processing and processes the query seamlessly in parallel behind the scenes. The results are streamed back to the client as though only one instance of SQL Server ran the query.
PDW Query Processing
Here’s a look into how PDW query processing works ‘under the covers’. First, a query client submits a T-SQL query to the Control Node, which coordinates the parallel query process. After receiving a query, PDW’s cost-based parallel query optimizer uses statistics to choose a query plan, from many options, for running the user query in parallel across the Compute Nodes. The Control Node sends the parallel query plan, called the dsql plan, to the Compute Nodes, and the Compute Nodes run the parallel query plan on their portion of the data.
The Compute Nodes each use SQL Server to run their portion of the query. When the Compute nodes finish, the results are quickly streamed back to the client through the Control node. All of this occurs quickly without landing data on the Control node, and the data does not bottleneck at the Control node.
PDW relies on co-located data, which means the right data must be on the right Compute Node at the right time before running a query. When two tables use the same distribution column they can be joined without moving data. Data movement is necessary though, when a distributed table is joined to another distributed table and the two tables are not distributed on the same column.
PDW Data Movement Service (DMS) Transfers Data Fast
PDW uses Data Movement Service (DMS) to efficiently move and transfer data among the SQL Server Compute Nodes, as necessary, for parallel query processing. Data movement is necessary when tables are joined where the data isn’t co-located DMS only moves the minimum amount of data necessary to satisfy the query. Since data movement takes time, the query optimizer considers the cost of moving the data when it chooses a query plan.
Microsoft Analytics Platform System (APS)
See my article Microsoft Analytics Platform System (APS) for a more in-depth look at Microsoft APS.
These views are my own and may not necessarily reflect those of my current or previous employers.
Disclosure: I am a real user, and this review is based on my own experience and opinions.
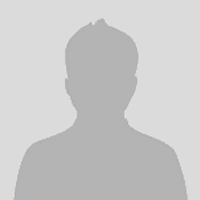
Senior Data Architect at a pharma/biotech company with 1,001-5,000 employees
Microsoft PDW History
Originally published at https://www.linkedin.com/pulse/microsoft-pdw-history-datallegro-stephen-c-folkerts
Microsoft SQL Server Parallel Data Warehouse (PDW) is the result of the DATAllegro acquisition in 2008 for roughly $238M. Datallegro was the invention of Stuart Frost to compete with Netezza which is now IBM PureData System for Analytics. Stuart Frost founded DATAllegro in 2003, was CEO of the company from the beginning, and specified the architecture of the product.Netezza came to market with a compelling value proposition. It leveraged an open source Postgres DBMS. It used an appliance business model to create a tightly integrated software and hardware stack, removing a significant area of complexity for DBAs and other system staff. It shifted to sequential I/O from the more typical random I/O in SMP architectures. This allowed the use of much larger and cheaper SATA disk drives and led to a highly competitive price/performance ratio. However, there was a significant flaw in Netezza's strategy. They created a highly proprietary hardware platform and, effectively, a proprietary software platform, with little of Postgres remaining.
Netezza secured its first few customers around the time DATAllegro was being founded. Looking at the Netezza architecture, Stuart Frost realized that there was an opportunity to create a similar value proposition while using a completely non-proprietary platform. Frost’s vision was to create a massively parallel DW appliance with an embedded, off-the-shelf open source Ingres DBMS running on Linux and using completely standard servers, networking and storage from major vendors.
Each server in DATAllegro ran a highly tuned copy of the Ingres DBMS and custom Java on SuSe Linux. These separate database servers were turned into a massively parallel, shared nothing database system that offered incredibly good performance, especially under complex mixed workloads.
Once Microsoft acquired DATAllegro in 2008, the first obvious task was to port the appliance over to the Microsoft SQL Server Windows stack. Microsoft internally went to work on this migration between the 2008 and 2010 period of time. It was known then as project ‘Madison’. In 2010, IBM ponied up $1.8 billion for DATAllegro's biggest competitor, Netezza.
Microsoft Parallel Data Warehouse (PDW)
See my article Microsoft Parallel Data Warehouse (PDW) for a more in-depth look at Microsoft SQL Server PDW.
Microsoft Analytics Platform System (APS)
See my article Microsoft Analytics Platform System (APS) for a more in-depth look at Microsoft APS.
These views are my own and may not necessarily reflect those of my current or previous employers.
Disclosure: I am a real user, and this review is based on my own experience and opinions.
Buyer's Guide
Microsoft Parallel Data Warehouse
November 2024
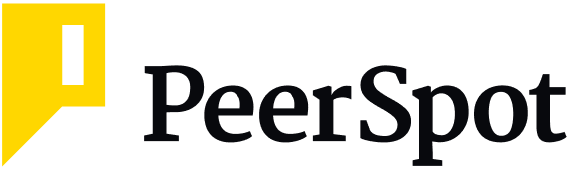
Learn what your peers think about Microsoft Parallel Data Warehouse. Get advice and tips from experienced pros sharing their opinions. Updated: November 2024.
816,406 professionals have used our research since 2012.
IT manager at Farmatrejd
An easy to setup solution with good reporting capabilities
Pros and Cons
- "Data collection and reporting are valuable features of the solution."
- "SQL installation is pretty tricky. The scalability and customer support also should be improved."
What is our primary use case?
What is most valuable?
Data collection and reporting are the tool's valuable features.
What needs improvement?
SQL installation is pretty tricky. Its scalability and customer support also should be improved.
For how long have I used the solution?
I have been using Microsoft Parallel Data Warehouse for more than 10 years.
What do I think about the stability of the solution?
The solution is stable.
What do I think about the scalability of the solution?
Currently, 54 individuals in our company use it.
How are customer service and support?
My interactions with customer service have not been quite satisfactory. The customer service team should possess a higher level of knowledge.
How was the initial setup?
The installation process can be time-consuming, and it does require some level of experience. For instance, understanding mixed mode and working with Windows logging, especially for beginners using the solution, might pose some challenges. The deployment process takes a bit longer, but it's manageable. However, comparing it to installing SQL, both tasks are relatively straightforward. Two people are required for deployment.
What about the implementation team?
The solution was implemented in-house.
Disclosure: PeerSpot contacted the reviewer to collect the review and to validate authenticity. The reviewer was referred by the vendor, but the review is not subject to editing or approval by the vendor.
Managing Partner at INFOLOJIK
A stable and expandable product that is easy to deploy and performs well
Pros and Cons
- "It is a very stable database."
- "The product must provide more frequent updates."
What is our primary use case?
We can use the solution for financial, banking, insurance, or retail sectors.
What is most valuable?
It is a very stable database.
What needs improvement?
The product must provide more frequent updates.
For how long have I used the solution?
I have been using the solution for 15 years.
What do I think about the stability of the solution?
I rate the stability a ten out of ten.
What do I think about the scalability of the solution?
The tool is scalable. I rate the scalability a ten out of ten. We have about 200 users.
Which solution did I use previously and why did I switch?
I work with Oracle, too. Data Warehouse is stable and expandable. The choice of solution depends on our customer’s requirements.
How was the initial setup?
The setup is very easy. The deployment takes one day. The deployment has a lot of steps. We need one or two engineers to deploy and maintain the tool.
What about the implementation team?
The deployment can be done in-house.
What was our ROI?
It's a quick solution for our customers.
What's my experience with pricing, setup cost, and licensing?
The price is normal. The license fee is paid yearly.
What other advice do I have?
I recommend the product to others. Overall, I rate the product a ten out of ten.
Disclosure: I am a real user, and this review is based on my own experience and opinions.
Managing Partner at Dynamis Informatica
User-friendly UI and good support
Pros and Cons
- "The UI is very simple and functional for my clients, most of the clients that use the solution are not experts."
- "The solution is expensive and has room for improvement."
What is our primary use case?
Our clients use Microsoft SQL Server Parallel Data Warehouse to create a separate database and build a data warehouse model. However, they do not use the appropriate product built into Microsoft SQL Server Parallel Data Warehouse for business intelligence. Instead, they create another database, link the model there, move the data from the online transaction database to the data warehouse to create a business intelligence database, and install a reporting tool on top of that. This is what our client does. We have experience with this process, as we are often the ones they call to transfer data from one database to the other, tune the database for performance, and create reports using a reporting tool.
How has it helped my organization?
In recent years, we have not been heavily involved with warehouse projects. Most of the time, we are involved with integrating different systems from different vendors. It is not possible to use one enterprise system to solve all of a company's problems, so they usually have three or four big systems and have difficulty integrating them. We spend most of our time creating integrations and transferring small chunks of information from one system to the other. When it comes to our house and business intelligence projects, they are usually limited to reporting; people just want to collect data, aggregate it, and view it from some reports. These days, Microsoft SQL Server Parallel Data Warehouse is the most commonly used tool for our clients with Power BI. However, the cost of Power BI is expensive, so the project often does not take off. I believe the biggest problem is that these business intelligence tools are usually too expensive.
What is most valuable?
The UI is very simple and functional for my clients, most of the clients that use the solution are not experts. They have fairly limited knowledge of computer usage, and they can create things with this solution. Microsoft Parallel Data Warehouse is very easy to use.
What needs improvement?
The solution is expensive and has room for improvement.
I believe the cost is the biggest issue. In Brazil, money is not easy to come by, and paying for this stuff is difficult. Most of my clients are moving to the cloud and purchasing some of the infrastructure there, but it is expensive and they don't always get the economic benefits the cloud can offer. They are creating the infrastructure from the cloud and paying a lot of money for it, and other costs are becoming problematic. Power BI is just one example. The process is the biggest barrier here, and sometimes lack of professional knowledge is also an issue. It is hard to find companies that really know what they are doing. The project I am involved in now is creating a data lake in the cloud for a big client. They hired another company, not mine, to create the project, but when we started the meetings I discovered that the big company they hired doesn't really know what they are doing and is still searching for professionals.
For how long have I used the solution?
I am currently using the solution.
How are customer service and support?
A license is required to access Microsoft technical support. Some of our clients use the support and it is fine.
What's my experience with pricing, setup cost, and licensing?
Technical support is an additional fee and is expensive.
The solution is expensive.
What other advice do I have?
I give the solution an eight out of ten.
Which deployment model are you using for this solution?
On-premises
Disclosure: I am a real user, and this review is based on my own experience and opinions.
CTO at Data Semantics Pvt Limited
A fairly stable solution that can be used to create an enterprise data warehouse for customers
Pros and Cons
- "Microsoft Parallel Data Warehouse integrates beautifully with other Microsoft ecosystem products."
- "The feature updates on the on-premise solution come very slowly, and it would be great if they came faster."
What is our primary use case?
On any given day, we use Microsoft Parallel Data Warehouse to create an enterprise data warehouse for our customers.
What is most valuable?
Microsoft Parallel Data Warehouse integrates beautifully with other Microsoft ecosystem products. The solution makes it easy to build the code and maintain it. At the same time, you get enough resources in the market if you have any queries or need support for the ecosystem.
What needs improvement?
The feature updates on the on-premise solution come very slowly, and it would be great if they came faster.
For how long have I used the solution?
I have been working with Microsoft Parallel Data Warehouse for the last ten years.
What do I think about the stability of the solution?
Microsoft Parallel Data Warehouse is a fairly stable solution.
I rate Microsoft Parallel Data Warehouse a nine out of ten for stability.
What do I think about the scalability of the solution?
Apart from a few use cases that we are not able to do, the solution's scalability is fairly decent.
I rate Microsoft Parallel Data Warehouse an eight out of ten for scalability.
How are customer service and support?
With Microsoft support and the ecosystem built around it, you can typically find help for your issues.
How would you rate customer service and support?
Positive
How was the initial setup?
Microsoft Parallel Data Warehouse's initial setup is fairly simple. I rate the solution an eight out of ten for ease of initial setup.
What about the implementation team?
A complete end-to-end setup of Microsoft Parallel Data Warehouse, which includes setting up the firewall and ensuring all the compliances and security features are met, could be a week-long activity. However, spinning up the resources is hardly a day's activity.
What's my experience with pricing, setup cost, and licensing?
Organizations with a huge data size typically require Microsoft Parallel Data Warehouse. No small customer would like to go and invest in such an infrastructure. The solution's pricing is fairly decent for organizations with huge data sizes.
What other advice do I have?
Overall, I rate Microsoft Parallel Data Warehouse a seven or eight out of ten.
Which deployment model are you using for this solution?
Hybrid Cloud
Disclosure: My company has a business relationship with this vendor other than being a customer: partner
A good interface and BI functionality, but scalability is an area that needs improvement
Pros and Cons
- "The most valuable feature is the business intelligence (BI) part of it."
- "This solution would be improved with an option for in-memory data analysis."
What is our primary use case?
I am a consultant and I assist in implementing this solution for our clients.
The projects that we are working on right now are related to finance, banking, and government.
What is most valuable?
The most valuable feature is the business intelligence (BI) part of it. BI includes decision trees and similar functionality. This happens post-building of the data warehouse when you start using the analysis features.
The user interface is definitely good.
What needs improvement?
This solution would be improved with an option for in-memory data analysis.
Scalability can be improved because there are other tools that perform better with very large datasets.
For how long have I used the solution?
We have been working with Microsoft Parallel Data Warehouse for more than five years.
What do I think about the stability of the solution?
This is a stable solution and we haven't had any issues. Even if we encounter issues, there is a lot of help available. If issues are identified then we will have a quick turnaround time for fixing them.
What do I think about the scalability of the solution?
There are some tools that are more scalable than this one, and the performance is better as well. This is an area that could use improvement, especially if you have a huge set of data.
We have about 50 analysts and another 30 or 40 regular users who work with this solution every day.
How are customer service and technical support?
We have been in contact with technical support in India and we are satisfied with them.
Which solution did I use previously and why did I switch?
We are using Pentaho Data Warehouse in addition to this solution. We select which one is implemented depending on the customer's request and what they want to do.
How was the initial setup?
The initial setup is straightforward. There is a lot of help available to assist with each step, so it's pretty easy.
The deployment took longer than expected because of continuous improvement and upgrades that were needed for the projects. Once we started and got a handle on how this solution could benefit our projects, we had to incorporate those new ideas. In total, it took between one and a half and two years to deploy.
What about the implementation team?
We implemented this solution with our in-house team.
What other advice do I have?
This is a solution that has good performance and I recommend it. The support from Microsoft is also another thing that makes the Parallel Data Warehouse a good option.
The biggest lesson that we have learned from using this solution is that customers are most interested in a quick project turnaround time, which is something that Parallel Data Warehouse provides.
This is a good solution but there is always room for improvement.
I would rate this solution a seven out of ten.
Disclosure: I am a real user, and this review is based on my own experience and opinions.
Team Leader at a tech services company with 201-500 employees
A stable and easy-to-deploy solution that enables users to manage large volumes of data
Pros and Cons
- "We can store the data in a data lake for a very low cost."
- "The product does not have all of the features that the native products have."
What is most valuable?
It's a very good solution because we can manage large volumes of data. We can store the data in a data lake for a very low cost. We can move the data in Parallel when we need more performance or decide to leave the data in the data lake. It's a very good product at the moment.
What needs improvement?
The product does not have all of the features that the native products have. If we want to do something advanced, we must use Data Factory and Databricks.
For how long have I used the solution?
I have been working with the product for about three to four years. I am leading a team. I don't work in depth with the solution.
What do I think about the stability of the solution?
The solution is pretty stable. Sometimes we have problems, but Microsoft manages and solves them quickly. We had some problems with Python and ETL processes inside Synapse. However, Microsoft solved the problem in less than an hour.
What do I think about the scalability of the solution?
The tool is scalable by design. Our customers are medium and enterprise-level businesses.
How are customer service and support?
The support is pretty good. Support could be faster. The team must be more knowledgeable about Azure.
How would you rate customer service and support?
Positive
How was the initial setup?
The initial setup is really easy. We don't need to do anything major. The deployment takes a few minutes.
The number of people required to deploy the tool depends on the number of environments we have. Usually, one architect is enough to deploy the product.
What about the implementation team?
Maintaining the product is easy because Microsoft does it for us. We don't need to do anything. It is upgraded by default.
What's my experience with pricing, setup cost, and licensing?
The pricing depends on the solution. If we decide to use a low-cost product, it is not expensive. The tool could be expensive if we need to manage a lot of data. The pricing depends on what we need to do. The tool might be expensive if we need high performance and want to manage a lot of data.
What other advice do I have?
All of the components related to the pipeline are included in Synapse. We have some ETL tools based on Data Factory and other advanced functions based on Databricks. Not all of the functionality is included in Synapse. It makes no sense to have the product without all of the functionality.
It is tricky to manage the storage mode of the data because if we don't read the documentation and don't create a good distribution of the data, we will have problems in the performance. If we do it, we will have no problem.
Overall, I rate the solution an eight out of ten.
Disclosure: My company has a business relationship with this vendor other than being a customer: Partner
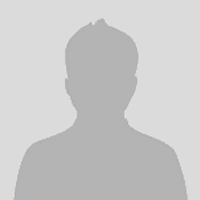
Buyer's Guide
Download our free Microsoft Parallel Data Warehouse Report and get advice and tips from experienced pros
sharing their opinions.
Updated: November 2024
Product Categories
Data WarehousePopular Comparisons
Oracle Exadata
VMware Tanzu Data Solutions
Apache Hadoop
Oracle Database Appliance
Buyer's Guide
Download our free Microsoft Parallel Data Warehouse Report and get advice and tips from experienced pros
sharing their opinions.
Quick Links
Learn More: Questions:
- Infobright vs. Exadata vs. Teradata vs. SQL Server Data Warehouse- which is most compatible with front end tools?
- Oracle Exadata vs. HPE Vertica vs. EMC GreenPlum vs. IBM Netezza
- When evaluating Data Warehouse solutions, what aspect do you think is the most important to look for?
- At what point does a business typically invest in building a data warehouse?
- Is a data warehouse the best option to consolidate data into one location?
- What are the main differences between Data Lake and Data Warehouse?
- Infobright vs. Exadata vs. Teradata vs. SQL Server Data Warehouse- which is most compatible with front end tools?
- What is the best data warehouse tool?
- Which Data Strategy solution have you used?