The product helps us to manage Docker easily using automation.
Software Architect at AIOPS group
Helps to automate Docker management
Pros and Cons
- "The solution simplified deployment, making it more automated. Previously, Docker required manual configuration, often done by developers on their computers. However, with Google Kubernetes Engine, automation extends to configuration, deployment, scalability, and viability, primarily originating from Docker rather than Kubernetes. Its most valuable feature is the ease of configuration."
- "The tool's configuration features need improvement."
What is our primary use case?
What is most valuable?
The solution simplified deployment, making it more automated. Previously, Docker required manual configuration, often done by developers on their computers. However, with Google Kubernetes Engine, automation extends to configuration, deployment, scalability, and viability, primarily originating from Docker rather than Kubernetes. Its most valuable feature is the ease of configuration.
What needs improvement?
The tool's configuration features need improvement.
For how long have I used the solution?
I have been using the product for two years.
Buyer's Guide
Google Kubernetes Engine
December 2024
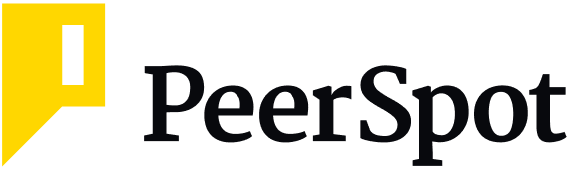
Learn what your peers think about Google Kubernetes Engine. Get advice and tips from experienced pros sharing their opinions. Updated: December 2024.
824,067 professionals have used our research since 2012.
What do I think about the stability of the solution?
We had some stability issues in the past. I rate the tool's stability a nine out of ten.
What do I think about the scalability of the solution?
I rate the solution's scalability a ten out of ten. Google Kubernetes Engine has around 100-200 users in my company.
How are customer service and support?
Google's support is good and fast. It's available 24/7.
How was the initial setup?
It will take some time for someone to get used to it, and there's a learning curve that shouldn't be skipped or neglected. But then, things will start to click, and you'll notice that the product is easy to deploy. The deployment setups are readily available from Google or Microsoft. You need to configure them, which can be done with these scripts and by automating your CI/CD processes. It's all interconnected with CI/CD.
What about the implementation team?
Google Kubernetes Engine can be deployed in-house.
What's my experience with pricing, setup cost, and licensing?
The tool's licensing costs are yearly.
What other advice do I have?
The inter-system communication, including the ports used, is all described within Docker. The product manages these Docker pieces and builds the bigger picture.
We integrate it as part of our DevOps script. It's all connected, with actions for the desktop, the CD Engine, and deployment on managed Kubernetes instances on Google Cloud. It's all automated and works well together.
I rate the overall product a nine out of ten.
Disclosure: I am a real user, and this review is based on my own experience and opinions.
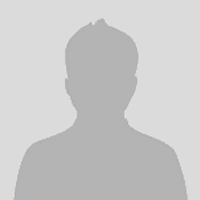
Senior DevOps/Build Engineer at Dataart
Easy to control and manage containers at all levels and is easy to create CD
Pros and Cons
- "The initial setup was very easy because it's like a Google platform as a service. It's just one button to set it up. The deployment took only a few minutes."
- "There are some security issues, but it might just be because we are not up to speed yet as much as we should be and so we haven't found it in the documentation yet. That's why I don't want to confuse this. Still, it could be a little bit easier to understand and implement."
What is our primary use case?
Kubernetes Engine is a platform that spins off applications so they can be run at scale at a high level.
We are currently migrating from on-premises to the cloud version.
How has it helped my organization?
First of all, it's easier to control and manage the containers at all levels. It becomes easy to create CD, or continuous delivery, and it's easier to scale.
What is most valuable?
Kubernetes Engines is easy to deploy and manage.
What needs improvement?
There are some security issues, but it might just be because we are not up to speed yet as much as we should be and so we haven't found it in the documentation yet. That's why I don't want to confuse this. Still, it could be a little bit easier to understand and implement.
They could also probably improve their monitoring features. We mostly don't use the graphical display. We use command lines, so this isn't a big issue for us.
For how long have I used the solution?
We've been using Google Kubernetes for about half a year.
What do I think about the stability of the solution?
For me, Kubernetes Engines was pretty stable.
What do I think about the scalability of the solution?
This is a very scalable product. We are increasing our use because our customer has a lot of products and he wants to migrate out of the application to cloud. He will use Kubernetes to do this.
Which solution did I use previously and why did I switch?
As I work with different customers, it was a customer decision. I have no choice. I used Amazon Container Services, ACS, before. It was not bad, but I like Kubernetes better.
How was the initial setup?
The initial setup was very easy because it's like a Google platform as a service. It's just one button to set it up. The deployment took only a few minutes.
What other advice do I have?
Management and deployment of a lot of containers could be very easy. It saves us time.
I think Kubernetes is really a fast developing and easy to use platform.
I would probably rate it as nine out of ten since it does have a little bit of room for improvement.
Disclosure: I am a real user, and this review is based on my own experience and opinions.
Buyer's Guide
Google Kubernetes Engine
December 2024
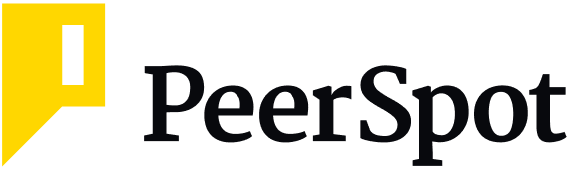
Learn what your peers think about Google Kubernetes Engine. Get advice and tips from experienced pros sharing their opinions. Updated: December 2024.
824,067 professionals have used our research since 2012.
Senior FullStack Developer/Engineer/Architect at Capitbrok
A highly scalable and stable solution with an intuitive dashboard
Pros and Cons
- "The product’s dashboard is very intuitive."
- "The solution does not have a visual interface."
What is our primary use case?
I use the solution to orchestrate different containers that need microservice architecture.
What is most valuable?
The product’s dashboard is very intuitive. The solution is very useful for monitoring.
What needs improvement?
The solution does not have a visual interface.
The solution could be improved by adding some visual drag-and-drop features.
For how long have I used the solution?
I have been using the solution for the past three years.
What do I think about the stability of the solution?
The solution is very stable.
What do I think about the scalability of the solution?
The solution is very scalable.
How was the initial setup?
The initial setup is a little bit complex.
What about the implementation team?
The deployment can be done in one to three months.
What's my experience with pricing, setup cost, and licensing?
The product is a little bit expensive.
What other advice do I have?
The solution is cloud-based. There are more tools available that are more visually intuitive. Overall, I rate the solution a nine out of ten.
Disclosure: I am a real user, and this review is based on my own experience and opinions.
Senior System Analyst at Evello System
A stable and flexible product with auto-scaling features
Pros and Cons
- "I am impressed with the product's output scaling."
- "I would like the solution to integrate with another Kubernetes product. I would also like it to monitor other platforms. It needs to also include scale-up container in the tool's next release."
What is most valuable?
I am impressed with the product's auto-scaling.
What needs improvement?
I would like the solution to integrate with another Kubernetes product. I would also like it to monitor other platforms. It needs to also include scale-up container in the tool's next release.
For how long have I used the solution?
I have been working with the product for four years.
What do I think about the stability of the solution?
I would rate the solution's stability a nine out of ten.
What do I think about the scalability of the solution?
I would rate the product's scalability a seven out of ten. It can scale up automatically. My company has 5 users for the solution.
How are customer service and support?
We rely on technical support from USA or India since it is not available in the local area. It is very difficult to get support.
How would you rate customer service and support?
Neutral
Which solution did I use previously and why did I switch?
I have used VMware's virtual machine before. We switched to the product since it offered flexibility and the ability to auto-scale.
How was the initial setup?
The product's setup is easy and I would rate it an eight out of ten. The deployment got completed in three hours. We relied on a DevOps engineer to complete the deployment and maintenance.
What about the implementation team?
We did the solution's deployment in-house.
What was our ROI?
The solution is worth its money.
What's my experience with pricing, setup cost, and licensing?
I would rate the solution's pricing a nine out of ten. The tool costs around 3000 dollars per month. There are no additional costs apart from these.
What other advice do I have?
I would rate the product an eight out of ten.
If public cloud, private cloud, or hybrid cloud, which cloud provider do you use?
Google
Disclosure: I am a real user, and this review is based on my own experience and opinions.
Principal Enterprise Architect at a tech vendor with 51-200 employees
A tool that offers resilience and high availability but needs to improve its stability
Pros and Cons
- "On the tip of a command, you can scale in or scale out, and it offers every robust platform to implement DevOps processes for your automation solutions. The product fully supports the IaC concept."
- "The product's stability is an area of concern where improvements are required."
What is our primary use case?
I have deployed the solution as a service into my private cloud, as well as into Azure infrastructure.
Google Kubernetes Engine is useful for cloud-native business applications, especially microservices-based architectures since business applications require scalability and resilience and must be highly available. Typically, Google Kubernetes Engine is used to deploy business applications and also to manage the integrations with the cloud services. There are a lot of SaaS solutions as service offerings provided by the Google Cloud Platform, so it helps with the integration to compile the solutions in the business space or, basically, the cloud-native space.
What is most valuable?
Google is the founder of Kubernetes. Google invented Kubernetes platform, and then later on, at some point in time, they had open-source scripts. Since Google started offering open-source scripts, a lot of other players in the market have adopted the same strategy for their products. Google Kubernetes Engine made a late entry into the market, but the good part that I like about it is that you have a seamless way of creating highly scalable and highly available solutions with it. On the tip of a command, you can scale in or scale out, and it offers every robust platform to implement DevOps processes for your automation solutions. The product fully supports the IaC concept.
What needs improvement?
The product's stability is an area of concern where improvements are required. Google Kubernetes Engine needs to mature more to be able to offer more stability compared to its competitors like Amazon and Microsoft, which have allowed for aggressive growth due to the area covered in terms of offering the complimentary services offered around Kubernetes. Google is behind even though it offers a robust platform. Google needs to offer more intensive services to fulfill the needs of customers and serve as a one-box or one-stop solution that covers everything enterprises need. Google needs to evolve more in terms of the richness of the services offered.
For how long have I used the solution?
I haven't worked full-fledged with Google Kubernetes Engine. since I have used it from an experimentation perspective. It is mainly Kubernetes that I have worked for for more than three years. Google Kubernetes is basically a managed service, but Kubernetes 1.24 is what I have extensively used.
What do I think about the stability of the solution?
Stability-wise, I rate the solution a seven out of ten.
What do I think about the scalability of the solution?
Scalability-wise, I rate the solution a six to seven out of ten.
My company's clients who use the solution are enterprise-sized businesses.
How are customer service and support?
I have no experience with the solution's technical support because I have not done any production-related work revolving around Google Kubernetes Engine. I believe that Google provides better technical support, but I don't have the relevant background to speak about it.
How was the initial setup?
I rate the product's initial setup phase a five or six on a scale of one to ten, where one is a difficult setup phase, and ten is an easy setup phase.
The concept revolving around Kubernetes is inherently complex when it comes to its setup phase. Kubernetes is not an easy domain to design and operate. The adoption of Kubernetes from a production perspective can be a bit of a complex task, not only for Google but for every other vendor in the world. The complexity is not much for a person who understands Kubernetes. With Google, considering its lack of maturity compared to the competitors, I will say the complexity during the setup phase may be a bit higher, based on which I rate the setup phase a six out of ten.
The solution is deployed on a public cloud by Google.
The solution can be deployed in a week since the major effort is in building the application, and getting the application to the production stage takes at least a week or so.
What was our ROI?
From an ROI perspective, the tool should be better, but since I have not done any production-grade application deployment on Google Kubernetes Engine, I won't be able to comment on the product's ROI part. As per my understanding, the overall Kubernetes ecosystem ensures a high ROI because it promotes the ability of a business, and that's what the platform is all about.
What's my experience with pricing, setup cost, and licensing?
I rate the product's price a six on a scale of one to ten, where one is low price and ten is high price. The product is competitively priced.
What other advice do I have?
I rate the overall product a seven out of ten.
Which deployment model are you using for this solution?
Public Cloud
If public cloud, private cloud, or hybrid cloud, which cloud provider do you use?
Google
Disclosure: My company has a business relationship with this vendor other than being a customer: Deployer
Engineer at SLT Visioncom Pvt Ltd
Offers GUI-based deployment and efficient integration features
Pros and Cons
- "The features are typical Kubernetes, but Google One offers a better GUI-based deployment. It's more sophisticated and integrates well with other services, providing a better customer experience."
- "There is room for improvement in this solution. For example, auto-scaling can be complex. We expect it to be easier to set up and manage, even for our customers."
What is our primary use case?
Mainly, we target SMEs for Kubernetes services. Currently, we have a few customers, and they expect more customized experiences.
What is most valuable?
The features are typical Kubernetes, but Google One offers a better GUI-based deployment. It's more sophisticated and integrates well with other services, providing a better customer experience.
What needs improvement?
There is room for improvement in this solution. For example, auto-scaling can be complex. We expect it to be easier to set up and manage, even for our customers.
For how long have I used the solution?
We started recently. I have around one and a half months of experience with it.
What do I think about the stability of the solution?
The stability is good. I would rate it a nine out of ten.
What do I think about the scalability of the solution?
It is a scalable solution. I would rate the scalability a nine out of ten.
How are customer service and support?
The customer service and support are good.
How would you rate customer service and support?
Positive
How was the initial setup?
The initial setup is pretty simple; we can deploy a cluster within a minute. Just choose a region and the required parameters.
What about the implementation team?
One person can handle it unless they're inexperienced.
What's my experience with pricing, setup cost, and licensing?
Pricing is a bit expensive compared to some other products, but it's acceptable.
I would rate the pricing an eight out of ten, where one is a low price, and ten is a high price.
What other advice do I have?
The auto-scaling can be complicated, so they should be prepared for that. It is difficult to hand over the solution to customers because of scaling up and scaling down issues. If Google improves that aspect, it'll be easier to manage.
Overall, I would rate the solution a nine out of ten.
Disclosure: My company has a business relationship with this vendor other than being a customer: Partner
CTO at Translucent Computing Inc
Extremely scalable, easy to setup, and has good machine learning
Pros and Cons
- "The deployment of the cluster is very easy."
- "Our critique is that we have to do too much work to get the cluster production-ready."
What is our primary use case?
We have everything in Kubernetes. We're basically moving everything from the cloud into Kubernetes - inverting the cloud. We have all that built for the CIT pipeline and have our tools within the cluster.
This is to support application development. The application side is always within the cluster. We have a security cluster. So everything is there. We have a database within the cluster as well. We don't need a managed database. There's a cloud database due to the fact that we use Kubernetes database. Everything goes into the cluster.
It makes it easy for us to be consistent across different environments, including development environments or in Oracle environments, as everything runs within the cluster.
What is most valuable?
The solution allows you to work on and from multiple clouds. You can use Google's cloud, or mix and match clouds across suppliers.
You can split into regions within your own cloud.
The deployment of the cluster is very easy. You just click a button and it's deployed, or just run a simple command and it deploys itself. You don't have to go through the steps of installing the cluster yourself. It's already deployed and managed.
The master of the cluster is also managed by Google. If there are any updates, they are responsible to handle that. It just takes a little bit of a load from our task load. You don't have to manage the master, or the version of the cluster yourself.
You don't have to think about the installation process. They take care of the underlying infrastructure deployment and managing the versioning of the cluster. When we need to update, it's simple. They'll help us to easily, smoothly update those cluster nodes. You don't have to deal with that either.
When it comes to the Google Cloud, the Kubernetes advantage that's there for machine learning is that they have a CPU, which is a central processing unit, which is much faster than GPU. If the clients are willing to pay for it, we'll run the machine learning jobs within the Kubernetes cluster, then connect to Google CPU, which gives us the ability to finish the job much, much faster.
What needs improvement?
It's maybe a controversial topic, as Kubernetes itself should be just your bottom layer. However, within your own engine, you expect to do more with time. Since we're putting so much into the cluster, it would be nice if some of this stuff was already done, baked into the cluster.
Our critique is that we have to do too much work to get the cluster production-ready. Most people just start it and think that's production. That's not really production. That's just bootstrapping the cluster, with all the tools that you need.
A lot of people rely on cloud tools, or a cloud-built system, to get going. We would like to have that baked into the cluster. Due to our usage pattern of the cluster and how heavily we use it, our expectation is to have more tools baked into the cluster. There should be more emphasis on tools developed immediately from the cluster to support application development versus relying on third-party vendors, like Jenkins.
The third-party vendors have to adapt to Kubernetes, and that creates a problem, as there's always a delay. Third parties don't have much incentive to do anything right away. That means we have to wait for these guys to catch up. We don't have a big enough team to actually change every open source code, as there's so much of it.
For how long have I used the solution?
We started using Kubernetes in 2015, around the time it started. Whenever Google launched their tools is about the time we started. Before that, we used Kubernetes, however, we were deploying it ourselves.
What do I think about the stability of the solution?
The solution is stable.
The solution is cloud-native and every cloud is using basically the same version. That's what makes it easy for us to move between clouds. Google wants users to integrate with their own cloud storage and security, however, which is where issues can arise.
It allows you to create private clusters. There's no competitive advantage for cluster clouds right now, which is good for us, as it's a uniform-looking ecosystem that allows us to move between clouds easily.
What do I think about the scalability of the solution?
Kubernetes is designed to scale horizontally and vertically as well. It scales quite well.
We have unlimited scaling through horizontal scaling. We can add more Kubernetes nodes. When applications do grow, we need to maybe horizontally scale applications or our databases. We just kick off another node when we need however much memory CPU and just keep on scaling it. Obviously, you pay for it, however, scaling is extremely easy.
A lot of time we automate the scaling as well. Based a little bit on AI and cloud automation processing, we detect the CPU usage or the GPU usage and if we exceed a certain threshold, the cluster automatically adds another data node, so it's self-serving. So scaling is almost automated around cases that we do.
The system knows by itself how to scale dynamically. The dynamic elastic scaling is baked into our systems as well. When people do use, for example, the machine learning special cluster, that one goes automatically from zero to 23 nodes depending on the users. A lot of times, we do shut it down, with just no usage. When people kick off a job, it automatically spins up on a new cluster node and deploys the job, gets another job, and spins up another one. It dynamically grows. We have to allocate pools that we have of an increased cluster in Google Cloud and it works well.
How are customer service and technical support?
We basically are able to solve our own problems on our end. We don't need the assistance of technical support. We might have used it once in the past six or seven years and that is it.
How was the initial setup?
The initial setup is very straightforward. Everything is basically done for you. It's nice and easy.
We can do the cluster management. There are cluster administrators who take a more serious role. They are responsible for the disks backing some of these applications, the databases, deployment of these tools, the infrastructure tools, et cetera.
What about the implementation team?
We have application developers which work with the administrators to actually deploy the application, as you still have to just be meaningful in the cluster, and add some sort of business logic. Those have to work with our administrators so we get that done and we do support, and a lot of different tools, monitoring tools for visibility. It's important to us, because if we do, we follow a microservice architecture across applications. They're like little black boxes and we have to be able to see inside of them.
What's my experience with pricing, setup cost, and licensing?
Multi-cloud is a sort of an expensive endeavor as the tools are overpriced. We're looking at options that aren't based on Anthos, which is Google's multi-cloud solution.
While you pay money to Google, they also take a piece of the action as well.
CPU is very cheap, however, GPU is very expensive. If you want to iterate on your data client's tasks within a Kubernetes cluster, it will cost you.
There is no licensing cost. You pay for the cloud and you pay for what you use based on the CPU and RAM usage based on the VM, the virtual machines. The cluster is still made up of computers, so you pay for the computers that are backing the clusters. If you kick off a Kubernetes node, which has three nodes in your cluster, you have to pay for each of these nodes, these computers, these virtual machines that you get bootstrapped with. You just use the machine time as with any cloud and you get a price in Google for the machine type and your machine type is defined based on your CPU and RAM usage. If you want to have 60 GBs of RAM, you pay for that RAM or for CPUs.
The same thing is true if you ask for a GPU computer, as most of the virtual machines don't come with a video card unless you ask for it. Then you have to pay for that the computer and the video card
What other advice do I have?
We're actually certified as well Kubernetes vendor.
We're using version 1.19. The most up-to-date is 1.20. We're never on the latest version, we're always like a version behind, or even two versions behind, to give them time to sort through their issues. We're using 1.19 in both Azure's, Google Cloud's, and EKS, however, EKS might be two versions behind, maybe.
Most of the time we're deploying in, as a private cluster within the cloud. It's isolated from public infrastructure. That's for security reasons. We don't want our cluster to be exposed to the public internet.
We also have a hybrid deployment Azure on-premises. This is just to make things easier for integration purposes. On-premise, it's connected to the cloud and then we can just use the same tools to be Kube-Native source. We develop the same tools for Kubernetes and then we can just deploy Kubernetes on-premises or in the cloud, it doesn't matter.
We also are doing multi-cloud as well, and we're deploying from Google Cloud into AWS.
With Azure, we have one giant cloud right now. That way, we can partition a cluster and see multiple clouds and multiple visions. If Google Cloud goes down for whatever reason, as it happened, two years ago, due to bad configurations, too many clusters in a cloud, we're covered. We do multi-cloud as the solution is critical and we can't afford to have it go down.
We are basically are a full-service company. We do everything for our clients - including application development and everything that entails.
I'd advise users to take security seriously. Don't just deploy things on the internet. Make sure your cluster's secure. You want to be able to tell your clients that you have a secure implementation of a cluster. That requires a little bit of cloud set up with every cloud to create a private network, private subnetwork, manage the ingress and egress, so input and outputs of the requests coming into your cluster.
These are things you have to think about when you deploy, just initially before you get started. All the clouds support it, you just have to know how to set up your VPC, virtual private network connection tier with every cloud and how to set up subnets to isolate your cluster to specific subnets, so it's not exposed on the internet, it's private and then any requests coming from the internet have to go to your load balancer directly to your cluster.
However, if you manage that and some peoples' requests going out of your cluster, you won't be able to manage those as well, since they're on NAT and a cloud router as well. So you know what's coming in, what's going out. You can monitor your traffic coming in and within your cluster.
These days a lot of people just use the containers directly from third-party sources or public repositories in the docker containers in which the Kubernetes cluster runs and those could come with malware. You want basically, in the main cluster, to have security policies implemented for every cluster. You don't get that from your cluster loggers. You have to get that from third-party vendors. This is where the competition comes with the Kubernetes.
In general, I would rate the solution at an eight out of ten.
Which deployment model are you using for this solution?
Public Cloud
If public cloud, private cloud, or hybrid cloud, which cloud provider do you use?
Google
Disclosure: I am a real user, and this review is based on my own experience and opinions.
Head of Infra and Applications support department at a financial services firm with 201-500 employees
An improvement over our traditional methods but additional security is required
Pros and Cons
- "Before using this solution, it was a lot of manual tasks and a lot of people participated in the process."
- "I think that security is an important point, and there should be additional features for the evaluation of data in containers that will create a more secure environment for usage in multi-parent models."
What is our primary use case?
Our primary use case is to arrange the correct CICD (Continuous Integration / Continous Deployment) conveyor to provide for continuous changes in production.
How has it helped my organization?
The improvement is mainly connected to the speed of change implementation. In the case of the automatic convenor, we spent less time due to the automation of the process. Before using this solution, it was a lot of manual tasks and a lot of people participated in the process.
What is most valuable?
The most valuable feature is the horizontal staging of applications. Other important features include isolation of applications and more effective usage of infrastructure due to less consumption of resources by containers.
What needs improvement?
I think that security is an important point, and there should be additional features for the evaluation of data in containers that will create a more secure environment for usage in multi-parent models.
For how long have I used the solution?
One year (pre-production).
What do I think about the stability of the solution?
We have not seen any signs of instability, and have an optimistic view of the solution.
What do I think about the scalability of the solution?
Scalability is an important feature of this solution, and we are happy with it.
We have approximately one hundred people using the solution. It is mostly developers and quality assurance people who are working on the preparation for CICD.
Once we move to production, next year, our usage will increase.
How are customer service and technical support?
I think technical support is good enough.
Which solution did I use previously and why did I switch?
We did not use an integrated solution prior to this. Rather, it was done in a more traditional way. This included virtual machine creation, installation of additional software, and connection to an external CICD conveyor, etc. We are switching because we are interested in more widely using continuous technology.
Our motivation for switching is to simplify creating a CICD process. We have a lot of small changes and after testing, we will be using an automated process for product delivery.
How was the initial setup?
We are currently in a test phase and are estimating the feasibility of moving this to production. Our plan is to finalize testing by the end of this year and move the solution to production at the beginning of next year.
We have two people working the maintenance of this solution, but frankly speaking, it is not enough. We are planning to improve our skills and capacity and expand these resources.
What about the implementation team?
We communicate directly with somebody who is part of OpenShift. They are top guys and have enough experience to help us build our system. It's no problem.
We do not have a local team in Russia, but at some point, that may change and we will use a local integrator.
What was our ROI?
We are planning to reach a positive ROI using this solution.
What's my experience with pricing, setup cost, and licensing?
We are planning to use external support, and hire a commercial partner for it. Usually, this is about twenty percent of the solution.
Which other solutions did I evaluate?
We have been watching what is happening the market, and for some time it has been obvious that Kubernetes has the most followers and most potential. This is why we are starting with Kubernetes from the beginning.
What other advice do I have?
My advice is not to implement this solution unless there is a genuine demand for it from the business side. It can be useful to start from the bottom of the infrastructure and take it to the highest level because it requires changes in the development and business levels to work with this technology.
I think that there is enough documentation available to start to work with this product. The technology provides a very good opportunity to grow and improve.
I would rate this solution a six out of ten.
Disclosure: I am a real user, and this review is based on my own experience and opinions.
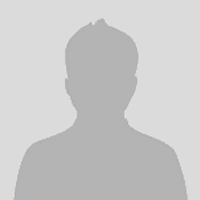
Buyer's Guide
Download our free Google Kubernetes Engine Report and get advice and tips from experienced pros
sharing their opinions.
Updated: December 2024
Product Categories
Container ManagementPopular Comparisons
VMware Tanzu Platform
Red Hat OpenShift Container Platform
Rancher Labs
Kubernetes
Nutanix Kubernetes Engine NKE
Amazon Elastic Container Service
HPE Ezmeral Container Platform
Buyer's Guide
Download our free Google Kubernetes Engine Report and get advice and tips from experienced pros
sharing their opinions.