Amazon Kinesis is a service in AWS used for data ingestion. We pull data into Kinesis streams from various sources like OCS and then consume it for analysis and reporting.
AWS / Big Data Engineer at Waste Management, Inc.
Efficient Data Ingestion and good performance
Pros and Cons
- "I find almost all features valuable, especially the timing and fast pace movement."
- "The price is not much cheaper. So, there is room for improvement in the pricing."
What is our primary use case?
What is most valuable?
I find almost all features valuable, especially the timing and fast pace movement. The best part is that there is no loss of data.
What needs improvement?
The price is not much cheaper. So, there is room for improvement in the pricing.
For how long have I used the solution?
I have been working with Amazon Kinesis for about 18 months. I have hands-on experience using and providing it.
Buyer's Guide
Amazon Kinesis
November 2024
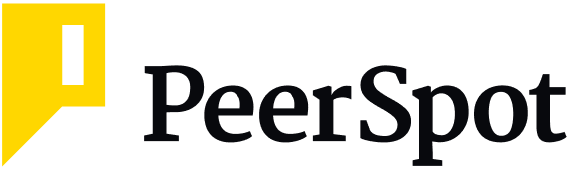
Learn what your peers think about Amazon Kinesis. Get advice and tips from experienced pros sharing their opinions. Updated: November 2024.
814,649 professionals have used our research since 2012.
What do I think about the stability of the solution?
The performance is quite good, and it's stable. Stability is okay. We didn't face any issues with stability.
What do I think about the scalability of the solution?
It is a scalable solution. It has been in continuous use for two years. So, there are over 20,000 end-users of this solution.
How are customer service and support?
The customer service and support are great. They have provided great assistance when needed.
How was the initial setup?
The initial setup is not straightforward; it has medium complexity. It took almost two weeks to complete the implementation and coding.
What about the implementation team?
Developers are required, along with release managers and other roles. There is a big team that works on the maintenance of the solution.
What's my experience with pricing, setup cost, and licensing?
The price is not much cheaper, but I can't say it's too expensive either. However, the customers do not have to pay any additional costs.
What other advice do I have?
I would definitely recommend using the solution. It's a great service, and it can be used wherever it's applicable in their model and architecture.
Overall, I would rate the solution an eight out of ten.
Which deployment model are you using for this solution?
Public Cloud
If public cloud, private cloud, or hybrid cloud, which cloud provider do you use?
Amazon Web Services (AWS)
Disclosure: My company has a business relationship with this vendor other than being a customer: Partner

Head of BI at Wind Mobility
The ability to have one single flow of inputting data from multiple consumers simplified our architecture
Pros and Cons
- "Amazon Kinesis has improved our ROI."
- "Something else to mention is that we use Kinesis with Lambda a lot and the fact that you can only connect one Stream to one Lambda, I find is a limiting factor. I would definitely recommend to remove that constraint."
What is our primary use case?
In terms of use cases, it depends of which component we're talking about, as we use three of the 4 components. The only one we don't use is the Video Streams.
Kinesis Data Stream is the module that we have been using the longest, essentially we use it to hold data which will be processed by multiple consumers. We have multiple data sources and we use Kinesis to funnel that data which is then consumed by multiple other consumers. We gather data coming from IoT devices, user phones, databases and a variety of other sources and then, as we have multiple consumers, we use Kinesis to actually gather the data and then we process it directly in Lambda, in Firehose, or in other applications.
How has it helped my organization?
Amazon Kinesis has absolutely improved our organization. Before Data Streams, we were using a couple of other solutions, including Talend and Pentaho, to move data around. Each of them were their own silos. So the ability to have one single flow of data from multiple consumers simplified our architecture a lot because you didn't need to copy or read the data multiple times, you just pull that data and then use multiple consumers. It actually simplified our architecture. It will also help us in the future when we have to build additional applications based on the same input data. We already have that data available. It will just be a matter of building the application itself. So it saves us a lot of time.
For Firehose, we perceive time-savings as a result of its incorporation. It takes you a couple of minutes to configure and it saves quite a lot of time in trying to get our information into the data lake.
Regarding Kinesis Analytics, we have real-time alarms and real-time data flows to populate other systems. For example, we populate Salesforce using a tumbling window implemented with Kinesis Data Analytics and Lambda. We also have alarms for things like knowing when someone is affecting our assets and we need to warn the operators in real-time. So Kinesis Analytics has actually given us the ability to track things in real-time that before we didn't have the ability to track.
Because we couldn't do that in the database we needed a component that had the ability to get the last window of data super quickly and if something was wrong, to notify and identify the failing record or the information that we wanted to trigger and with Lambda to notify the user. At certain points, when we had operational issues, we implemented alarms that have the key indicators to help us attack those issues before they grew and it was too late to attack them. So that has been essential for us.
What is most valuable?
I think that all Kinesis components have their own features and their own value. Starting from Data Streams, you have to have it as the data queue or else you would need to go to Kafka or another message broker (with higher implementation effort if your ecosystem is fully hosted in AWS already). I think that the solution they have put together in Kinesis is fairly easy to use. It is definitely a core component in any data architecture.
On the other hand, I find Firehose super simple and super useful for certain use cases. I wouldn't say it is as essential as Data Streams, but it is very handy if you want to just dump data. The connection between Data Streams and Firehose allows you to do that without worrying too much about performance and configuration. I find Firehose super simple to use for a very specific use case, but that use case is very common.
Kinesis Analytics is definitely more cutting edge. Out of Kinesis this is the most innovative part. We have used it for some alarms and for some batch processing in time windows. If we are talking about massive amounts of data, then you need to move to other solutions such as EMR or Glue for big data. If the amount of data is manageable and you want something to analyze on the fly, Kinesis Analytics is very appropriate and it gives you the ability to interact via SQL. So it makes your life easier if you want to develop a relatively self-contained application to do analytics on the fly.
I would say that Data Streams, in a matter of weeks, created a massive time-saving. Something that we haven't factored in is cost savings because we don't need to repeat the same data flow multiple times since each of those data flows are actually cost associated. We're talking about a couple of $100's per month, which is significant. In terms of time-savings here, we are in the scale of weeks.
What needs improvement?
In terms of what can be improved, I would say that within Data Streams, you have a variety of ways to interact with the data; you have the Kinesis client library, the KCL, and you have the Kinesis agent. When we were developing our architecture a couple years back, all the libraries to aggregate the data were very problematic. So the Kinesis Aggregator, which essentially improves the performance and cost by aggregating individual records into bigger one, is something that I found had a lot of room for improvement to make it a lot more refined. At the time I found a couple of limitations that I had to work around. So definitely on that side I found room for improvement.
Something else to mention is that we use Kinesis with Lambda a lot and the fact that you can only connect one Stream to one Lambda, I find is a limiting factor. I would definitely recommend to remove that constraint.
For how long have I used the solution?
I have been using Amazon Kinesis for over 2 years.
What do I think about the stability of the solution?
Kinesis is super stable. This is one of the only few components in AWS for which we have never had any issues with the stability.
What do I think about the scalability of the solution?
Regarding scalability, you wouldn't use Kinesis Analytics for huge, vast amounts of data or for complex processing. It's for relatively simple processing with not too much data. So I wouldn't say that it is infinitely scalable, it really depends on your application and the volume of data.
Right now I don't see us using more of Kinesis. It has a very clear role in our architecture and satisfies that perfectly well. This is one of the initial components that you build. In a roadmap that would be the first 10%. All our work is spent in different actions right now, but we don't have any plans to grow Kinesis further. We used to do some specific real-time analysis with Kinesis Analytics on a case by case basis.It's more on a per need basis.
In other companies we use Kafka, but we didn't replace it with Kinesis.
How was the initial setup?
The initial setup is relatively straight forward.
In terms of the initial setup of Kinesis Streams, is no big deal, you just choose the number of streams and assign a name to your application and that's pretty much it. The effort is in the applications that talk to Kinesis. I would say implementation took around six weeks. Deployment just took two people.
We have our own internal strategy which we started from scratch. So obviously we knew which components we would be deploying first. At the time we didn't use either CloudFormation or CodeBuild. So when we started, we didn't have these tools which we now use all the time for managing the architecture and CICD. But we didn't have it in the initial deployment.
What was our ROI?
Amazon Kinesis has improved our ROI. We obviously pay monthly for Kinesis but for us it is an enabler. We wouldn't have an architecture, or we'd have a terrible architecture, if Kinesis wasn't there.
For the data analytics component, we definitely saw that our ROI clearly improved. The alarms are something that we have actually implemented in very critical tasks when we had a company issue and that we have given visibility and a prompt response to the issues thanks to Kinesis Analytics. So that has definitely proven its ROI.
What's my experience with pricing, setup cost, and licensing?
In terms of the prices, I think it is a fair price. Kinesis Data Stream has a very fair price relative to the value that it provides. Same for Firehose. As for Kinesis Analytics, I find it on the more expensive side because it's a newer component, something fewer people use, and something more innovative, cutting edge, and more specific. I would say Analytics is more on the expensive side of the spectrum. I would say that Kinesis Analytics is the only one that I may complain about if you like low pricing.
Which other solutions did I evaluate?
Kafka is comparable to Data Streams, not to Kinesis Analytics. For Analytics on the fly, I can talk about doing Spark streaming, which is a lot more complex and you need to spend a lot more time setting it up, but it also has more capability in terms of the scaling, so I wouldn't say it's a one-to-one comparison.
I also used StreamSets in the past, where you can gather data and you can also do some transformations on the fly. But again it's not comparable one-to-one so I wouldn't use it for the same use cases.
What other advice do I have?
My recommendation for Data Streams is to do a deep dive into the documentation before implementing to avoid what we did at the beginning. You try to process record by record or push record by record into Kinesis and then realize that it is not cost effective or even efficient. So you need to know that you need to aggregate your data before you push it into Kinesis. So documenting yourself about the best practices in using Kinesis is definitely something I would recommend to anyone. For Kinesis Analytics, I was actually surprised at how easy it is to use an application with such power. I would say with a trial, users will realize that for for such a fairly complex application such as Kinesis Analytics, it is something that you can do very quickly with minimal resources and it gives you a lot of value for specific use cases.
On a scale of one to ten, I would give Amazon Kinesis a nine. I don't have much to complain about Kinesis.
Which deployment model are you using for this solution?
Public Cloud
If public cloud, private cloud, or hybrid cloud, which cloud provider do you use?
Amazon Web Services (AWS)
Disclosure: I am a real user, and this review is based on my own experience and opinions.
Buyer's Guide
Amazon Kinesis
November 2024
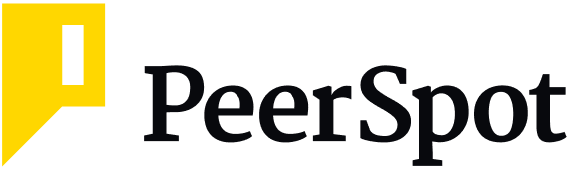
Learn what your peers think about Amazon Kinesis. Get advice and tips from experienced pros sharing their opinions. Updated: November 2024.
814,649 professionals have used our research since 2012.
Data is available when the solution is down, but the timeframe of retention support is too short
Pros and Cons
- "One of the best features of Amazon Kinesis is the multi-partition."
- "It would be beneficial if Amazon Kinesis provided document based support on the internet to be able to read the data from the Kinesis site."
What is our primary use case?
We are using Kinesis' third-party streaming engine. We are using the AWS cloud and are moving to Azure.
What is most valuable?
One of the best features of Amazon Kinesis is the multi-partition.
Another valuable feature of Kinesis is that when it is down, and in the backup stage, the data is still available.
What needs improvement?
Currently, Kinesis provides only seven days of retention support. It would be beneficial if this could be extended to upwards of 40 days or more.
In the next future release, I would like to see a library that is Java-compliant. It would be beneficial if Amazon Kinesis provided document-based support on the internet to be able to read the data from the Kinesis site.
For how long have I used the solution?
I have been using Amazon Kinesis for almost two years.
What do I think about the stability of the solution?
Amazon Kinesis is stable. I do not see any issues.
What do I think about the scalability of the solution?
The solution is scalable. We have 20 team members using Kinesis.
How are customer service and support?
We have not required support from Amazon.
How was the initial setup?
The initial setup of Amazon Kinesis is easy.
Which other solutions did I evaluate?
We have been looking for a streaming tool. We looked into Kafka, E-Hub, and Kinesis. Kafta is better than Kinesis as it has multiple cloud connectors. More features are available by default with Kafta.
What other advice do I have?
Overall, I would rate Amazon Kinesis a seven out of ten.
Which deployment model are you using for this solution?
Public Cloud
If public cloud, private cloud, or hybrid cloud, which cloud provider do you use?
Other
Disclosure: I am a real user, and this review is based on my own experience and opinions.
Chapter Lead - Data and Infrastructure (Head of Department) at a media company with 51-200 employees
Enables us to respond in real time; great auto-scaling feature
Pros and Cons
- "Great auto-scaling, auto-sharing, and auto-correction features."
- "Lacks first in, first out queuing."
What is our primary use case?
Our primary use case of this solution is as an intricate part of our data pipeline to deal with all of our big data problems. The traffic in our industry is highly volatile. At any given time we could have 10,000 users, and five minutes later it could be 100,000. We need systems fast enough to deal with that elasticity of demand, and the ability to deal with all the big data problems. Volume, velocity, ferocity, things like that. That's where we use the Kinesis platform. They have different iterations of it. The normal Kinesis Stream, is a little bit more manual, but we use that for our legacy technology, and for the more recent ones, we use Kinesis Firehose.
How has it helped my organization?
We dynamically change some of our product offerings based on user interaction. We can respond faster to user behavior, rather than waiting for the data to be at rest. We run some analytics models, and can then react in real time.
What is most valuable?
When it comes to Kinesis Firehose, the most valuable feature is the auto-scaling. It does auto-sharing, auto-correction, things like that and responds dynamically. Secondly, it innately has all the features of our reliable data pipeline, allowing you to store raw documents and transform data on the fly. When data comes into the stream through Firehose, we can see it and analyze every single object, keep the raw objects, carry out some transformations on it in flight, and then put it at rest. It allows us to do some real time analytics using Kinesis Analytics. We do anomaly detection in flight as well. We receive any changes with regards to user patterns and behaviors, in real time because Kinesis allows that.
What needs improvement?
They recently expanded the feature sets, but when we were implementing it, it could only deliver to one platform. I'm not sure where it's at now but multiple platforms would be beneficial. I'd also like to have some ability to do first in, first out queuing. If I put several messages into Firehose, there's no guarantee that everything will be processed in the order it was sent.
What do I think about the stability of the solution?
We've had no problems with stability and we implemented well over a year ago.
What do I think about the scalability of the solution?
The scalability of this solution is good. We are using it extensively with pretty much every single one of the flows.
How are customer service and technical support?
The technical support could be improved. They tend to send you back to the documentation.
Which solution did I use previously and why did I switch?
We switched to Kinesis because of the technical complexity of the previous solution. In the previous solution, Ops would write feeds on the SQS queue, and then it required physical machines to connect, pull the data, transform it and write. That required three or four different technologies. Kinesis has removed a lot of technical complexity to the architecture.
How was the initial setup?
The initial setup was straightforward. Both the user interface and the programmatic access is very intuitive. And again, it's not difficult, even non-technical people would be able to set it up. It took two people to implement. I was responsible for data architecture and we had a developer to transform the data inside. Deployment took less than an hour. The documentation was very helpful.
What was our ROI?
We've been able to drop our costs for ingesting data by about 60 to 70%.
Which other solutions did I evaluate?
We didn't evaluate anything else because no other product offered that type of fast solution at the time. Whatever we looked at added technical complexity to the architecture.
What other advice do I have?
It's important to think about how you are going to fix the end points that connect to your Kinesis files.
I would rate this solution a nine out of 10.
Disclosure: I am a real user, and this review is based on my own experience and opinions.
Senior Consultant at a tech vendor with 10,001+ employees
Useful for tracking silent events and capturing events
Pros and Cons
- "From my experience, one of the most valuable features is the ability to track silent events on endpoints. Previously, these events might have gone unnoticed, but now we can access them within the product range. For example, if a customer reports that their calls are not reaching the portal files, we can use this feature to troubleshoot and optimize the system."
- "I suggest integrating additional features, such as incorporating Amazon Pinpoint or Amazon Connect as bundled offerings, rather than deploying them as separate services."
What is our primary use case?
The solution provides real-time event streaming.
What is most valuable?
From my experience, one of the most valuable features is the ability to track silent events on endpoints. Previously, these events might have gone unnoticed, but now we can access them within the product range. For example, if a customer reports that their calls are not reaching the portal files, we can use this feature to troubleshoot and optimize the system.
What needs improvement?
I suggest integrating additional features, such as incorporating Amazon Pinpoint or Amazon Connect as bundled offerings, rather than deploying them as separate services.
For how long have I used the solution?
I have been using the product for a year.
What do I think about the scalability of the solution?
My company has four to five customers. The scalability of Amazon Kinesis has improved various data processing capabilities.
How are customer service and support?
I am happy with the tool's customer support.
How was the initial setup?
Amazon Kinesis' deployment is easy.
What's my experience with pricing, setup cost, and licensing?
The tool's pricing is cheap.
What other advice do I have?
If you're considering using Amazon Kinesis for the first time, I would advise exploring their services. It can be useful for capturing real-time events, greatly benefiting your solution. I rate it a seven out of ten.
Disclosure: My company has a business relationship with this vendor other than being a customer: customer/partner
Last updated: Apr 10, 2024
Flag as inappropriateCTO at ReNew
A cost-effectively processes and analyzes streaming data at any scale as a fully managed service
Pros and Cons
- "The management and analytics are valuable features."
- "Snapshot from the the from the the stream of the data analytic I have already on the cloud, do a snapshot to not to make great or to get the data out size of the web service. But to stop the process and restart a few weeks later when I have more data or more available of the client teams."
What is our primary use case?
To recover data and send it to the cloud. A few of our clients have Amazon Web Services and we use Kinesis to deploy the data to their mobiles and to their data processing system. Also to do data analytics.
What is most valuable?
The management and analytics are valuable features.
What needs improvement?
A snapshot from the stream of the data analytics I already have on the cloud. do a snapshot to stop the process and restart a few weeks later when I have more data or more availability of the client teams.
For how long have I used the solution?
I have been using Amazon Kinesis for two years.
What do I think about the stability of the solution?
The stability is a ten out of ten.
How was the initial setup?
We use cloud automation for deployment. We deploy the tags in minutes, and we can also use confirmation to test each part and test end-to-end use cases before we deploy them to the client. So we do everything with cloud automation, and it takes a few minutes to deploy a production environment.
What's my experience with pricing, setup cost, and licensing?
The solution is cheap.
What other advice do I have?
Overall, I rate the solution an eight out of ten.
Which deployment model are you using for this solution?
Public Cloud
Disclosure: My company has a business relationship with this vendor other than being a customer: Partner
Last updated: Feb 26, 2024
Flag as inappropriatePrincipal Data Engineer at a transportation company with 1,001-5,000 employees
A great managed service that's simple and easy to maintain
Pros and Cons
- "Everything is hosted and simple."
- "Could include features that make it easier to scale."
What is our primary use case?
Our primary use case of this solution is for a streaming bus architecture, we get events and they come in through Kinesis like a Jason event. It's usually a change to a database, but it can be any event such as in our application, which feeds into the Kinesis and then we have a Lambda that consumes them and then finally it puts those into a data warehouse which is the ultimate goal. So it's a near real-time data warehouse.
How has it helped my organization?
Instead of doing batch jobs of an ETL, like moving data into a data warehouse, we're now able to do it all through a continuous stream in Kinesis. It means that the data is more up-to-date in our data warehouse, it's more real time.
What is most valuable?
I've used Kafka in the past and Kinesis is a lot simpler. It's all hosted, it's nice it's really good. There aren't too many knobs and things to turn and ways to screw up. It's a pretty simple product and a lot easier to manage because it's hosted by AWS and it accomplishes what we need it to. The other nice thing is that we can make it available to external customers if they want to get a Kinesis feed of our things.
What needs improvement?
I would say that the solution probably has the capability to do sharding so that you can do a lot of things in parallel. I think that the way the sharding works could be simplified and include features that make it easier to scale in a parallel way.
For how long have I used the solution?
I've been using this solution for five years.
What do I think about the stability of the solution?
It's much easier to maintain than a Kafka cluster, but there were some nuances with it. I'd say maybe once a month or once every couple of months we'd get some weird things like AWS getting overwhelmed or the service was degraded for a few hours in a day. I guess that's a drawback of going with the fully managed service, it's just that it depends on the company keeping everything up. To me it's pretty stable, we would just get kind of slow once every month or once every couple of months. Overall I would say it's not perfect, but it was totally great, acceptable.
What do I think about the scalability of the solution?
You can do a bunch of shards, we only use one or a few shards and you can scale way up. It's way more scalable than we ever needed. And we were doing massive, millions of updates per minute. This is a backend service, so it's mostly used by developers and data engineers that were using Kinesis in our company, but our customers all benefited. Customers were sending send data through it, but they don't know that they are using Kinesis. We built the system and then they use it, so they don't know that under the covers it was consistent. We have five to 10 internal employees using it, but probably around 5,000 customers. Where I worked before, we used it basically everywhere. Probably 70% of all of our data was falling through Kinesis. Where I am now, we're just starting, so it's not yet being widely used. We plan to increase usage.
How are customer service and technical support?
When we had some of those slow downs, we used AWS support and I can't say that we had a great experience and they resolved the issues, but they looked into some of the flow downs and ultimately we just decided there was nothing we could do. It left me feeling there was something lacking on the technical support side. They didn't get to the bottom of all my issues, but the issues weren't bad enough to be unhappy about the product overall.
Which solution did I use previously and why did I switch?
We previously used Apache Kafka. We switched because we were already using Amazon for everything else so it made sense, and it was a nice managed solution that would be a lot easier to deal with. It also integrated well with Lambdas. The whole AWS ecosystem is nice to work with because everything integrates with each other.
How was the initial setup?
The initial setup is definitely straightforward because it's a managed service and you only get a few options when you set up a Kinesis stream. It's a lot less overwhelming than setting up a whole Kafka cluster or even if you've managed Kafka, there's still a lot of configuration required to get it up and running and all the choices you can make about topics and things. Kinesis is just much simpler. It only lets you configure what you need to configure. I'd say that kind of POC took about a week and then real production probably a month. We used Terraform for our implementation strategy, but I used CloudFormation in my past job to do that. The deployment was essentially running the CloudFormation template.
What was our ROI?
Compared to what we were doing with Kinesis or with Kafka, which was taking about 30% just to keep things together, with Kinesis I think we're probably saving tens of thousands, if not $100,000 per year.
What's my experience with pricing, setup cost, and licensing?
I would say pricing is really great. If pricing is an issue, I'd definitely recommend Kinesis because our Kinesis costs are under $1,000 a month. The product is super cost effective and it's the same with the licensing. Compared to Google Cloud and Azure, they're probably pretty similarly priced. I wouldn't say you're going to get a huge benefit going to Kinesis, but if you're considering using Kafka or another solution that's not hosted, it's not really worth all the effort when you could just go with a managed solution. It's a lot better cost-wise.
Which other solutions did I evaluate?
We took a look at Google cloud and Azure, they're Pub/Sub solutions, but not really in depth. Because we were already using Amazon, it just didn't make sense to use any other cloud provider.
What other advice do I have?
It's nice to deploy this with the Amazon goodness of Cloud Formation and Terraform, to have it all deployed in a repeatable way. I know that it's easy to go into the console and do it manually, but it's best to do infrastructure as code, in particular with Kinesis.
I would rate this solution a nine out of 10.
Which deployment model are you using for this solution?
Public Cloud
Disclosure: I am a real user, and this review is based on my own experience and opinions.
Senior DevOps Engineer at a tech services company with 201-500 employees
Provides near real-time data streaming at a consistent rate, but its cost is too high
Pros and Cons
- "Amazon Kinesis's main purpose is to provide near real-time data streaming at a consistent 2Mbps rate, which is really impressive."
- "We were charged high costs for the solution’s enhanced fan-out feature."
What is our primary use case?
Amazon Kinesis is a queuing or buffering system that we use as a central place to buffer the incoming data we receive from the source. The actual destination is open-faced. Amazon Kinesis is used as a buffer in between to decouple the workload.
What is most valuable?
Amazon Kinesis's main purpose is to provide near real-time data streaming at a consistent 2Mbps rate, which is really impressive.
What needs improvement?
The solution currently provides an option to retrieve data in the stream or the queue, but it's not that helpful. We have to write some custom scripts to fetch data from there. An option to search for data in the queue can really help us in our day-to-day operations.
Since the solution is a buffer system, you write to it and read from it. The readers are called consumers. If you want to run multiple consumers reading from the queue, you have to enable the enhanced fan-out feature on Amazon Kinesis. This enhanced fan-out feature is quite costly.
There was a point when we had a huge budget increase in one week just because of the enhanced fan-out feature. This feature does not provide any special out-of-the-box functionality. Hence, we struggle to optimize multiple consumers reading from a single queue. We were charged high costs for the solution’s enhanced fan-out feature.
For how long have I used the solution?
I have been using Amazon Kinesis for more than two years.
What do I think about the scalability of the solution?
The solution is pretty good in terms of scaling. Amazon Kinesis has shards, which are the instances or units that the solution spins up for you. Depending upon your account quota, you can spin up as many shards as you want. You can even raise a request to increase that quota, which will be done sooner. Overall, Amazon Kinesis is a really scalable solution.
Our team, consisting of four to five people, uses the solution extensively in our organization.
How are customer service and support?
We really struggle to get better support for Amazon Kinesis.
What's my experience with pricing, setup cost, and licensing?
Amazon Kinesis is an expensive solution.
What other advice do I have?
Amazon Kinesis is an AWS-managed service, just like S3 or EC. We don't have to deploy it; it is just there, and we spin it up. You must go to AWS' service page and click on Kinesis. Then, you can create it by clicking on Create and entering the name.
I would not recommend Amazon Kinesis to other users. Users can choose a cheaper alternative. They can use any other queuing system or in-house Kafka if they have a Kafka team. Amazon Kinesis provides near real-time read-and-write, but its cost is too high. Users can choose another option that provides the same functionality at less cost.
With Amazon Kinesis, you have to run a consumer who sees from Amazon Kinesis. AWS provides the Kinesis Client Library (KCL), which reads from the Kinesis stream. That library is also used in DynamoDB for data checkpointing. For example, if you have one day of data in Amazon Kinesis and started reading from 12 AM yesterday. The Kinesis Client Library (KCL) will check on the data in the DynamoDB. You get charged for the DynamoDB table out-of-the-box, along with Amazon Kinesis.
The DynamoDB table also costs a lot, which should not be the case. It is just read-and-write and is downloaded from the Kinesis Client Library (KCL). The DynamoDB table's cost should be very minimal, but that's not the case. The consumer is not optimal for efficient read-and-write, which further increases the cost. Both Amazon Kinesis and DynamoDB come into the picture.
Overall, I rate the solution a five or six out of ten.
Disclosure: I am a real user, and this review is based on my own experience and opinions.
Last updated: Apr 28, 2024
Flag as inappropriate
Buyer's Guide
Download our free Amazon Kinesis Report and get advice and tips from experienced pros
sharing their opinions.
Updated: November 2024
Product Categories
Streaming AnalyticsPopular Comparisons
Databricks
Confluent
Azure Stream Analytics
Apache Flink
Amazon MSK
Google Cloud Dataflow
Spring Cloud Data Flow
PubSub+ Platform
Apache Spark Streaming
Cloudera DataFlow
Apache Pulsar
IBM Streams
Buyer's Guide
Download our free Amazon Kinesis Report and get advice and tips from experienced pros
sharing their opinions.