We primarily use Microsoft Azure Cosmos DB as a transactional data store and for some event-driven applications. We utilize the change feed, and the function app triggers quite a bit. MPerks, our customer loyalty application, uses it. It has become our go-to database, and we hardly touch SQL Server for new stuff.
Cloud Engineer at a energy/utilities company with 10,001+ employees
Has incredible latency and availability
Pros and Cons
- "The features most valuable to us in Microsoft Azure Cosmos DB are the auto scale and change feed. These features allow us to do some operations that are not possible with SQL Server."
- "Latency and availability are incredible."
- "One of our biggest pain points is the backup and restore functionality needs improvement. They've gotten a little better in this area. SQL Server's long-term retention is amazing, and you can restore data from years ago. You need to open a support Microsoft ticket to restore your Cosmos DB backup, and it comes in on a different Cosmos account. It's just kind of a headache to restore data."
- "One of our biggest pain points is the backup and restore functionality needs improvement."
What is our primary use case?
How has it helped my organization?
Our developers find Microsoft Azure Cosmos DB easy to use and more scalable. The whole cloud model of only paying for what you use fits our organization well.
What is most valuable?
The features most valuable to us in Microsoft Azure Cosmos DB are the auto scale and change feed. These features allow us to do some operations that are not possible with SQL Server. It is super configurable, allowing us to pick and choose the different Cosmos databases we need, whether or not dynamic scaling is the right thing for that workload.
Latency and availability are incredible. Given that our data is partitioned and indexed correctly, we can run queries and get results in less than five milliseconds. This has resulted in happier customers.
Cosmos is super-easy to use. It adopts a whole document database strategy with no relational data, so what you see is what you get. It's straightforward to understand, and you no longer need to worry about entity diagrams.
What needs improvement?
One of our biggest pain points is the backup and restore functionality needs improvement. They've gotten a little better in this area. SQL Server's long-term retention is amazing, and you can restore data from years ago. You need to open a support Microsoft ticket to restore your Cosmos DB backup, and it comes in on a different Cosmos account. It's just kind of a headache to restore data.
CosmosDB's ability to search through large amounts of data isn't great. It kills the RUs if you're using the transactional store. We use Synapse Analytics for our more analytical workloads. We love Synapse for that purpose.
Buyer's Guide
Microsoft Azure Cosmos DB
February 2025
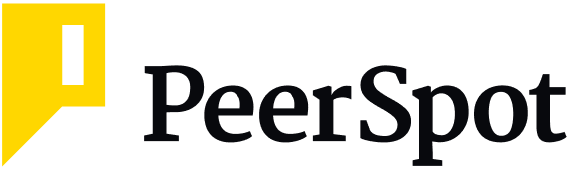
Learn what your peers think about Microsoft Azure Cosmos DB. Get advice and tips from experienced pros sharing their opinions. Updated: February 2025.
839,164 professionals have used our research since 2012.
For how long have I used the solution?
I have been using Microsoft Azure Cosmos DB for about six years.
What do I think about the scalability of the solution?
There are no critical scalability issues with Microsoft Azure Cosmos DB. It scales well with RUs, and it is never an issue for us. Our issues usually lie more on the application side.
How are customer service and support?
The support experience has been pretty good, and I don't have a lot of complaints.
How would you rate customer service and support?
Positive
Which solution did I use previously and why did I switch?
Previously, we used SQL Server. Microsoft Azure Cosmos DB was chosen because it is the go-to document data store, and our developers are familiar with SQL syntax.
How was the initial setup?
New developers are able to get jumpstarted on Microsoft Azure Cosmos DB quickly. Although we learned some lessons on how to structure and partition data, the initial setup was not problematic.
What was our ROI?
I can't specify the exact ROI, but Microsoft Azure Cosmos DB has decreased our total cost of ownership.
What's my experience with pricing, setup cost, and licensing?
We pay for what we use, with the flexibility to reserve our use. Autoscaling is a premium option, but it helps when our database isn't in high demand. It provides flexibility in configuring our RUs, whether we want to do it at the database or container level. We have lots of options to configure and pay for the solution.
Which other solutions did I evaluate?
We evaluated AWS solutions, but ultimately chose Microsoft Azure Cosmos DB.
What other advice do I have?
I would rate Microsoft Azure Cosmos DB a nine out of 10. Both Microsoft Azure Cosmos DB and Cosmos SQL DB are familiar to our developers who come from a SQL Server background.
Which deployment model are you using for this solution?
Public Cloud
Disclosure: I am a real user, and this review is based on my own experience and opinions.
Last updated: Nov 24, 2024
Flag as inappropriate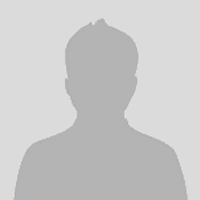
Manager, Development Practice at All Lines Technology
Having data in a flat file format speeds up processes
Pros and Cons
- "Azure Cosmos DB's graph queries are its most valuable feature. Although I have not yet explored vector search, it's coming to Cosmos DB, and I plan to look into it. Having data in a flat file format in a document database speeds up processes, which is the primary purpose. Additionally, Cosmos DB's use of the Mongo platform makes it intuitive and cost-effective."
- "Cosmos DB has helped us by providing faster response times for everything, which significantly improved our search results quality."
- "There are no specific areas I believe need improvement as I am happy with what I am getting currently. However, I am open to new features in future versions, like possibly integrating AI features natively into Cosmos DB. Any improvement would be beneficial."
What is our primary use case?
Our primary use case is mirroring the data for reporting.
How has it helped my organization?
Cosmos DB has helped us by providing faster response times for everything, which significantly improved our search results quality.
What is most valuable?
Azure Cosmos DB's graph queries are its most valuable feature. Although I have not yet explored vector search, it's coming to Cosmos DB, and I plan to look into it. Having data in a flat-file format in a document database speeds up processes, which is the primary purpose. Additionally, Cosmos DB's use of the Mongo platform makes it intuitive and cost-effective.
I use Azure AI services, including cognitive services and OCR. I recently built a chatbot using the model. Cosmos DB integrates well with other apps.
What needs improvement?
There are no specific areas I believe need improvement as I am happy with what I am getting currently. However, I am open to new features in future versions, like possibly integrating AI features natively into Cosmos DB. Any improvement would be beneficial.
For how long have I used the solution?
I have been using Azure Cosmos DB for more than five years.
What do I think about the stability of the solution?
I have not encountered any issues related to the stability of Cosmos DB. The challenge does not lie in the technical aspect of Cosmos DB but in the non-technical aspects.
What do I think about the scalability of the solution?
Cosmos DB has impressive scalability. We have been able to scale workloads as needed during peak hours without any issues, effectively meeting our expectations.
How was the initial setup?
The initial setup was quite quick, taking only a few days for the team to be onboarded with Cosmos DB. The primary challenge was non-technical.
What about the implementation team?
The implementation involved just the normal onboarding process for any human resource.
What was our ROI?
Our organization's total cost of ownership has been reduced by 20 percent due to the backend data mirroring for setting up the repository for reporting purposes. Dynamic scaling has also helped decrease our organization's overhead cost by automating the scaling process and reducing the need for human intervention.
What's my experience with pricing, setup cost, and licensing?
Our experience with the pricing and setup cost is that it aligns with what we expect based on the pricing we see. However, I would absolutely like it to be less if possible.
What other advice do I have?
I rate Azure Cosmos DB eight out of 10. There is always room for improvement, and the company could develop new features that could make it even better, but I am very satisfied with the current performance.
Disclosure: My company has a business relationship with this vendor other than being a customer: Partners
Last updated: Dec 16, 2024
Flag as inappropriateBuyer's Guide
Microsoft Azure Cosmos DB
February 2025
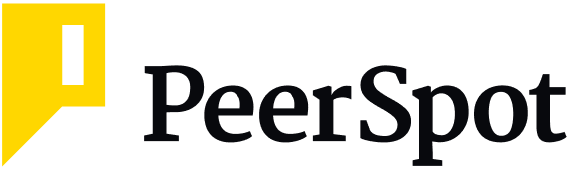
Learn what your peers think about Microsoft Azure Cosmos DB. Get advice and tips from experienced pros sharing their opinions. Updated: February 2025.
839,164 professionals have used our research since 2012.
CEO at a tech vendor with 201-500 employees
Amazing cost reduction and the best in terms of performance and scale
Pros and Cons
- "Change feed is a pretty amazing feature. Once you make the changes, they are quickly read for you, and then you also have geo-replication. You can do a lot of things in your region, and the same regions can be replicated all over the world."
- "In the long run, there should be an addition of more features, especially because this space is evolving quickly. It all boils down to how many more features you are adding, how many integrations you are supporting, and how many more APIs you have that are standard APIs."
What is our primary use case?
We use it for different companies and different clients. We have Fortune 500, startups, and mid-sized companies as our clients. They are in healthcare, finance, fintech, tech, manufacturing, construction, real estate, telecom, and a lot of other industries. They all love it.
How has it helped my organization?
It is the best in terms of performance and scale, and it can do both SQL and NoSQL workloads, so it is pretty impressive. One of the least understood use cases happens to be cost and caching. It has a pretty amazing caching engine, and its cost is amazingly low. Especially with the strategies we have designed, we can show a cost reduction of 99% in certain cases. The request charge reduction is anywhere between 75% to 99%. It has been pretty amazing to get cost, quality, and time. We can get all three with it. It is one of the very few databases that can even get there.
We use the built-in vector database capability. It is pretty fascinating. You get recalls that are pretty high. In the competitive landscape of databases, it is surprisingly better in terms of p95 latency and also requests per second, which is something that every customer wants but does not easily get by default. You can also use HNSW, for example, a lot cheaper than you would otherwise because of the DiskANN technology. It is similar to HNSW, but it is on the disk, so it is cheaper. You are not going to the memory. That saves you a lot of money, which is important because when you are running workloads that are getting to terabytes and terabytes, the cost is a huge concern, especially to support the underlying business. That is pretty amazing in terms of DiskANN, which is a Microsoft technology that is very well implemented in Azure Cosmos DB.
Usually, the vector database is integrated with a bunch of other applications. It could be a CRM system behind the scenes, or it could be any LLM-based application. The interoperability with other solutions is fairly simple because, at the end of the day, it is just an API. You can make it work with anything.
We use a lot of different models including Azure OpenAI and some open-source ones through Azure AI Studio. They are very easy to use with it because it is just an API.
It is as fast as what you would find elsewhere. It scales, and they do that part for you. Performance and scale are the things that Azure Cosmos DB got right. That is definitely a positive. If you do not use the vector database, you may get into hallucination issues. Things might slow down. Such issues do not happen if you are using the vector database correctly. Your LLM is now supported with the rack pattern, which is done very well by DiskANN and Azure Cosmos DB.
DiskANN does a great job with recalls. We can decide how high we want them to be. That is the best it gets. If you are using vector searches, it does a great job. I do not usually use Azure Cosmos to compete with a regular or classic search engine.
In terms of Azure Cosmos DB’s ability to search through large amounts of data, currently, the maximum we have on it is in terabytes, but a lot of that depends on how you do a lot of things. That includes data modeling and partitioning, and then your entire vector strategy, which is what we specialize in. We have seen great results. You get the best of all worlds. For example, there is a higher recall at pretty amazing requests per second, which in some cases is 10x to 15x of what you would get for the same recall with another engine. Your latency is also a lot lower. In some cases, it is incredibly low. For example, it is 10x to 15x lower than others. This combination is very hard to get with other databases that we have tried, so from that angle, Azure Cosmos DB has done a terrific job.
A few years ago, we put out a report that took Azure Cosmos DB as it is and compared it with other databases out there, and it was 92% cheaper on reads and 20% cheaper on writes. After that, we used our optimization, and we were able to further reduce that by another 75% to 99% in different cases. We have an online talk about it where we partially show how to get there. Those are not full solutions. It was a conference where I had 15 minutes, and I ended up doing a demo.
It is pretty fascinating because it is very hard for other databases to come anything close to it in terms of the cost given the fact that you have pretty amazing performance and scale. A lot of people can beat you on Azure Cosmos DB, but they do not give you the right performance and scale you need for business, so those cost savings are meaningless. For our customers, it has got to be the best of all worlds, and fortunately, Azure Cosmos DB has that.
What is most valuable?
Pretty much all of the features are valuable. Change feed is a pretty amazing feature. Once you make the changes, they are quickly read for you, and then you also have geo-replication. You can do a lot of things in your region, and the same regions can be replicated all over the world. There are different geographies. I can have my servers pretty much anywhere in the world. The data could be within the country or continent when there is a data restriction policy and things like that. Security is big. There are a lot of very good features.
It is very easy and very simple now given all the improvements, but it is also designed very well. Especially because we specialize in it, it is the easiest thing on our side. Data modeling happens to be a lot easier than SQL and others. The learning curve is a lot smaller than a typical RDBMS. It is very like code, and that is another benefit. Developers love it because you do not have to learn something new. You can use the same object that you are using in your code, and you can write stored procedures in JavaScript if you want to. If you want to do anything else, you could use the SQL API or NoSQL API, or you could use MongoDB API. It supports a lot of different APIs. You do not have to learn anything new, so the learning curve is way smaller than pretty much anything out there.
The best part of Azure Cosmos DB is that you barely have any maintenance. This is what I liked about it in the first place.
What needs improvement?
In the long run, there should be an addition of more features, especially because this space is evolving quickly. It all boils down to how many more features you are adding, how many integrations you are supporting, and how many more APIs you have that are standard APIs. The team is already doing a great job. They are already doing all that is needed, but the more features we have, the easier it is for us and our clients.
For example, when you have these vectors, it requires us to know a little bit about the configuration behind things such as HNSW. When it comes to the MongoDB vCore piece of Azure Cosmos DB, people like us know how to get to higher recalls easily but a regular user may not. If they have a feature that provides an easy way to get to a certain recall you need, and that is a configuration by default, that would be great. Currently, the flexibility is amazing, and we love that. The competition is usually not providing that. The competition sometimes gives you a recall of 80%, but they are taking away the latency and requests per second. Azure Cosmos DB does not do that. It is a better solution. If Azure Cosmos DB has configurations and a feature allowing us to pick any of the use cases we want, it would be great. For example, if I have an application that I am okay with, and my application does not require a huge recall that is 80% but needs one that is 60%, for us, it is very easy to take HNSW and do it that way and reduce the requests per second because that might not be a concern. If there is a feature that allows people to pick out of five different permutations and combinations, it would be very easy for anyone else to do it. However, keep in mind that competition does not even have that flexibility, so competition is lagging behind on that, at least in the case of the ones we have tried. If Azure Cosmos DB has such a feature, it will be easy for more people to take advantage of the things we are taking advantage of.
For how long have I used the solution?
I started using it when the first version of DocumentDB came out, and then a couple of years later, it was renamed to Azure Cosmos DB. I have been working with it even before it was called Azure Cosmos DB. I am still using it, and it is my favorite database.
What do I think about the stability of the solution?
In 2020, we put out a report. It is on our website. This was the only one out of all the major databases out there that had a linear throughput increase for hundreds of servers. What is crazy is that everyone else said that they do it, but you could literally see that after a certain number, they would slow down. One of them was a pretty majorly known, multibillion-dollar NoSQL database, but after 50 servers, it would just slow down completely. You could literally say, "Wow, it is taking time to go to the next level." Azure Cosmos DB was the only one that had linear throughput. Our team thinks that the underlying infrastructure of Azure Cosmos DB is procured in advance, and that is why they can have a linear scale for as much as you want to go. It totally depends on how big, literally, Azure is. The competition is running on someone else's cloud mostly. They probably procure machines as they go, and maybe that is why they are slow. This is an interesting thing that made us love Azure Cosmos DB.
Latency is pretty straightforward. They guarantee 10 milliseconds read and write times now, which used to be 10 and 15 earlier. That is one thing that is pretty incredible. A few things that we have shared with customers is that there is always a wrong way of doing things. You may know the right thing, and it is easy for you to get the latencies, but you may completely mess up the data modeling as well as your code and hundreds of different things. You may be making five calls when you need to make one. You may be doing a lot of other things that are not necessarily best practices. In some cases, we have seen people having a latency of more than 200 milliseconds, which we brought down to 10 milliseconds.
What do I think about the scalability of the solution?
Azure Cosmos DB’s dynamic scaling decreases an organization’s overhead costs big time. This is where it stands out. A lot of our clients who were previously using RDBMS kind of solutions found them to be slow. It would take forever. They would not scale beyond a certain point, and they would get extremely costly after some time because you have huge machines. Some of them were paying millions of dollars for one machine, whereas now, they just pay and go and they can scale. They can go all the way up, and if they want to go down, they go down. For example, on Thanksgiving, they may be scaling to hundreds of nodes behind the scenes, and the next day, they could be scaling back to three nodes. Imagine the cost savings when you never had to procure those servers or anything else. This is the genius of this whole thing. They are being able to take advantage of a scale that they could have never had as an organization. This is not just for startups. Even big corporations can take advantage of the same exact thing. It works for every single one out there.
How are customer service and support?
I never had to contact support. If you know what you are doing, then it is really good. I do not even know of anyone calling Azure Cosmos DB support for anything.
Which solution did I use previously and why did I switch?
I have worked with almost all of the competitor solutions. I cannot think of any disadvantages of Azure Cosmos DB unless the competition is for a specific use case. For example, in the beginning, Redis was great for caching. It had a great Pub/Sub Over a period of time, Cosmos DB got there, but Azure Cosmos DB is a lot more than just that use case. Today, it is easy for me to pick Azure Cosmos DB as a caching engine. I would not have done that five or six years ago.
How was the initial setup?
We do not do much with the on-premises version. We only work with the cloud version.
Its deployment is pretty easy. I have been doing it for a long while, so it is easy for us and our clients. I do not know about others.
Our implementation strategy depends on what kind of project it is. We do greenfield, brownfield, and all of the projects. We do integration projects. We also do projects where they are only doing an addition of LLM. We start with understanding the client's needs and then figuring out what is currently there. If there is nothing, we data model the whole thing from scratch and go with the best practices. A lot of times, if it is brownfield, a bunch of work is already done, so we are not going and figuring out what is the most optimum way to do it within client constraints. We create a strategy based on that and implement it based on their tech stack because everyone has a different one. Once we get the approval on that, we move forward with the implementation.
The number of people required and the time required depend on the client and their workload. If you have a small app, you could be onboarded on day one. If you have a big app with petabytes of data, it is usually a month of work. It totally depends on what we are looking at or the use case.
In terms of the learning curve, Azure Cosmos DB is one of the simplest ones out there. It is on the easier side. There are a couple of them that are pretty easy.
What other advice do I have?
To new users, I would advise understanding different propositions. Start with understanding what kind of data set you have and every single thing. Also, know the tech stack you have and pick your strategy accordingly. What you do not want to do is go with the flow without understanding what a NoSQL database is supposed to be like and make changes down the line.
A lot of people with an SQL background, unfortunately, start using any NoSQL databases, not just Azure Cosmos DB, in a way that is not very good for them because the patterns that usually are the best patterns for SQL may not be the best patterns for NoSQL. For example, there is a reason we do normalization in SQL. That takes away duplicate data, which is perfectly okay, but in the case of NoSQL or Azure Cosmos DB, we can scale and have duplicate data in places if we have a different kind of use case. If I want to make different kinds of searches available, I can have three different kinds of searches available for similar kinds of parameters. I will not be worried about doing that in a NoSQL environment because I can scale out pretty easily, so data does not hold me back. In an RDBMS environment, I might be doing two or three joins to make sure that I am making it fully normalized because if my data increases drastically, that will create a scale up situation. Scale-up is the only thing you can literally do with RDBMSs. Mostly, scale-out is not that easy unless you are on the cloud and you are using the scale of the cloud, and then you have performance issues. In those kinds of different scenarios, the DBAs or people with an RDBMS background need to come up with an open mind and understand what this is.
It is not that you have to learn a lot about Azure Cosmos DB. You will have to learn about this new paradigm. It is not very new. It has been going on for more than a decade. We have been doing it for more than a decade, but we see a lot of people coming from an RDBMS background and getting it wrong, and then you're paying people like us a lot more money to fix it. It is easier to work with someone like us in the beginning or do a little bit more due diligence and learn that paradigm before you get started. That will save you a lot of money and time, and hopefully, you will not need our services at that point of time. That is definitely my advice.
I would rate Azure Cosmos DB a ten out of ten at this time.
Disclosure: PeerSpot contacted the reviewer to collect the review and to validate authenticity. The reviewer was referred by the vendor, but the review is not subject to editing or approval by the vendor. The reviewer's company has a business relationship with this vendor other than being a customer: Implementer
Last updated: Sep 19, 2024
Flag as inappropriateCEO at Interloop Data
Enables us to handle transactional and analytical workloads in the same database
Pros and Cons
- "We love the ability to land data with Cosmos DB easily. Cosmos is native to Azure, so everything works seamlessly with it. You need good data to have good AI, and Cosmos makes it easy to land the data."
- "We have both our SaaS app and the analytical side running without throttling issues."
- "We would like to see advancements in AI with the ability to benchmark vector search capabilities, ensuring it answers questions accurately. During our initial implementation, we faced challenges with indexing and sorting, which are natively available in other offerings but required specific configurations in Cosmos."
- "We would like to see advancements in AI with the ability to benchmark vector search capabilities, ensuring it answers questions accurately. During our initial implementation, we faced challenges with indexing and sorting, which are natively available in other offerings but required specific configurations in Cosmos."
What is our primary use case?
Our corporate mission is to help companies achieve more with their data, which often means unifying your data. We have a SaaS solution and have built a Copilot with Copilot Studio on top, as well as some of the Azure AI services, which is now Foundry. We are starting to use it to allow people to use natural language to ask questions of their data. We are early in our journey, but I suspect it will work well for us.
How has it helped my organization?
By incorporating Cosmos DB into our Azure ecosystem, we have streamlined costs and improved efficiency. The integration has allowed us to manage Cosmos alongside our other services, providing a comprehensive view of resources. The inclusion of advanced capabilities has been beneficial, positively impacting our internal operations and the services we offer to clients.
We're early in our journey, but I believe it will improve the quality of our search results. We're having a lot of success with Copilot and are excited to see how it'll work in a traditional sense as well. We're in analytics, so we work with a lot of massive data and look at tens of millions of rows. We haven't had any capacity challenges or thresholds. We started with small data, so having a tool that will grow with you is great.
What is most valuable?
We love the ability to land data with Cosmos DB easily. Cosmos is native to Azure, so everything works seamlessly with it. You need good data to have good AI, and Cosmos makes it easy to land the data.
The recently added ability to mirror to Fabric has been beneficial. Cosmos DB enables us to handle transactional and analytical workloads in the same database.
Cosmos DB is easy to use. You can set up a database with a couple of clicks, and it's simple to scale it up and down based on your needs. Within Azure, the Explorer UX has been great for us, too. You don't have to install another tool to run a quick query or explore some data. Additionally, the ability to estimate your Cosmos costs through the portal and manage features has been useful.
Like most database tools, it takes some time to understand. If you come from SQL or even from the Mongo world, many concepts will be familiar to you. While it takes some learning and expertise, it's not a large hill to climb. You must learn the advanced capabilities, but they make your solutions more powerful.
The vector database requires an additional engineering step to move the data from a transactional database to a vector store so that you can query it and use it in AI. However, because the vector capabilities are built in, it saved us engineering time and allowed us to get our solution out faster.
What needs improvement?
We would like to see advancements in AI with the ability to benchmark vector search capabilities, ensuring it answers questions accurately. During our initial implementation, we faced challenges with indexing and sorting, which are natively available in other offerings but required specific configurations in Cosmos.
For how long have I used the solution?
We have used Cosmos SQL for more than five years.
What do I think about the stability of the solution?
There have been no challenges with Cosmos DB's stability. We have both our SaaS app and the analytical side running without throttling issues.
What do I think about the scalability of the solution?
The scalability is great, both horizontally and vertically.
Which solution did I use previously and why did I switch?
In the past, we've worked with traditional SQL Server and MongoDB. However, Cosmos being native to Azure and the seamless integration prompted our switch.
How was the initial setup?
The onboarding process was relatively quick for us. We were up and running within two weeks, including a pilot test.
What was our ROI?
The dynamic scaling during peak times has been crucial in cost management.
What's my experience with pricing, setup cost, and licensing?
The integration of Cosmos with our other Azure services allows us to manage costs proactively. The built-in capabilities help control costs in line with our growth expectations through the portal.
What other advice do I have?
I rate Cosmos DB eight out of 10.
Which deployment model are you using for this solution?
Public Cloud
If public cloud, private cloud, or hybrid cloud, which cloud provider do you use?
Microsoft Azure
Disclosure: My company has a business relationship with this vendor other than being a customer: Reseller
Last updated: Dec 18, 2024
Flag as inappropriateApplication Development Analyst at Accenture
Provides multi-region storage, low latency, and automatic scaling
Pros and Cons
- "In Microsoft Azure Cosmos DB, one valuable feature is its ability to store data in multiple regions. If one region fails, it automatically switches to a healthy region, ensuring minimal latency and disaster recovery without impacting data latency in applications."
- "Microsoft Azure Cosmos DB is very easy to use."
- "Currently, I have no suggestions for enhancement or new implementations in Microsoft Azure Cosmos DB. However, the cost can sometimes be high, especially during cross-partition queries with large data amounts."
- "The cost can sometimes be high, especially during cross-partition queries with large data amounts."
What is our primary use case?
We use Microsoft Azure Cosmos DB to store document-type data, graph data, and key-value type data. It is a globally distributed database, which we mainly utilize to store document-type JSON data.
In my project, I work with core SQL-type queries. Using the API, we are storing JSON data in Microsoft Azure Cosmos DB with a database and container-level architecture. This involves storing items using a partition key for optimized query performance.
We get data from BLOB storage. After some processing, we are storing it in the JSON format in Microsoft Azure Cosmos DB.
How has it helped my organization?
Microsoft Azure Cosmos DB automatically indexes documents. By indexing every field in the document, it is easy to get fast performance to retrieve the records. While fetching, it fetches only specific fields required for further processing, which makes it efficient. Fetching all the fields from a document takes more time.
Storing data with a partition key makes data fetching easier and faster.
Microsoft Azure Cosmos DB helps in fetching data faster. There is a single-digit millisecond response to fetch those records.
Microsoft Azure Cosmos DB supports scalability. At a peak time, it will automatically scale the RUs. When there is less data, it will decrease them.
What is most valuable?
In Microsoft Azure Cosmos DB, one valuable feature is its ability to store data in multiple regions. If one region fails, it automatically switches to a healthy region, ensuring minimal latency and disaster recovery without impacting data latency in applications. It scales automatically based on query performance and peak traffic.
Microsoft Azure Cosmos DB is very easy to use.
What needs improvement?
Currently, I have no suggestions for enhancement or new implementations in Microsoft Azure Cosmos DB. However, the cost can sometimes be high, especially during cross-partition queries with large data amounts.
For how long have I used the solution?
I have been using Microsoft Azure Cosmos DB for the last two years.
What do I think about the stability of the solution?
Microsoft Azure Cosmos DB provides high availability with 99.9% reliability. When we store documents in Microsoft Azure Cosmos DB, it stores them in multiple regions, not only at specific regions. If one region fails, it automatically switches to a healthy region.
There is low latency. The partition key helps achieve low latency by ensuring data is stored and accessed efficiently.
What do I think about the scalability of the solution?
Microsoft Azure Cosmos DB offers both automatic and manual scaling. The automatic scaling feature adjusts RUs based on peak demands, which helps manage workloads efficiently. The dynamic scaling feature has helped reduce overhead costs by automatically managing resource utilization.
Our application is being used globally, and we have ten members in our team.
Which solution did I use previously and why did I switch?
When I joined the organization, Microsoft Azure Cosmos DB was already in use. I have not worked with other NoSQL databases before.
How was the initial setup?
It is a Platform as a Service. It was already implemented before I joined.
I started working with it in the first month. I had the support of the senior developers of the time.
It does not require maintenance from our end.
What was our ROI?
We monitor the cost daily through Azure Monitor to evaluate how much it is costing for documents, thereby keeping track of the return on investment.
What's my experience with pricing, setup cost, and licensing?
Microsoft Azure Cosmos DB pricing is based on RUs. Reading 1 KB document costs one RU, whereas writing one document costs five RUs. Pricing for querying depends on the complexity of the query. If you increase the document size, it will automatically increase the RU cost.
What other advice do I have?
I would recommend this solution. For e-commerce applications, it is more beneficial because it can store semi-structured data. It is the best option if you want to get data quickly because it organizes the data in a good way. When a region fails, it automatically switches to a healthy region. It has backup storage, and it scales automatically based on the peak time or low time.
I would rate Microsoft Azure Cosmos DB an eight out of ten. It is a good solution, but the cost can increase with cross-partition queries due to data distribution.
Disclosure: PeerSpot contacted the reviewer to collect the review and to validate authenticity. The reviewer was referred by the vendor, but the review is not subject to editing or approval by the vendor.
Last updated: Jan 23, 2025
Flag as inappropriateLead Software Architect at CPower
The ability to scale efficiently improves our performance and scalability
Pros and Cons
- "Change notification works well, and the ability to process documents in a scalable way is important. This means we can efficiently thread out different operations and meet our organizational performance and scalability needs."
- "Scaling the workloads is one of the key advantages of Cosmos, preventing the database from becoming a performance bottleneck."
- "One area that could be improved is indexing. Some of the developers struggle with the way the indexing works. We are exploring vector indexing, which we haven't examined fully yet. Indexing is an aspect we're looking to improve upon potentially."
What is our primary use case?
We are using Cosmos DB in several different ways. We receive unstructured and semi-structured documents from partners, and we use Cosmos DB to push the data in and scale it to kick off internal processes.
We receive notifications from our customers to take action quickly regarding the energy grid. Cosmos DB is also used in a different project for our settlement system, where it is used as a queuing engine for the change notification portion.
How has it helped my organization?
The ability to scale efficiently improves our performance and scalability. Although we haven't yet used Cosmos to improve search result quality, we believe it can be useful with vector search and data architecture improvements. We are exploring AI, but I don't think our focus will be generative. We do a lot of ML models, and we plan to restructure our data to use the data lake or leverage the efficiency of already created models to reduce our resource costs and improve efficiency.
What is most valuable?
Change notification works well, and the ability to process documents in a scalable way is important. This means we can efficiently thread out different operations and meet our organizational performance and scalability needs.
Cosmos DB is pretty straightforward. I'm not 100 percent an expert. I have three or four different developers up to speed on it and working on it. They do most of the daily operations, while I do a lot of the prototyping and conceptual aspects.
While we don't use the vector database system, some interesting features might benefit our future data architecture. In one of the workshops, we learned about its capabilities and how it's used as part of Copilot and the backend database. I'm thinking about AI, our data, and some performance benefits.
What needs improvement?
One area that could be improved is indexing. Some of the developers struggle with the way the indexing works. We are exploring vector indexing, which we haven't examined fully yet. Indexing is an aspect we're looking to improve upon potentially.
For how long have I used the solution?
I started dabbling in Cosmos before COVID approximately four or five years ago. Initially, I just wanted to test some concepts and figure out its benefits, using the Cosmos local engine to better understand its functionality.
What do I think about the stability of the solution?
We have not encountered any issues with latency or availability. As we continue to grow and scale, we will keep assessing to ensure our expectations are met.
What do I think about the scalability of the solution?
Scaling the workloads is one of the key advantages of Cosmos, preventing the database from becoming a performance bottleneck.
Which solution did I use previously and why did I switch?
We assessed other databases like MongoDB but chose Cosmos for its object-style database capabilities, user-friendliness, and ease of access. It aligned well with our needs, and a Microsoft conference initially piqued our interest.
How was the initial setup?
Onboarding to proficiency took a couple of months. The transition from a traditional relational database programmer to an object database was straightforward. The learning curve was manageable and engaging.
What was our ROI?
I don't know how much money Cosmos DB has saved us. We're still using some of the old databases, but when phase them out, we'll see a significant cost reduction.
What's my experience with pricing, setup cost, and licensing?
The pricing model aligns with our budget. It's expected to lower overhead costs, especially as we phase out older databases. Cosmos DB is great compared to other databases because we can reduce the cost while doing the same things.
Which other solutions did I evaluate?
We considered Mongo DB among other databases, but Cosmos had the desirable capabilities we were seeking.
What other advice do I have?
I rate Cosmos DB eight out of 10.
Disclosure: I am a real user, and this review is based on my own experience and opinions.
Last updated: Dec 16, 2024
Flag as inappropriateVice President, Machine Learning at a healthcare company with 10,001+ employees
The real-time analytics capabilities allow for turnaround times in milliseconds
Pros and Cons
- "The most valuable feature of Microsoft Azure Cosmos DB is its real-time analytics capabilities, which allow for turnaround times in milliseconds. This is crucial for applications like fraud detection."
- "The most valuable feature of Microsoft Azure Cosmos DB is its real-time analytics capabilities, which allow for turnaround times in milliseconds."
- "It would be beneficial if Cosmos supported batch and real-time use cases to make the system more seamless."
- "If you want to bring the data from AWS, you must pay data egress costs. That's a pain point."
What is our primary use case?
We have numerous healthcare AI use cases, including utilization management, documentation, letter generation, and voice call creation. These are both real-time and non-real-time use cases. My team is the platform team that enables the services. The ML teams are the practitioners who work on these products.
How has it helped my organization?
The vector database has had a significant impact by making everything searchable, and the number of potential use cases exploded when GenAI was added. We've transformed many tasks into AI machine-learning problems. We have a ton of institutional expertise across the enterprise. It's crucial to be able to bring all of that into one place, ask questions, and get answers.
What is most valuable?
The most valuable feature of Microsoft Azure Cosmos DB is its real-time analytics capabilities, which allow for turnaround times in milliseconds. This is crucial for applications like fraud detection.
Using and optimizing Cosmos DB is relatively straightforward. We talk regularly with the Microsoft team, and hands-on help is available when needed, so the experience was seamless.
We have migrated to Cosmos' vector database search from Azure AI Search. We don't face too many challenges with interoperability because everything is built on Azure, and we don't have any multi-cloud applications.
Azure AI services integrate and perform well with the vector database. Sometimes, we struggle to customize the RAG pipeline instead of using the embedded settings. Those are rare use cases, but they are useful for most use cases.
The search capabilities work well once you have your data set up. It's more of a challenge in the knowledge-based integration than the modeling side. Our data is scattered. SharePoint, Confluence, and meeting minutes data are separate. We are working actively to make all the data flow.
What needs improvement?
It would be beneficial if Cosmos supported batch and real-time use cases to make the system more seamless. Our biggest challenge migrating data is the fact that we're a multi-cloud organization with data stored in multiple platforms like AWS and Snowflake. It's all over the place, so we are using solutions like Fabric to migrate the data. If you want to bring the data from AWS, you must pay data egress costs. That's a pain point.
For how long have I used the solution?
I have been using Microsoft Azure Cosmos DB for about two and a half years.
What do I think about the stability of the solution?
The latency numbers of Cosmos DB are satisfactory and align with expectations for clinical decision support engines.
What do I think about the scalability of the solution?
While I have not personally tested it, the information I have suggests that Cosmos DB has robust scalability capabilities.
How are customer service and support?
We have regular connections with the Microsoft team, which provides hands-on support and makes the use of Cosmos DB straightforward.
How would you rate customer service and support?
Positive
Which solution did I use previously and why did I switch?
We previously used Azure AI Search, but we are transitioning to utilize built-in capabilities in Cosmos DB.
What about the implementation team?
The platform team is responsible for enabling the services, while the ML teams use these products.
What's my experience with pricing, setup cost, and licensing?
The pricing model aligns with our budget expectations, and we get a significant corporate discount from Microsoft because we're a partner.
What other advice do I have?
I would rate Microsoft Azure Cosmos DB eight out of 10.
Which deployment model are you using for this solution?
Public Cloud
If public cloud, private cloud, or hybrid cloud, which cloud provider do you use?
Microsoft Azure
Disclosure: My company has a business relationship with this vendor other than being a customer:
Last updated: Nov 27, 2024
Flag as inappropriateProject Associate at a consultancy with 10,001+ employees
The high speed compared to other competitors is remarkable
Pros and Cons
- "The high speed of Azure Cosmos DB compared to other competitors is remarkable."
- "The high speed of Azure Cosmos DB compared to other competitors is remarkable."
- "Overall, it is a good resource. I am not aware of the background, but it seems to currently support only JSON documents."
- "Azure Cosmos DB is generally a costly resource compared to other Azure resources. It comes with a high cost."
What is our primary use case?
I am using it to store our data. We are using Azure Cosmos DB to store our JSON-based documents.
What is most valuable?
The high speed of Azure Cosmos DB compared to other competitors is remarkable. It is one of the most powerful features, offering high availability and high speed. Its benefits can be seen immediately after the deployment.
What needs improvement?
Overall, it is a good resource. I am not aware of the background, but it seems to currently support only JSON documents. They could expand their scope to support other types of data, such as XML or EDI formats. EDI is an old technology, but it is still in high use in supply chain and retail industries.
For how long have I used the solution?
I have more than two years of experience with Azure Cosmos DB, whereas with Azure, it has been more than four years.
What do I think about the stability of the solution?
Choosing the correct partition key is crucial, as it affects our database speed and related operations.
Latency and availability depend on the consistency level.
What do I think about the scalability of the solution?
It is a Platform as a Service, so we are concerned about the underlying interface. We can move to a higher tier as all Azure cloud resources are open to easy scaling.
Which solution did I use previously and why did I switch?
It offers an option alongside the Azure SQL database. Azure SQL database has its own capabilities, whereas Azure Cosmos DB supports all major big data requirements like Cassandra and Gremlin. Azure SQL database is more focused on transactional data instead of analytic data. Azure Cosmos DB covers a wider area.
How was the initial setup?
I have not personally deployed Azure Cosmos DB, but DevOps pipelines provide options for this. It should be easily deployable with the help of Microsoft's documentation.
It takes a couple of minutes to be up and running. It also depends on how we are deploying, whether it is via an ARM template, Azure pipeline, or directly via Azure release.
What's my experience with pricing, setup cost, and licensing?
Azure Cosmos DB is generally a costly resource compared to other Azure resources. It comes with a high cost. We have reserved one thousand RUs. Free usage is also limited.
What other advice do I have?
It is not like a traditional database. Choosing the partition key needs an understanding because it will affect the database speed. By making your partitions in a logical and efficient way, you can improve the speed of search analysis.
I would rate Azure Cosmos DB an eight out of ten.
Disclosure: My company has a business relationship with this vendor other than being a customer: Partner
Last updated: Jan 23, 2025
Flag as inappropriate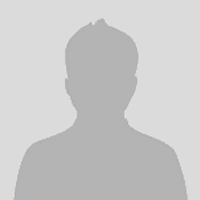
Buyer's Guide
Download our free Microsoft Azure Cosmos DB Report and get advice and tips from experienced pros
sharing their opinions.
Updated: February 2025
Product Categories
Managed NoSQL Databases Database as a Service (DBaaS) NoSQL Databases Vector DatabasesPopular Comparisons
Amazon DynamoDB
Amazon Neptune
Amazon DocumentDB
Google Cloud Bigtable
Amazon Timestream
Neo4j AuraDB
Oracle NoSQL Database Cloud
Buyer's Guide
Download our free Microsoft Azure Cosmos DB Report and get advice and tips from experienced pros
sharing their opinions.