The main feature that we find valuable is that it is very fast. In terms of big data, the main feature is that the data is in so many different nodes. It goes through many data nodes so whenever we use the data, it enables us to parse the data from different data nodes.
Lead Consultant at a tech services company with 51-200 employees
The data storage capacity means we can inject somewhere in the user database in more efficient ways
Pros and Cons
- "The main feature that we find valuable is that it is very fast."
- "We use big data manager but we cannot use it as conditional data so whenever we're trying to fetch the data, it takes a bit of time."
What is most valuable?
What needs improvement?
We use big data manager but we cannot use it as conditional data so whenever we're trying to fetch the data, it takes a bit of time. There is some latency in the system and latency in the data caching. The main issue is that we need to design it in a way that data will be available to us very quickly. It takes a long time and the latest data should be available to us much quicked.
What do I think about the stability of the solution?
We don't have any problems with stability.
How are customer service and support?
I'm not the one who would contact their support if we needed it.
Buyer's Guide
Apache Spark
March 2025
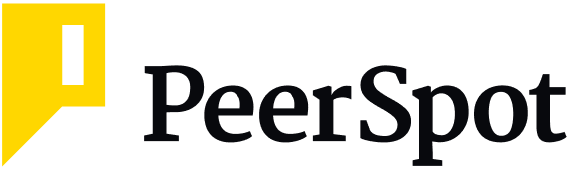
Learn what your peers think about Apache Spark. Get advice and tips from experienced pros sharing their opinions. Updated: March 2025.
839,422 professionals have used our research since 2012.
How was the initial setup?
The initial setup is straightforward.
What other advice do I have?
The advice that I would give to someone considering this solution is that the quality of data has key streaming capabilities like velocity. This means how quickly you are going to refer to the data. These things matter by designing the solution. We need to take these things out.
I would rate Apache Spark an eight out of ten.
To make it a ten they should improve the speed. The data storage capacity means we can inject somewhere in the user database in more efficient ways.
Disclosure: I am a real user, and this review is based on my own experience and opinions.
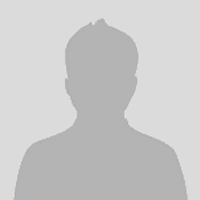
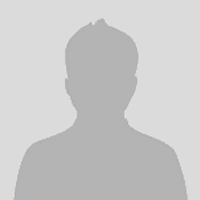
Buyer's Guide
Download our free Apache Spark Report and get advice and tips from experienced pros
sharing their opinions.
Updated: March 2025
Popular Comparisons
Amazon EMR
Cloudera Distribution for Hadoop
Spark SQL
IBM Spectrum Computing
Hortonworks Data Platform
Informatica Big Data Parser
IBM Db2 Big SQL
Buyer's Guide
Download our free Apache Spark Report and get advice and tips from experienced pros
sharing their opinions.
Quick Links
Learn More: Questions:
- Which is the best RDMBS solution for big data?
- Apache Spark without Hadoop -- Is this recommended?
- Which solution has better performance: Spring Boot or Apache Spark?
- AWS EMR vs Hadoop
- Handling real and fast data - how do BigInsight and other solutions perform?
- When evaluating Hadoop, what aspect do you think is the most important to look for?
- Should we choose InfoSphere BigInsights or Cloudera?