We use the product for extensive data analysis. It helps us analyze a huge amount of data and transfer it to data scientists in our organization.
Vice President at Goldman Sachs at a computer software company with 10,001+ employees
Stable product with a valuable SQL tool
Pros and Cons
- "The product’s most valuable feature is the SQL tool. It enables us to create a database and publish it."
- "At the initial stage, the product provides no container logs to check the activity."
What is our primary use case?
What is most valuable?
The product’s most valuable feature is the SQL tool. It enables us to create a database and publish it. It is a useful feature for us.
What needs improvement?
At the initial stage, the product provides no container logs to check the activity. It remains inactive for a long time without giving us any information. The containers could start quickly, similar to that of Jupyter Notebook.
For how long have I used the solution?
We have been using Apache Spark for eight months to one year.
Buyer's Guide
Apache Spark
March 2025
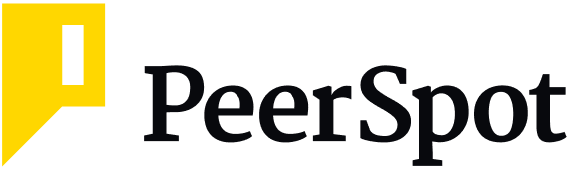
Learn what your peers think about Apache Spark. Get advice and tips from experienced pros sharing their opinions. Updated: March 2025.
839,422 professionals have used our research since 2012.
What do I think about the stability of the solution?
It is a stable product. I rate its stability an eight out of ten.
What do I think about the scalability of the solution?
We have 45 Apache Spark users. I rate its scalability a nine out of ten.
How was the initial setup?
The complexity of the initial setup depends on the kind of environment an organization is working with. It requires one executive for deployment. I rate the process an eight out of ten.
What's my experience with pricing, setup cost, and licensing?
The product is expensive, considering the setup. However, from a standalone perspective, it is inexpensive.
What other advice do I have?
I advise others to analyze data and understand your business requirements before purchasing the product. I rate it an eight out of ten.
Which deployment model are you using for this solution?
On-premises
Disclosure: I am a real user, and this review is based on my own experience and opinions.
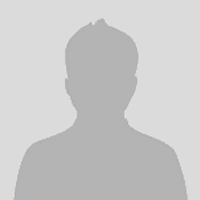
Lead Data Scientist at International School of Engineering
A flexible solution that can be used for storage and processing
Pros and Cons
- "The most valuable feature of Apache Spark is its flexibility."
- "Apache Spark's GUI and scalability could be improved."
What is our primary use case?
We use Apache Spark for storage and processing.
What is most valuable?
The most valuable feature of Apache Spark is its flexibility.
What needs improvement?
Apache Spark's GUI and scalability could be improved.
For how long have I used the solution?
I have been using Apache Spark for four to five years.
What do I think about the scalability of the solution?
Around 15 data scientists are using Apache Spark in our organization.
How was the initial setup?
Apache Spark's initial setup is slightly complex compared to other other solutions. Data scientists could install our previous tools with minimal supervision, whereas Apache Spark requires some IT support. Apache Spark's installation is a time-consuming process because it requires ensuring that all the ports have been accessed properly following certain guidelines.
What about the implementation team?
While installing Apache Spark, I must look at the documentation and be very specific about the configuration settings. Only then I'll be able to install it.
What's my experience with pricing, setup cost, and licensing?
Apache Spark is an expensive solution.
What other advice do I have?
I would recommend Apache Spark to other users.
Overall, I rate Apache Spark an eight out of ten.
Disclosure: I am a real user, and this review is based on my own experience and opinions.
Buyer's Guide
Apache Spark
March 2025
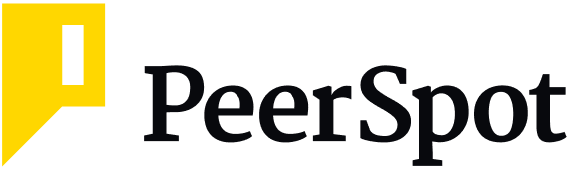
Learn what your peers think about Apache Spark. Get advice and tips from experienced pros sharing their opinions. Updated: March 2025.
839,422 professionals have used our research since 2012.
Quantitative Developer at a marketing services firm with 11-50 employees
Seamless in distributing tasks, including its impressive map-reduce functionality
Pros and Cons
- "The distribution of tasks, like the seamless map-reduce functionality, is quite impressive."
- "When using Spark, users may need to write their own parallelization logic, which requires additional effort and expertise."
What is our primary use case?
Predominantly, I use Spark for data analysis on top of datasets containing tens of millions of records.
How has it helped my organization?
I have an example. We had a single-threaded application that used to run for about four to five hours, but with Spark, it got reduced to under one hour.
What is most valuable?
The distribution of tasks, like the seamless map-reduce functionality, is quite impressive. For the user, it appears as simple single-line data manipulations, but behind the scenes, the executor pool intelligently distributes the map and reduce functions.
What needs improvement?
The visualization could be improved.
For how long have I used the solution?
I have been working with Apache Spark for only a few months, not too long.
What do I think about the stability of the solution?
I haven't faced any stability issues. It has been stable in my experience.
What do I think about the scalability of the solution?
When it comes to the scalability of Spark, it's primarily a processing engine, not a database engine. I haven't tested it extensively with large record sizes.
In my organization, quite a few people are using Spark. In my smaller team, there are only two users.
What about the implementation team?
In terms of maintenance, when the load hits around 95%, we need to prioritize scripts and analysis within the team.
We coordinate and prioritize based on the available resources. If there were self-service tools or better hand-holding for such situations, it would make things easier.
Which other solutions did I evaluate?
Currently, we extensively use pandas and Polaris. We are leveraging Docker and Kubernetes as a framework, along with AWS Batch for distribution. This is the closest substitute we have for Spark Distribution.
Both Docker and Kubernetes are more general-purpose solutions. If someone is already using Kubernetes and it's provided as a service, it can be used for special-purpose utilization, similar to Docker and Kubernetes.
In such cases, users may need to write the parallelization logic themselves, but it's relatively easy to onboard and start with a distributed load. Spark, on the other hand, is primarily used for special-purpose utilization. Users typically choose Spark when they have data-intensive tasks.
Another significant issue with Spark is its syntactics. For instance, if we have libraries like Panda or Polaris, we can run them single-threaded on a single core, or we can distribute them leveraging Kubernetes.
We don't need to rewrite that code base for Spark. However, if we are writing code specifically for Spark Executors, it will not be amenable to running it locally.
What other advice do I have?
I would recommend understanding the use case better. Only if it fits your use case, then go for it. But it is a great tool.
Overall, I would rate Apache Spark an eight out of ten.
Disclosure: I am a real user, and this review is based on my own experience and opinions.
Co-Founder at FORMCEPT Technologies
Offers good machine learning, data learning, and Spark Analytics features
Pros and Cons
- "The features we find most valuable are the machine learning, data learning, and Spark Analytics."
- "We've had problems using a Python process to try to access something in a large volume of data. It crashes if somebody gives me the wrong code because it cannot handle a large volume of data."
What is our primary use case?
We have built a product called "NetBot." We take any form of data, large email data, image, videos or transactional data and we transform unstructured textual data videos in their structured form into reading into transactional data and we create an enterprise-wide smart data grid. That smart data grid is being used by the downstream analytics tool. We also provide machine-building for people to get faster insight into their data.
What is most valuable?
We use all the features. We use it for end-to-end. All of our data analysis and execution happens through Spark.
The features we find most valuable are the:
- Machine learning
- Data learning
- Spark Analytics.
What needs improvement?
We've had problems using a Python process to try to access something in a large volume of data. It crashes if somebody gives me the wrong code because it cannot handle a large volume of data.
For how long have I used the solution?
I have been using Apache Spark for more than five years.
What do I think about the stability of the solution?
We haven't had any issues with stability so far.
What do I think about the scalability of the solution?
As long as you do it correctly, it is scalable.
Our users mostly consist of data analysts, engineers, data scientists, and DB admins.
Which solution did I use previously and why did I switch?
Before using this solution we used Apache Storm.
How was the initial setup?
The initial setup is complex.
What about the implementation team?
We installed it ourselves.
What other advice do I have?
I would rate it a nine out of ten.
Which deployment model are you using for this solution?
On-premises
Disclosure: My company has a business relationship with this vendor other than being a customer: Partner
Data Engineer at Berief Food GmbH
A useful and easy-to-deploy product that has an excellent data processing framework
Pros and Cons
- "The data processing framework is good."
- "The solution must improve its performance."
What is our primary use case?
Our customers configure their software applications, and I use Apache to check them. We use it for data processing.
What is most valuable?
The data processing framework is good. The product is very useful.
What needs improvement?
The solution must improve its performance.
For how long have I used the solution?
I have been using the solution for four to five years.
What do I think about the stability of the solution?
The tool is stable. I rate the stability more than nine out of ten.
What do I think about the scalability of the solution?
We have a small business. Around four people in my organization use the solution.
How was the initial setup?
The deployment was easy.
What about the implementation team?
The solution was deployed with the help of third-party consultants.
What other advice do I have?
Overall, I rate the product more than eight out of ten.
Which deployment model are you using for this solution?
On-premises
Disclosure: I am a real user, and this review is based on my own experience and opinions.
Director at Nihil Solutions
Stable and easy to set up with a very good memory processing engine
Pros and Cons
- "The memory processing engine is the solution's most valuable aspect. It processes everything extremely fast, and it's in the cluster itself. It acts as a memory engine and is very effective in processing data correctly."
- "The graphical user interface (UI) could be a bit more clear. It's very hard to figure out the execution logs and understand how long it takes to send everything. If an execution is lost, it's not so easy to understand why or where it went. I have to manually drill down on the data processes which takes a lot of time. Maybe there could be like a metrics monitor, or maybe the whole log analysis could be improved to make it easier to understand and navigate."
What is our primary use case?
When we receive data from the messaging queue, we process everything using Apache Spark. Data Bricks does the processing and sends back everything the Apache file in the data lake. The machine learning program does some kind of analysis using the ML prediction algorithm.
What is most valuable?
The memory processing engine is the solution's most valuable aspect. It processes everything extremely fast, and it's in the cluster itself. It acts as a memory engine and is very effective in processing data correctly.
What needs improvement?
There are lots of items coming down the pipeline in the future. I don't know what features are missing. From my point of view, everything looks good.
The graphical user interface (UI) could be a bit more clear. It's very hard to figure out the execution logs and understand how long it takes to send everything. If an execution is lost, it's not so easy to understand why or where it went. I have to manually drill down on the data processes which takes a lot of time. Maybe there could be like a metrics monitor, or maybe the whole log analysis could be improved to make it easier to understand and navigate.
There should be more information shared to the user. The solution already has all the information tracked in the cluster. It just needs to be accessible or searchable.
For how long have I used the solution?
I started using the solution about four years ago. However, it's been on and off since then. I would estimate in total I have about a year and a half of experience using the solution.
What do I think about the stability of the solution?
The stability of the solution is very, very good. It doesn't crash or have glitches. It's quite reliable for us.
What do I think about the scalability of the solution?
The scalability of the solution is very good. If a company has to expand it, they can do so.
Right now, we have about six or seven users that are directly on the product. We're encouraging them to use more data. We do plan to increase usage in the future.
How are customer service and technical support?
I'm a developer, so I don't interact directly with technical support. I can't speak to the quality of their service as I've never directly dealt with them.
Which solution did I use previously and why did I switch?
We did previously use a lot of different mechanisms, however, we needed something that was good at processing data for analytical purposes, and this solution fit the bill. It's a very powerful tool. I haven't seen other tools that could do precisely what this one does.
How was the initial setup?
The initial setup isn't too complex. It's quite straightforward.
We use CACD DevOps from deployment. We only use Spark for processing and for the Data Bricks cluster to spin off and do the job. It's continuously running int he background.
There isn't really any maintenance required per se. We just click the button and it comes up automatically, with the whole cluster and the Spark and everything ready to go.
What's my experience with pricing, setup cost, and licensing?
I'm unsure as to how much the licensing is for the solution. It's not an aspect of the product I deal with directly.
What other advice do I have?
We're customers and also partners with Apache.
While we are on version 2.6, we are considering upgrading to version 3.0.
I'd rate the solution nine out of ten. It works very well for us and suits our purposes almost perfectly.
Which deployment model are you using for this solution?
On-premises
Disclosure: My company has a business relationship with this vendor other than being a customer: Partner
Chief System Architect at a marketing services firm with 501-1,000 employees
Spark gives us the ability to run queries on MySQL database without pressurising our database
What is most valuable?
With spark SQL we've now the capabilities to analyse very large quantities of data located in S3 on Amazon at very low cost comparing other solution we checked.
We also use our own Spark cluster to aggregate data on near real time and save the result on MySQL database.
We've started new projects using the machine learning library ML.
How has it helped my organization?
Until Spark we didn't have the ability to analyse this quantity of data we're talking about two TB/hour. So we're now able to produce a lot of reports, and are also able to develop machine learning based analysis to optimize our business.
We've central access to every piece of data in the company including finance, business, debug etc. and the ability to join all this data together.
What needs improvement?
Spark is actually very good for batch analysis much more good than Hadoop, it's much simple, much more quicker etc., but it actually lacks the ability to perform real-time querying like Vertica or Redshift.
Also, it is more difficult for an end user to work with Spark than normal database. even comparing with analytic database like Vertica or Redshift.
For how long have I used the solution?
We're now using Spark-Streaming and Spark-SQL for almost 2 years.
What was my experience with deployment of the solution?
We're working on AWS so we need to have a managed environment. We've choose to go with a solution based on Chef to deploy and configure the spark clusters. Tip : if you don't have any devops you can use the ec2 script (provided by spark distro) to deploy cluster on amazon. We've tested it and work perfectly.
What do I think about the stability of the solution?
Spark Streaming is difficult to stabilize as you're always dependant to your stream flow. If you start to be late on the consumer you've a serious problem. We've encountered a lot of stability issue to configure it as expected
What do I think about the scalability of the solution?
It's linked to stability in our case it's takes time to evaluate what is the correct size of the cluster you need. It's very important to always add to you jobs monitoring to be able to understand what's the problem. We use datadog as monitoring platform
Which solution did I use previously and why did I switch?
Yes to make this job we've used a MySQL database. We switch because MySQL is not a scalable solution and we've reach it's limits.
How was the initial setup?
Setup a spark cluster can be difficult. it's related to your clustering strategy. There is 4 solution at least.
ec2 script : work only on Amazon AWS
Standalone : manually configuration (hard)
Yarn : to leverage your already existing Hadoop environment.
Mesos : to use with your other Mesos ready application
What about the implementation team?
We use Databricks as online DB ad hoc query. It's work on AWS as managed service, it manage for you the cluster creation, configuration and monitoring.
Give a notebook oriented user interface to query any data source using Spark: DB, Parquet, CSV, Avro etc...
Which other solutions did I evaluate?
Yes we've started to evaluate analytics databases : vertica, exasol, and other for all the them the price was an issue regarding the quantity of data we want to manipulate.
Disclosure: I am a real user, and this review is based on my own experience and opinions.
Partner / Head of Data & Analytics at Intelligence Software Consulting
Great for machine learning applications; good documentation available
Pros and Cons
- "Provides a lot of good documentation compared to other solutions."
- "The migration of data between different versions could be improved."
What is our primary use case?
We use Spark for machine learning applications, clustering, and segmentation of customers.
What is most valuable?
Apache provides a lot of good documentation compared to other solutions.
What needs improvement?
The migration of data between different versions could be improved.
For how long have I used the solution?
I've been using this solution for four years.
What do I think about the stability of the solution?
The solution is stable.
What do I think about the scalability of the solution?
The solution is scalable.
How are customer service and support?
If you pay for customer support then you get a quick and efficient response, otherwise the community support offers good help.
How was the initial setup?
The initial setup has been simplified over the past few years and is now relatively straightforward.
What's my experience with pricing, setup cost, and licensing?
Licensing costs depend on where you source the solution.
What other advice do I have?
This is a good solution for big data use cases and I rate it eight out of 10.
Disclosure: I am a real user, and this review is based on my own experience and opinions.
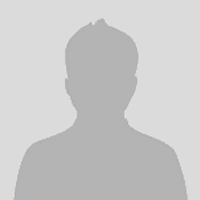
Buyer's Guide
Download our free Apache Spark Report and get advice and tips from experienced pros
sharing their opinions.
Updated: March 2025
Popular Comparisons
Amazon EMR
Cloudera Distribution for Hadoop
Spark SQL
IBM Spectrum Computing
Hortonworks Data Platform
Informatica Big Data Parser
IBM Db2 Big SQL
Buyer's Guide
Download our free Apache Spark Report and get advice and tips from experienced pros
sharing their opinions.
Quick Links
Learn More: Questions:
- Which is the best RDMBS solution for big data?
- Apache Spark without Hadoop -- Is this recommended?
- Which solution has better performance: Spring Boot or Apache Spark?
- AWS EMR vs Hadoop
- Handling real and fast data - how do BigInsight and other solutions perform?
- When evaluating Hadoop, what aspect do you think is the most important to look for?
- Should we choose InfoSphere BigInsights or Cloudera?