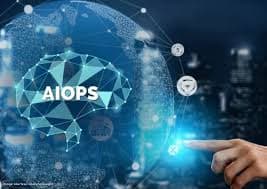
What Is AIOps?
AIOps is the practice of applying analytics and machine learning to big data to automate and improve IT operations. These new learning systems can analyze massive amounts of network and machine data to find patterns not always identified by human operators. These patterns can both identify the cause of existing problems and predict future impacts. The ultimate goal of AIOps is to automate routine practices in order to increase accuracy and speed of issue recognition, enabling IT staff to more effectively meet increasing demands.
History and Beginnings
The term AIOps was coined by Gartner in 2016. In the Market Guide for AIOps Platforms, Gartner describes AIOps platforms as “software systems that combine big data and artificial intelligence (AI) or machine learning functionality to enhance and partially replace a broad range of IT operations processes and tasks, including availability and performance monitoring, event correlation and analysis, IT service management and automation.”
AIOps Today
Ops teams are being asked to do more than ever before. In a common practice that can sometimes even feel laughable, old tools and systems never seem to die. Yet the same ops teams are under constant pressure to support more new projects and
technologies, very often with flat or declining staffing. To top it off, increased change frequencies and higher throughput in systems often mean the data these monitoring tools produce is almost impossible to digest.
To combat these challenges, AIOps:
•Brings together data from multiple sources: Conventional IT operations methods, tools and solutions aggregate and average data in simplistic ways that compromise data fidelity (as an example, consider the aggregation technique known as “averages of averages”). They weren’t designed for the volume, variety and velocity of data generated by today’s complex and connected IT environments. A fundamental tenet of an AIOps platform is its ability to capture large data sets of any type while maintaining full data fidelity for comprehensive analysis. An analyst should always be able to drill down to the source data that feeds any aggregated conclusions.
•Simplifies data analysis: One of the big differentiators for AIOps platforms is their ability to correlate these massive, diverse data sets. The best analysis is only possible with all of the best data. The platform then applies automated analysis on that data to identify the cause(s) of existing issues and predict future issues by examining intersections between seemingly disparate streams from many sources.
•Automates response: Identifying and predicting issues is important, but AIOps platforms have the most impact when they also notify the correct personnel, automatically remediate the issue once identified or, ideally, execute commands to prevent the issue altogether. Common remedies such as restarting a component or cleaning up a full disk can be handled automatically so that the staff is only involved once typical solutions have been exhausted.
Key Business Benefits of AIOps
By automating IT operations functions to enhance and improve system performance, AIOps can provide significant business benefits to an organization. For example:
•Avoiding downtime improves both customer and employee satisfaction and confidence.
•Bringing together data sources that had previously been siloed allows more complete analysis and insight.
•Accelerating root-cause analysis and remediation saves time, money and resources.
•Increasing the speed and consistency of incident response improves service delivery.
•Finding and fixing complicated issues more quickly improves IT’s capacity to support growth.
•Proactively identifying and preventing errors empowers IT teams to focus on higher-value analysis and optimization.
•Proactive response improves forecasting for system and application growth to meet future demand.
•Adding “slack” to an overwhelmed system by handling mundane work, allowing humans to focus on higher-order problems, yielding higher productivity and better morale.
Data Is Vital for AIOps
Data is the foundation for any successful automated solution. You need both historical and real-time data to understand the past and predict what’s most likely to happen in the future. To achieve a broad picture of events, organizations must access a range of historical and streaming data types of both human- and machine-generated data.
Better data from more sources will yield analytics algorithms better able to find correlations too difficult for humans to isolate, allowing the resulting automation tasks to be better curated. For example, it’s not hard in most semi-modern monitoring systems to automate some sort of response. However, if response times slow down an application, AIOps would help ensure the correct automated response and not just the “knee-jerk” response that’s statically connected. Adding more capacity to a service may in fact make a slowdown worse if the bottleneck isn’t related to capacity. And it certainly can result in unintended and unnecessary costs in cloud environments. Thus, having the right data to make more complete decisions results in better outcomes.
For total visibility, it’s necessary to access data in one place across all of your IT silos. It’s important to understand the underlying data supporting your services and applications — defining KPIs that determine health and performance status. As you move beyond data aggregation, search and visualizations to monitor and troubleshoot your IT, machine learning become the key to achieving predictive analysis and automation.
Key AIOps Use Cases
According to Gartner, there are five primary use cases for AIOps:
1. Performance analysis
2. Anomaly detection
3. Event correlation and analysis
4. IT service management
5. Automation
1. Performance analysis:
It has become increasingly difficult for IT professionals to analyze their data using traditional IT methods, even as those methods have incorporated machine learning technology. The volume and variety of data are just too large. AIOps helps address the problem of increasing volume and complexity of data by applying more sophisticated techniques to analyze bigger data sets to identify accurate service levels, often preventing performance problems before they happen.
2. Anomaly detection:
Machine learning is especially efficient at identifying data outliers — that is, events and activities in a data set that stand out enough from historical data to suggest a potential problem. These outliers are called anomalous events. Anomaly detection can identify problems even when they haven’t been seen before, and without explicit alert configuration for every condition.
Anomaly detection relies on algorithms. A trending algorithm monitors a single key performance indicator (KPI) by comparing its current behavior to its past. If the score grows anomalously large, the algorithm raises an alert. A cohesive algorithm looks at a group of KPIs expected to behave similarly and raises alerts if the behavior of one or more changes. This approach provides more insight than simply monitoring raw metrics and can act as a bellwether for the health of components and services.
AIOps makes anomaly detection faster and more effective. Once a behavior has been identified, AIOps can monitor and detect significant deviations between the actual value of the KPI of interest versus what the machine learning model predicts. Accurate anomaly detection is vital in complex systems as failures often exist in ways that are not always immediately clear to the IT professionals supporting them.
3. Event correlation and analysis:
The ability to see through an “event storm” of multiple, related warnings to identify the
underlying cause of events. The reality of most complex systems is that something is always “red” or alerting. It’s inevitable. The problem with traditional IT tools, however, is that they don’t provide insights into the problem, just a storm of warnings. This creates a phenomenon known as “alert fatigue”; teams see a particular alert that turns out to be trivial so often that they ignore the alert even on the occasions when it’s important.
AIOps automatically groups notable events based on their similarity. Think of this as drawing a circle around events that belong together, regardless of their source or format. This grouping of similar events reduces the burden on IT teams and reduces unnecessary event traffic and noise. AIOps focuses on key event groups and performs rule-based actions such as consolidating duplicate events, suppressing alerts or closing notable events. This enables teams to compare information more effectively to identify the cause of the issue.
4. IT service management (ITSM): A general term for everything involved in designing, building, delivering, supporting and managing IT services within an organization. ITSM
encompasses the policies, processes and procedures of delivering IT services to end-users within an organization. AIOps provides benefits to ITSM by letting IT professionals manage their services as a whole rather than as individual components. They can then use those whole units to define the system thresholds and automated responses to align with their ITSM framework, helping IT departments run more efficiently.
AIOps for ITSM can help IT departments to manage the whole service from a business perspective rather than managing components individually. For example, if one server in a pool of three machines encounters problems during a normal-load
period, the risk to the overall service may be considered low, and the server can be taken offline without any user-facing impact. Conversely, if the same thing were to happen during a high-
load period, an automated decision could be taken to add new capacity before taking any poor-performing systems offline.
In addition, AIOps for ITSM can help:
• Manage infrastructure performance in a multi-cloud environment more consistently
• Make more accurate predictions for capacity planning
• Maximize storage resource availability by automatically adjusting capacity based on forecasting needs.
• Improve resource utilization based on historical data and predictions
• Manage connected devices across a complex network
5. Automation: Legacy tools often require manually cobbling information together from multiple sources before it’s possible to understand, troubleshoot and resolve incidents. AIOps provides a significant advantage — automatically collecting and correlating data from multiple sources into complete services, increasing the speed and accuracy of identifying necessary relationships. Once an organization has a good handle on correlating and analyzing data streams, the next step is to automate responses to abnormal conditions.
An AIOps approach automates these functions across an organization’s IT operations, taking simple actions that responders would otherwise be forced to take themselves. Take for example a server that tends to run out of disk space every few weeks during high-volume periods due to known-issue logging. In a typical situation, a responder would be tasked with logging in, checking for normal behavior, cleaning up the excessive logs, freeing up disk space and confirming nominal performance has resumed. These steps could be automated so that an incident is created and responders are notified only if normal responses have already been tried and have not remedied the situation. These actions can range from the simple, like restarting a server or taking a server out of load-balancer pools, to more sophisticated, like backing out a recent change or rebuilding a server (container or otherwise).
AIOps automation can also be applied to:
•Servers, OS and networks: Collect all logs, metrics, configurations and messages to search, correlate, alert and report across multiple servers.
•Containers: Collect, search and correlate container data with other infrastructure data for better service context, monitoring and reporting.
•Cloud monitoring: Monitor performance, usage and availability of cloud infrastructure.
•Virtualization monitoring: Gain visibility across the virtual stack, make faster event correlations, and search transactions spanning virtual and physical components.
•Storage monitoring: Understand storage systems in context with corresponding app performance, server response times and virtualization overhead.
•Application monitoring: Identify application service levels and suggest or automate response to maintain defined service level objectives.
AIOps and the Shift to Proactive IT
One of the primary benefits of AIOps is its ability to help IT departments predict and prevent incidents before they happen, rather than waiting to fix them after they do. AIOps, specifically the application of machine learning to all of the data monitored by an IT organization, is designed to help you make that shift today.
By reducing the manual tasks associated with detecting, troubleshooting and resolving incidents, your team not only saves time but adds critical “slack” to the system. This slack allows you to spend time on higher-value tasks focused on increasing the quality of customer service. Your customer experience is maintained and improved by consistently maintaining uptime.
AIOps can have a significant impact in improving key IT KPIs, including:
• Increasing mean time between failures (MTBF)
• Decreasing mean time to detect (MTTD)
• Decreasing mean time to investigate (MTTI)
• Decreasing mean time to resolution (MTTR)
IT organizations who have implemented a proactive monitoring approach with AIOps have seen significant improvement in a variety of IT metrics, including:
How to Get Started With AIOps
The best way to get started with AIOps is an incremental approach. As with most new technology initiatives, a plan is key. Here are some important considerations to get you started.
Choose Inspiring Examples
If you’re evaluating AIOps solutions, platforms and vendors for your organization, you’ve got a big task ahead of you. The most challenging aspect may not be the evaluation process itself, but gaining the support and executive buy-in you need to conduct the evaluation.
If you choose inspiring examples of other, similar organizations that have benefited from AIOps — and have metrics to prove it — you’ll have a much easier time getting the go-ahead. A good partner can help you do that.
Consider People and Process
It’s obvious that technology plays an important role in AIOps, but it’s just as important to make a plan to address people and process.
For example, if an AIOps solution identifies a problem that’s about to happen and pages a support team to intervene, a responder might ignore the warning because nothing has actually happened yet. This can undermine trust in the AIOps solution before it has a chance to be proven in operation.
It’s also important to give IT teams the time to work on building, maintaining and improving systems. This vital work can’t be assigned as a side project or entry-level job if you expect meaningful change. Put your best people on it. Make it a high priority so other work can’t infringe on it. AIOps practices are iterative and must be refined over time; this can only be done with a mature and consistent focus on improvement.
You’ll also need to re-examine and adjust previously manual processes that had multiple levels of manager approval, like restarting a server. This requires trust in both technology and team practices. Building trust takes time. Start with simple wins to build cultural acceptance of automation. For example, be prepared to build historical reports that show previous incidents were correctly handled by a consistent, simple activity (such as a restart or disk cleanup) and offer to automate those tasks on similar future issues. Choose a solution that allows for “automation compromise” by inserting approval gates for certain activities. Over time, those gates should be removed to improve speed as analytics proves its value in selecting correct automation tasks.
Finally, include in your plans a campaign to reassure staff that AIOps is not intended to replace people with robots. Show them how AIOps can free up key resources to work on higher-value activities — limiting the unplanned work your teams have to endure each day.
The Bottom Line: Now Is the Time for AIOps
If you’re an IT and networking professional, you’ve been told over and over that data is your company’s most important asset, and that big data will transform your world forever. Machine learning and artificial intelligence will be transformative and AIOps provides a concrete way to leverage its potential for IT. From improving responsiveness to streamlining complex operations to increasing productivity of your entire IT staff, AIOps is a practical, readily available way to help you grow and scale your IT operations to meet future challenges. Perhaps most important, AIOps can solidify IT’s role as a strategic enabler of business growth.
Great article, @Shibu Babuchandran! Thank you for sharing your knowledge with the community!
I always like this order a lot: "Consider People and Process" and only after, Technology.