We use Databricks for data science work in projects that create data pipelines, pre-processing, data wrangling, big data cluster management and ML, machine learning and deep learning tasks.
Data Engineering Manager at a pharma/biotech company with 10,001+ employees
A great and easy-to-use platform for data engineers and data scientists who rely on a large dataset to do advanced analytics reporting
Pros and Cons
- "The most valuable feature is the Spark cluster which is very fast for heavy loads, big data processing and Pi Spark."
- "It would be great if Databricks could integrate all the cloud platforms."
What is our primary use case?
How has it helped my organization?
Databricks collaborates very well with the Azure platform, Dataiku, and enterprise AI tool. Databricks is a new connection to pull the data or connect to the Spark cluster. It is helpful for us to instance it or distribute the load through the Spark cluster, and it is very user-friendly.
What is most valuable?
The most valuable feature is the Spark cluster which is very fast for heavy loads, big data processing and Pi Spark.
What needs improvement?
Databricks as a solution is integrated with Azure, but Google Cloud has some restrictions. I'm not sure about AWS Cloud, but it would be great if Databricks could integrate all the cloud platforms. Regarding additional features, we would like to see them mostly on the data engineering side, where we have a Spark cluster and some inbuilt ML. In addition, pre-processing steps will be useful.
Buyer's Guide
Databricks
November 2024
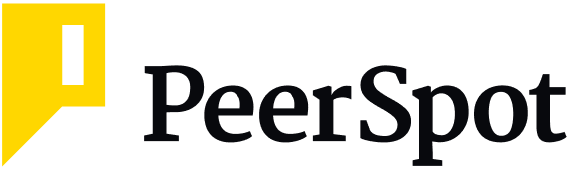
Learn what your peers think about Databricks. Get advice and tips from experienced pros sharing their opinions. Updated: November 2024.
816,406 professionals have used our research since 2012.
For how long have I used the solution?
We have been using this solution for two years and are using the latest update.
What do I think about the stability of the solution?
It is a stable solution as long as the Microsoft Azure Platform is stable too.
What do I think about the scalability of the solution?
It is a scalable solution, both vertically and horizontally, which is good. My organization is big, and we have a lot of users. In my department, we have about 15 people using Databricks.
How are customer service and support?
We have not escalated any issues to technical support, but we initially struggled with configuration and the settings of Hive metastore, but we resolved it. I rate the technical support a nine out of ten.
How would you rate customer service and support?
Positive
Which solution did I use previously and why did I switch?
We were using the looped EMR elastic MapReduce from AWS before using Databricks. We switched to Databricks because the whole platform changed from AWS to Azure platform, and Databricks comes as a package.
How was the initial setup?
The initial setup was easy to complete and not complex. It may initially be challenging for a new user, but it improves over time. The CICD pipeline works well with the Microsoft Azure platform because the continuous integration, development and deployment come with the Git integration. It makes it easier for Databricks and the CICD. The deployment should be improved from the perspective of auto ML functionality, so it doesn't have intensive automation learning capability.
We don't use Databricks directly because we work on a data science project. It requires an auto ML and inbuilt machine learning capability. We found capabilities like the large language model using NLP and other deep learning models that are not that intensive. It is meant for data engineering purposes rather than data science purposes. It'll be great if Databricks could be intensive for data science.
We used a third-party, Dataiku platform for the deployment, where we connected to Databricks and completed the ML ops. We required about three people for deployment, and it is easy to maintain the solution.
What was our ROI?
We have seen an ROI but cannot differentiate because it also comes with the Azure platform.
What's my experience with pricing, setup cost, and licensing?
I do not have details about the pricing.
What other advice do I have?
I rate this solution a nine out of ten. Regarding advice, Databricks is a very good platform, popular and easy to use daily for data engineers and data scientists who rely on a large dataset to do advanced analytics reporting. It's a very good tool.
Disclosure: I am a real user, and this review is based on my own experience and opinions.
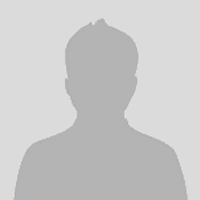
Machine Learning Engineer at a mining and metals company with 10,001+ employees
Highly scalable, stable and good technical support
Pros and Cons
- "Databricks is a scalable solution. It is the largest advantage of the solution."
- "The interface of Databricks could be easier to use when compared to other solutions. It is not easy for non-data scientists. The user interface is important before we had to write code manually and as solutions move to "No code AI" it is critical that the interface is very good."
What is our primary use case?
We were using Databricks to build an AI solution. We are only evaluating it, we have approximately three people that tried it out. Later we choose another solution, we did not fully deploy Databricks.
How has it helped my organization?
Before I used Databricks it took me a long time to do some functions and now with Databricks I can do them much quicker. It scales very well.
What needs improvement?
The interface of Databricks could be easier to use when compared to other solutions. It is not easy for non-data scientists. The user interface is important before we had to write code manually and as solutions move to "No code AI" it is critical that the interface is very good.
For how long have I used the solution?
I have used Databricks within the last 12 months.
What do I think about the stability of the solution?
The solution is stable.
What do I think about the scalability of the solution?
Databricks is a scalable solution. It is the largest advantage of the solution.
How are customer service and support?
We have been in contact with the technical support of Databricks, they were good.
Which solution did I use previously and why did I switch?
We have used a lot of different solutions, such as Watson and DataIQ.
How was the initial setup?
The initial setup is easy. However, I do not know much about the implementation because the company does it.
What about the implementation team?
We did the implementation of the solution.
What other advice do I have?
If companies want scalability, they should choose Databricks.
I rate Databricks a nine out of ten.
Which deployment model are you using for this solution?
Public Cloud
Disclosure: I am a real user, and this review is based on my own experience and opinions.
Buyer's Guide
Databricks
November 2024
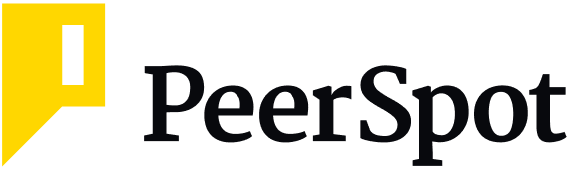
Learn what your peers think about Databricks. Get advice and tips from experienced pros sharing their opinions. Updated: November 2024.
816,406 professionals have used our research since 2012.
Executive Manager at Hexagon AB
Excellent data transformation but data-serving performance could be better
Pros and Cons
- "Databricks' most valuable feature is the data transformation through PySpark."
- "Databricks' performance when serving the data to an analytics tool isn't as good as Snowflake's."
What is our primary use case?
We mainly use Databricks to process ingest and do the ELT processes of data to get it ready for analytics and to serve the data to ThoughtSpot, which calls queries and Databricks to get the data.
How has it helped my organization?
We didn't have any good tooling for ELT processing prior to Databricks. We were using Microsoft HD Insight, but it was taking too long to process the data. When we changed our data-processing ELT processes over to Databricks, the amount of time to process the data was reduced to a fraction of what HD Insight used, so we were able to run jobs much faster.
What is most valuable?
Databricks' most valuable feature is the data transformation through PySpark.
What needs improvement?
Databricks' performance when serving the data to an analytics tool isn't as good as Snowflake's. In the next release, Databricks should include a better data-sharing platform to facilitate data sharing between companies.
For how long have I used the solution?
I've been using Databricks for three years.
What do I think about the stability of the solution?
Databricks' stability has been great, and I would rate it eight out of ten.
What do I think about the scalability of the solution?
Databricks is very scalable because it's very easy to spin up multiple clusters, but the cost of doing that is tremendous. I'd rate its scalability nine out of ten, but you'll pay for it.
How are customer service and support?
The technical support has been really bad, but that's because we don't have a direct agreement with Databricks.
How would you rate customer service and support?
Neutral
Which solution did I use previously and why did I switch?
I previously used HD Insight from Microsoft, but it took many, many hours to process data, so we switched to Databricks.
How was the initial setup?
The initial setup was pretty complex and required three people.
What about the implementation team?
We used an in-house team with some consulting help.
What was our ROI?
We've had a low ROI from Databricks.
What's my experience with pricing, setup cost, and licensing?
I would rate Databricks' pricing seven out of ten.
What other advice do I have?
I would advise anyone thinking of implementing Databricks to know their use case. For example, if you're looking for a big data repository to query data and do ELT processing, I recommend looking at other platforms, like Snowflake. However, if you're going to do AI and machine learning, then Databricks is probably stronger in that area. Overall, I would rate Databricks seven out of ten.
Which deployment model are you using for this solution?
Public Cloud
If public cloud, private cloud, or hybrid cloud, which cloud provider do you use?
Microsoft Azure
Disclosure: I am a real user, and this review is based on my own experience and opinions.
Senior Software Engineer at a computer software company with 201-500 employees
Valuable data analysis and engineering features with an easy setup
Pros and Cons
- "The setup is quite easy."
- "Can be improved by including drag-and-drop features."
What is our primary use case?
Our primary use case for the solution is data analysis by providing a Spark cluster environment with a driver to analyze a huge amount of data and gigabytes of data and can create Notebooks in Databricks. We can write SQL commands, Python code, Scala, or Spark with Python. With Databricks, we get a cluster hosted in the public cloud and we adjust it based on how much we use it.
What is most valuable?
The most valuable features are data engineering and data science because we can create Notebooks on them. We can use any Python library to build data science models, or we can use libraries like Seaborn or Matplotlib to create charts based on data for data analysis. It is a really valuable capability.
What needs improvement?
Microsoft Azure has its learning environment on the Microsoft website. We can complete certifications, but the Databricks certification is more expensive than Microsoft. It costs between $2,000 and $2,500, and the knowledge is linked. They're also charged based on whether a person doesn't want to analyze large amounts of data. Hence, we want to have the capacity for free student users so that people can learn and build their professional skills.
For how long have I used the solution?
We have been using the solution for approximately one year.
What do I think about the stability of the solution?
The solution is stable. Microsoft offers a public service, and we can get it from the Databricks website. Additionally, many companies use it to analyze their data or create a Spark cluster to run Python or SQL scripts based on their data. I rate the stability a nine out of ten.
How was the initial setup?
The setup is quite easy, and Databricks has also partnered with Microsoft, so we get this service on Microsoft Azure.
What was our ROI?
We have seen a return on investment.
What's my experience with pricing, setup cost, and licensing?
We have a pay-as-you-go subscription and pay for it based on our usage.
Which other solutions did I evaluate?
We chose this solution because my company uses Microsoft Azure for a project, and my role as a data engineer primarily focuses on data-related services. For storing data, we use Data Lake; similarly, for the data processing engine, we use Spark, which Databricks provides.
What other advice do I have?
I rate the solution an eight out of ten. The solution is good but can be improved by including drag-and-drop features because it can be helpful for users who are unfamiliar with coding. I advise new users to have prior experience with Python or SQL before utilizing this solution if they use it for data science or model building.
Disclosure: I am a real user, and this review is based on my own experience and opinions.
STI Data Leader at grupo gtd
Easy to use with a free community version and helpful documentation
Pros and Cons
- "The solution offers a free community version."
- "We'd like a more visual dashboard for analysis It needs better UI."
What is most valuable?
I like the simplicity and ease of use.
You can deploy the solution to many clouds easily.
The initial setup is straightforward.
The solution offers a free community version.
What needs improvement?
The auto models can be improved.
We can create auto models like Microsoft Azure Machine Learning. In Azure Machine Learning, they have these features, for example, for auto models or code, or by code. They need this in Databricks.
We need more connectors between on-premises and the cloud.
We'd like a more visual dashboard for analysis It needs better UI.
For how long have I used the solution?
I've used the solution for one and a half months.
What do I think about the stability of the solution?
The solution is very stable. There are no bugs or glitches. It doesn't crash or freeze.
What do I think about the scalability of the solution?
Scalability is no problem. At the beginning, we created a cluster, for example, and if we need more performance in the future, for example, or to accelerate the training, we can change the cluster. It's quite straightforward.
We have five people using the solution.
In one or two years, we'd like to promote the solution to clients and increase usage. Right now, the way it is used is limited. I know that some banks and aeronautics companies use it.
How are customer service and support?
In terms of technical support, for now, we use the community.
Which solution did I use previously and why did I switch?
We are also aware of KNIME, Azure Machine Learning, and Anaconda. In Anaconda, we use many frameworks, for example.
We started with other platforms, like Azure Machine Learning due to the fact that, with AutoML, it's easy to use. However, now that we have more skills, we need other tools or platforms like Databricks. It's a good platform to deploy and develop machine learning in employees.
How was the initial setup?
The implementation is quite easy. It's not complex or difficult. The first time, I did it using a tutorial which was quite helpful. Later, I took a course. I know it quite well.
The deployment only takes a few days.
You only need to deploy or maintain the solution.
What about the implementation team?
We did not need any outside assistance in terms of setting up the solution.
What's my experience with pricing, setup cost, and licensing?
For us, this product is free. We use the community version.
I am interested in using the enterprise version, however. Whether we use it or not depends on the projects and customers we get.
What other advice do I have?
I work with a solution provider. We are a Databrick customer.
We are not partners of Databricks. Only we are partnered with Microsoft Azure and Amazon AWS.
We are using the latest version of the solution. However, I do not know the exact version number.
I still need time with the solution before providing advice to others. I need to prepare the capacity internally. So far, it's been great.
I'd rate the solution eight out of ten.
Which deployment model are you using for this solution?
Public Cloud
Disclosure: I am a real user, and this review is based on my own experience and opinions.
Manager, Customer Journey at a retailer with 10,001+ employees
You can connect multiple data sources and share work easily
Pros and Cons
- "I like how easy it is to share your notebook with others. You can give people permission to read or edit. I think that's a great feature. You can also pull in code from GitHub pretty easily. I didn't use it that often, but I think that's a cool feature."
- "I would like it if Databricks adopted an interface more like R Studio. When I create a data frame or a table, R Studio provides a preview of the data. In R Studio, I can see that it created a table with so many columns or rows. Then I can click on it and open a preview of that data."
What is our primary use case?
I use Databricks for customer marketing analytics.
What is most valuable?
Databricks lets you schedule jobs pretty easily, and you can use SQL, Spark SQL, Python, or R. It also allows you to save a table or view.
I like that you can connect to multiple data sources. Most of our data is stored in the Azure data lake, but my previous company connected to SQL databases or even blob storage.
They've improved on many features. I don't do data engineering, but I had an issue a couple of years ago at my two companies ago. It took a long time to read and save tables, but I think the new Delta feature helped.
I like how easy it is to share your notebook with others. You can give people permission to read or edit. I think that's a great feature. You can also pull in code from GitHub pretty easily. I didn't use it that often, but I think that's a cool feature.
What needs improvement?
I would like it if Databricks adopted an interface more like R Studio. When I create a data frame or a table, R Studio provides a preview of the data. In R Studio, I can see that it created a table with so many columns or rows. Then I can click on it and open a preview of that data.
Because I work in analytics and not data engineering, I think that's probably the biggest one. There are better graphical tools, so I don't think Databricks can compete. You can do a simple graph, and it's not that great. However, I don't think they can ever stack up to Tableau, so it's probably not worth it to improve upon that.
For how long have I used the solution?
I've been using Databricks for two years.
What do I think about the stability of the solution?
Databricks is stable.
What do I think about the scalability of the solution?
Databricks is scalable.
How are customer service and support?
Databricks tech support has been great every time I've dealt with them. Their team is highly knowledgeable.
How was the initial setup?
Setting up Databricks is easy. I set it up at my previous company. That was on Azure as well, but they utilized a third-party team with expertise in Databricks to ensure everything was optimized.
What other advice do I have?
I rate Databricks 10 out of 10. I recommend taking advantage of Databricks support or a third-party provider to ensure it's set up optimally. I don't know if it's an additional service you must pay for, but we always had access to Databricks support in my last company.
I think that's worth the money because there are so many different scenarios with distributed computing. Even people who study analytics may not understand the ins and out of Spark. It's worth it to have a service contract for support.
Which deployment model are you using for this solution?
Public Cloud
If public cloud, private cloud, or hybrid cloud, which cloud provider do you use?
Microsoft Azure
Disclosure: I am a real user, and this review is based on my own experience and opinions.
Director - Data Engineering expert at Sankir Technologies
Is user friendly and has great performance, but documentation needs improvement
Pros and Cons
- "Databricks has a scalable Spark cluster creation process. The creators of Databricks are also the creators of Spark, and they are the industry leaders in terms of performance."
- "If I want to create a Databricks account, I need to have a prior cloud account such as an AWS account or an Azure account. Only then can I create a Databricks account on the cloud. However, if they can make it so that I can still try Databricks even if I don't have a cloud account on AWS and Azure, it would be great. That is, it would be nice if it were possible to create a pseudo account and be provided with a free trial. It is very essential to creating a workforce on Databricks. For example, students or corporate staff can then explore and learn Databricks."
What is our primary use case?
I use Databricks to explore new features and provide the industry visibility and scalability of Databricks to the companies that I work with.
I create proof of concepts for companies. As a consultant, I also create training courses on Databricks. If a company wants to leverage a service provided by Databricks and needs to train people, they use our courses.
What is most valuable?
Databricks has a scalable Spark cluster creation process. The creators of Databricks are also the creators of Spark, and they are the industry leaders in terms of performance.
Databricks has made great strides in terms of performance.
It is very user friendly. I like the ease of creating a Spark cluster, submitting a job, or creating a notebook.
The UI has also changed for the better compared to what it was two years ago.
What needs improvement?
If I want to create a Databricks account, I need to have a prior cloud account such as an AWS account or an Azure account. Only then can I create a Databricks account on the cloud. However, if they can make it so that I can still try Databricks even if I don't have a cloud account on AWS and Azure, it would be great. That is, it would be nice if it were possible to create a pseudo account and be provided with a free trial. It is very essential to creating a workforce on Databricks. For example, students or corporate staff can then explore and learn Databricks.
It's a big ask to have people jump through a lot of hoops to get approval to create a Databricks cluster just to explore it, but if they can try it on their own with a free trial without an underlying cloud account it would be more convenient.
Documentation can be improved as well. There are so many versions of documents. For example, when I tried to create a DBU vault and secrets file, I had to go through multiple versions of documents. This could be improved so that the documentation is easy to use.
For how long have I used the solution?
I've been using this solution for about two years.
What do I think about the stability of the solution?
Stability wise, it's quite okay. In my experience, it doesn't crash.
What do I think about the scalability of the solution?
I have not used autoscaling because it consumes a lot of money and because my experience has been alright. In some cases, though, it is tied to the quota of the underlying infrastructure. I have not tested the scalability to its fullest extent, but with the workloads I run, it has been fine.
How are customer service and support?
When I wanted to create an AWS account and contacted technical support via email, I never received a response. Recently, however, I think they have improved their support a little bit, and I did get a call in response to my question. Overall, I've not faced any issues with the person I had to contact directly.
How was the initial setup?
The initial setup is not very easy, but it's medium in complexity.
What's my experience with pricing, setup cost, and licensing?
Databricks is a very expensive solution. Pricing is an area that could definitely be improved. They could provide a lower end compute and probably reduce the price.
What other advice do I have?
I would rate Databricks at seven on a scale from one to ten. If you compare it to Snowflake, for example, Snowflake doesn't mandate an underlying cloud account. It creates one on its own. That's a subtle convenience that Snowflake has and one that Databricks could also build.
Snowflake's documentation is easy to use in comparison to that of Databricks.
Which deployment model are you using for this solution?
Public Cloud
Disclosure: I am a real user, and this review is based on my own experience and opinions.
Co-founder/Senior Data Scientist at Hence
Responsive support, integrates and scales well
Pros and Cons
- "The most valuable feature of Databricks is the integration of the data warehouse and data lake, and the development of the lake house. Additionally, it integrates well with Spark for processing data in production."
- "The solution could be improved by adding a feature that would make it more user-friendly for our team. The feature is simple, but it would be useful. Currently, our team is more familiar with the language R, but Databricks requires the use of Jupyter Notebooks which primarily supports Python. We have tried using RStudio, but it is not a fully integrated solution. To fully utilize Databricks, we have to use the Jupyter interface. One feature that would make it easier for our team to adopt the Jupyter interface would be the ability to select a specific variable or line of code and execute it within a cell. This feature is available in other Jupyter Notebooks outside of Databricks and in our own IDE, but it is not currently available within Databricks. If this feature were added, it would make the transition to using Databricks much smoother for our team."
What is our primary use case?
We are using Databricks for machine learning workloads specifically.
Databricks aligns well with our skillset and overall approach. We sought out their solution specifically for a big data application we are currently working on, as we needed a platform capable of handling large amounts of data and building models. Additionally, the fact that they use open-source software and can integrate data warehouse and data lake systems was particularly appealing, as we have encountered such issues in the past. We determined that Databricks would be an effective solution for our needs.
What is most valuable?
The most valuable feature of Databricks is the integration of the data warehouse and data lake, and the development of the lake house. Additionally, it integrates well with Spark for processing data in production.
What needs improvement?
The solution could be improved by adding a feature that would make it more user-friendly for our team. The feature is simple, but it would be useful. Currently, our team is more familiar with the language R, but Databricks requires the use of Jupyter Notebooks which primarily supports Python. We have tried using RStudio, but it is not a fully integrated solution. To fully utilize Databricks, we have to use the Jupyter interface. One feature that would make it easier for our team to adopt the Jupyter interface would be the ability to select a specific variable or line of code and execute it within a cell. This feature is available in other Jupyter Notebooks outside of Databricks and in our own IDE, but it is not currently available within Databricks. If this feature were added, it would make the transition to using Databricks much smoother for our team.
The most important feature other than the Jupyter interface would be to have the RStudio interface inside Databricks. This would be perfect.
For how long have I used the solution?
We have been using Databricks for approximately one year.
What do I think about the stability of the solution?
The stability of Databricks is good.
I rate the stability of Databricks a nine out of ten.
What do I think about the scalability of the solution?
Databricks is scalable.
I rate the scalability of Databricks a nine out of ten.
How are customer service and support?
I have been receiving responsive answers from Databricks's support. I have been pleased with the support.
I rate the support from Databricks a ten out of ten.
How would you rate customer service and support?
Positive
How was the initial setup?
The initial setup of Databricks is simple. I did not experience any challenges. The time it takes for the deployment is approximately four hours.
I rate the initial setup of Databricks.
What about the implementation team?
We did the deployment of the solution in-house. There were three people involved in the deployment. A data engineer, data analyst, and machine learning engineer.
What's my experience with pricing, setup cost, and licensing?
We have only incurred the cost of our AWS cloud services. This is because during this period, Databricks provided us with an extended evaluation period, and we have not spent much money yet. We are just starting to incur costs this month, I will know more later on the full cost perspective.
We only pay standard fees for the solution.
What other advice do I have?
We use a data engineer, data analyst, and machine learning engineer for the maintenance of the solution.
I rate Databricks a nine out of ten.
Which deployment model are you using for this solution?
Public Cloud
If public cloud, private cloud, or hybrid cloud, which cloud provider do you use?
Amazon Web Services (AWS)
Disclosure: I am a real user, and this review is based on my own experience and opinions.
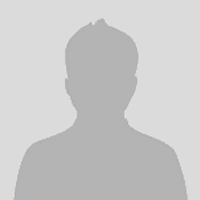
Buyer's Guide
Download our free Databricks Report and get advice and tips from experienced pros
sharing their opinions.
Updated: November 2024
Popular Comparisons
Microsoft Azure Machine Learning Studio
KNIME
Alteryx
Amazon SageMaker
Dataiku
RapidMiner
IBM SPSS Statistics
Dremio
IBM Watson Studio
IBM SPSS Modeler
Anaconda
Domino Data Science Platform
Starburst Enterprise
H2O.ai
Cloudera Data Science Workbench
Buyer's Guide
Download our free Databricks Report and get advice and tips from experienced pros
sharing their opinions.
Quick Links
Learn More: Questions:
- Which do you prefer - Databricks or Azure Machine Learning Studio?
- How would you compare Databricks vs Amazon SageMaker?
- Which would you choose - Databricks or Azure Stream Analytics?
- Which product would you choose for a data science team: Databricks vs Dataiku?
- Which are the best end-to-end data science platforms?
- What enterprise data analytics platform has the most powerful data visualization capabilities?
- What Data Science Platform is best suited to a large-scale enterprise?
- How can ML platforms be used to improve business processes?
- When evaluating Data Science Platforms, what aspect do you think is the most important to look for?