We use the solution for the model garden. We leverage both open-source models and Google Vertex AI's embedding models and use them to deploy our machine-learning models.
Data Scientist at Tradeindia.com-Infocom Network Ltd
Has model garden feature and easy to learn as a beginner
Pros and Cons
- "The most valuable feature we've found is the model garden, which allows us to deploy and use various models through the provided endpoints easily."
- "The tool's documentation is not good. It is hard."
What is our primary use case?
What is most valuable?
The most valuable feature we've found is the model garden, which allows us to deploy and use various models through the provided endpoints easily.
What needs improvement?
The tool's documentation is not good. It is hard.
For how long have I used the solution?
I have been working with the product for two years.
Buyer's Guide
Google Vertex AI
February 2025
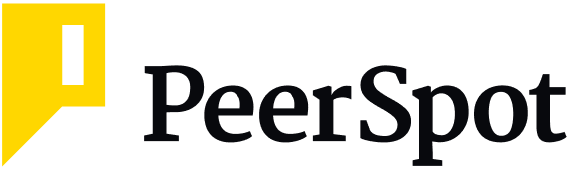
Learn what your peers think about Google Vertex AI. Get advice and tips from experienced pros sharing their opinions. Updated: February 2025.
837,501 professionals have used our research since 2012.
What do I think about the stability of the solution?
The tool is stable, and I haven't faced any bugs.
What do I think about the scalability of the solution?
Google Vertex AI is scalable. My company has four to five users.
How was the initial setup?
The tool's deployment was easy. The Cloud Run feature was tricky.
What's my experience with pricing, setup cost, and licensing?
The solution's pricing is moderate.
What other advice do I have?
I would recommend using Google Vertex AI. It's a good product, but it depends on your use case. If your needs align with its capabilities, it's worth considering. I started as a beginner and found it easy to learn and use. I rate it an eight out of ten.
Disclosure: I am a real user, and this review is based on my own experience and opinions.
Last updated: Jul 29, 2024
Flag as inappropriate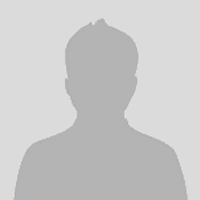
Data Engineer at a tech consulting company with 201-500 employees
Enables you to train and deploy ML models and AI applications, and customize LLMsfor use in your AI-powered applications
Pros and Cons
- "We extensively utilize Google Cloud's Vertex AI platform for our machine learning workflows. Specifically, we leverage the IO branch for EDA data in Suresh Live Virtual, employing Forte IT for training machine learning models. The AI model registry in Vertex AI is crucial for cataloging and managing various versions of the models we develop. When it comes to deploying models, we rely on Google Cloud's AI Prediction service, seamlessly integrating it into our workflow for real-time predictions or streaming. For monitoring and tracking the outcomes of model development, we employ Vertex AI Monitoring, ensuring a comprehensive understanding of the model's performance and results. This integrated approach within Vertex AI provides a unified platform for managing, deploying, and monitoring machine learning models efficiently."
- "I believe that Vertex AI is a robust platform, but its effectiveness depends significantly on the domain knowledge of the developer using it. While Vertex AI does offer support through the console UI in the Google Cloud environment, it is better suited for technical members who have a deeper understanding of machine learning concepts. The platform may be challenging for business process developers (BPDUs) who lack extensive technical knowledge, as it involves intricate customization and handling numerous parameters. Effectively utilizing Vertex AI requires not only familiarity with machine learning frameworks like TensorFlow or PyTorch but also a proficiency in Python programming. The complexity of these requirements might pose challenges for less technically oriented users, making it crucial to have a solid foundation in both machine learning principles and Python coding to extract the full value from Vertex AI. It would be beneficial to have a streamlined process where we can leverage the capabilities of Vertex AI directly through the BigQuery UI. This could involve functionalities such as creating machine learning models within the BigQuery UI, providing a more user-friendly and integrated experience. This would allow users to access and analyze data from BigQuery while simultaneously utilizing Vertex AI to build machine learning models, fostering a more cohesive and efficient workflow."
What is our primary use case?
We use Vertex AI for building machine learning workflows. This encompasses the entire process, from developing the workflow for training models to making predictions. Additionally, it handles the integration of diverse data types, including electronic data interchange (EDI), Salesforce (SFDC), and other formats. This aligns with the information you inquired about during our discussion last week.
What is most valuable?
We use Google Cloud's Vertex AI platform for our machine learning workflows. Specifically, we leverage the IO branch for EDA data. The AI model registry in Vertex AI is crucial for cataloging and managing various versions of the models we develop. When it comes to deploying models, we rely on Google Cloud's AI Prediction service, seamlessly integrating it into our workflow for real-time predictions or streaming.
For monitoring and tracking the outcomes of model development, we employ Vertex AI Monitoring, ensuring a comprehensive understanding of the model's performance and results. This integrated approach within Vertex AI provides a unified platform for managing, deploying, and monitoring machine learning models efficiently.
What needs improvement?
I believe that Vertex AI is a robust platform, but its effectiveness depends significantly on the domain knowledge of the developer using it. While Vertex AI does offer support through the console UI in the Google Cloud environment, it is better suited for technical members who have a deeper understanding of machine learning concepts.
The platform may be challenging for business process developers (BPDUs) who lack extensive technical knowledge, as it involves intricate customization and handling of numerous parameters. Effectively utilizing Vertex AI requires not only familiarity with machine learning frameworks like TensorFlow or PyTorch but also proficiency in Python programming. The complexity of these requirements might pose challenges for less technically oriented users, making it crucial to have a solid foundation in both machine learning principles and Python coding to extract the full value from Vertex AI.
It would be beneficial to have a streamlined process where we can leverage the capabilities of Vertex AI directly through the BigQuery UI. This could involve functionalities such as creating machine learning models within the BigQuery UI, providing a more user-friendly and integrated experience. This would allow users to access and analyze data from BigQuery while simultaneously utilizing Vertex AI to build machine learning models, fostering a more cohesive and efficient workflow.
For how long have I used the solution?
I have been using Google Vertex AI for two to three months.
What do I think about the stability of the solution?
I would rate the stability of Vertex AI at nine. It has proven to be highly reliable for both business and technical aspects. On the business side, Vertex AI empowers users to explore and implement machine learning models effectively, contributing to business growth and data analysis.
From a technical perspective, the infrastructure is robust, ensuring there is no downtime. The seamless integration with other tools like Cloud Composer and Dataflow allows for comprehensive control over the entire machine learning workflow, creating a cohesive and efficient experience.
What do I think about the scalability of the solution?
The scalability of Vertex AI is a perfect 10. Leveraging the robust infrastructure of Google has evidently provided us with ample resources to seamlessly run our machine learning processes. The absence of any limitations or constraints allows us to execute our tasks without hindrance, highlighting the efficiency and reliability of the tool.
How are customer service and support?
While the support is generally good, there have been instances where the response time was slower than expected. Since Vertex AI is a managed service, the support team sometimes takes longer to address queries as they need to escalate them to the product team. I believe there is room for improvement in terms of faster resolution of tickets and quicker responses, especially for issues that could be resolved more promptly.
How would you rate customer service and support?
Positive
How was the initial setup?
The initial setup with Vertex AI poses no challenges, especially given the seamless interaction with Google Cloud. However, I acknowledge that the platform involves a plethora of parameters and technical intricacies that must be addressed to configure the environment correctly. It seems like a more straightforward, user-friendly approach to configuring these parameters would be beneficial, ensuring that users can easily and accurately set up the environment without encountering complexities. Simplifying these technical aspects could further enhance the overall user experience with Vertex AI.Our workflow typically begins with the initial step of acquiring the Vertex AI API, a prerequisite for utilizing Vertex AI's capabilities. Subsequently, we proceed with the Vertex AI Workbench, focusing on exploratory data analysis (EDA) to extract relevant features crucial for our machine learning models. Following this, we engage in Extract, Transform, Load (ETL) processes, manipulating and storing data in platforms like BigQuery or Cloud Storage.
The subsequent phase involves coding the machine learning algorithm and encapsulating it into an image bundle. This bundle is then pushed into the Vertex AI training environment, initiating the training job. Once the model is successfully trained, we leverage the Vertex AI Model Registry for efficient model deployment into production. This deployment can take various forms, such as batch predictions or real-time streaming endpoints.
The final stage of our workflow often includes addressing customer requests for model monitoring, a task facilitated by the Vertex AI Monitoring tool. While some clients opt to include this monitoring step, others conclude their workflow at the AI model deployment stage. Overall, our workflow is comprehensive, covering all essential aspects from initial data analysis to deploying fully trained models into production.
What's my experience with pricing, setup cost, and licensing?
Vertex AI offers attractive pricing. With this pricing structure, I can leverage various opportunities to bring value to my business. It's a positive aspect worth considering.
What other advice do I have?
I would rate the overall solution 7 out of 10.
Disclosure: My company has a business relationship with this vendor other than being a customer: partner
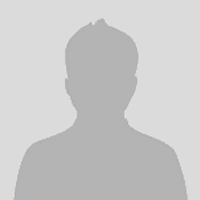
Buyer's Guide
Download our free Google Vertex AI Report and get advice and tips from experienced pros
sharing their opinions.
Updated: February 2025
Popular Comparisons
Microsoft Azure Machine Learning Studio
Amazon SageMaker
Azure OpenAI
Hugging Face
TensorFlow
Google Cloud AI Platform
IBM Watson Studio
Replicate
OpenVINO
DataRobot
Together Inference
IBM Watson Machine Learning
Fireworks AI
PyTorch
GroqCloud Platform
Buyer's Guide
Download our free Google Vertex AI Report and get advice and tips from experienced pros
sharing their opinions.
Quick Links
Learn More: Questions:
- When evaluating Artificial Intelligence Development Platforms, what aspect do you think is the most important to look for?
- What are the main storage requirements to support Artificial Intelligence and Deep Learning applications?
- What is the most effective AI platform to work with? Does it help if it is also "fun"?
- What are the major Edge AI technology use cases that can be used in the Banking/Finance, Power and Agricultural sectors?
- What are the top emerging trends in AI and ML in 2022?
- How do I do AI implementation?
- Why is AI Development Platforms important for companies?