The primary use case for Amazon SageMaker is leveraging its compute power, particularly for tasks like securing LMM notebooks using node instances. Additionally, its GPU capabilities are valuable for executing large language models. Users can create endpoints and access them from anywhere as needed.
Senior Project Lead at Intellect Design Arena
Has Studio Lab feature and useful for LLMs
Pros and Cons
- "We've had experience with unique ML projects using SageMaker. For example, we're developing a platform similar to ChatGPT that requires models. We utilize Amazon SageMaker to create endpoints for these models, making accessing them convenient as needed."
- "In my opinion, one improvement for Amazon SageMaker would be to offer serverless GPUs. Currently, we incur costs on an hourly basis. It would be beneficial if the tool could provide pay-as-you-go pricing based on endpoints."
What is our primary use case?
What is most valuable?
We've had experience with unique ML projects using SageMaker. For example, we're developing a platform similar to ChatGPT that requires models. We utilize Amazon SageMaker to create endpoints for these models, making accessing them convenient as needed.
The main function I prefer in Amazon SageMaker is the ability to create endpoints for large models. I haven't explored features like Studio Lab yet, but I've found the tutorials very helpful. The platform is user-friendly, with documentation attached to everything, making it easy to navigate and learn. Overall, I especially like the Studio Lab feature.
In the Studio Lab, tutorials provide direct snippets for tasks like connecting to S3 from Amazon SageMaker. These standard snippets make implementation straightforward and simplify the development process for me.
What needs improvement?
In my opinion, one improvement for Amazon SageMaker would be to offer serverless GPUs. Currently, we incur costs on an hourly basis. It would be beneficial if the tool could provide pay-as-you-go pricing based on endpoints.
In the three months I've been using it, I've noticed that higher GPU instances can be quite costly. To mitigate this cost impact, serverless GPUs would be beneficial.
For how long have I used the solution?
I have been working with the product for three months.
Buyer's Guide
Amazon SageMaker
April 2025
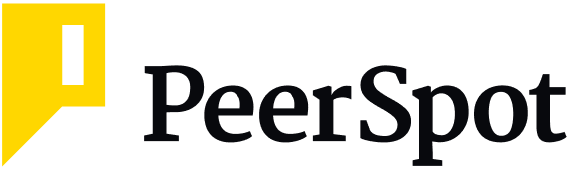
Learn what your peers think about Amazon SageMaker. Get advice and tips from experienced pros sharing their opinions. Updated: April 2025.
847,959 professionals have used our research since 2012.
What do I think about the stability of the solution?
I rate the solution's stability a nine out of ten.
What do I think about the scalability of the solution?
I rate the tool's scalability an eight out of ten. No issues with scalability as long as we ensure we have the necessary quotas in place before implementing a scalable process. I needed to request quota increases for certain services beforehand, and once those were provided, I could adjust the main and max nodes accordingly based on our planned requirements. My company has 25 users.
How are customer service and support?
We can schedule a direct call with the support team.
Which solution did I use previously and why did I switch?
Amazon SageMaker's Studio Lab feature differentiates it from products like Azure ML Studio. With Studio Lab, I can directly interact with the environment, making navigating and accessing documentation easier. In contrast, finding documentation and navigating Azure ML Studio was challenging.
However, we also use Azure for the Azure OpenEdge service, which operates on a pay-per-minute token basis. This payment model is not available in Amazon SageMaker.
How was the initial setup?
The initial setup and deployment process for Amazon SageMaker is straightforward. The only complexity I encountered was gaining access to the needed resources, which relied on coordination with the DevOps team. Once I had access sorted out, implementing my ideas for large language models and other models was comfortable.
What's my experience with pricing, setup cost, and licensing?
The tool's pricing is reasonable.
What other advice do I have?
I rate the overall solution an eight out of ten.
Disclosure: I am a real user, and this review is based on my own experience and opinions.
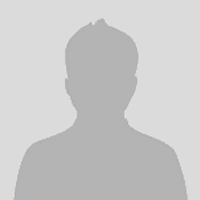
Executive Specialists at LineData
Enables quick development of AI models and improves the team’s productivity
Pros and Cons
- "We were able to use the product to automate processes."
- "The solution requires a lot of data to train the model."
What is our primary use case?
We use the solution to extract financial information and contractual data from unstructured documents.
What is most valuable?
The product provides the ability to develop AI models relatively quickly. My team develops the models using the tool. We use AI quite extensively in our business. We use the tool for predictive analytics. It helps predict which trade might fail based on historical data. Automatic Model Tuning helps improve the productivity of the investment operation team. Typically, an analyst spends about 45% of their time collecting, organizing, and ingesting data. We were able to use the product to automate processes.
What needs improvement?
The solution requires a lot of data to train the model.
For how long have I used the solution?
I have been using the solution for the past 12 months.
What do I think about the stability of the solution?
The tool’s stability is pretty high. I rate the stability a nine and a half or ten out of ten.
What do I think about the scalability of the solution?
The scalability is very high. I rate the scalability a nine out of ten. We are a small team of AI analysts. We have half a dozen users.
How are customer service and support?
The support is usually pretty responsive. The solution has a fair bit of content online. We haven't had any support challenges.
How would you rate customer service and support?
Positive
Which solution did I use previously and why did I switch?
We were using Azure’s tool before. We switched to Amazon SageMaker because it allows us to sell it to larger institutional clients. AWS is more prevalent in the broader institutional segment.
How was the initial setup?
The initial setup is relatively straightforward. The same team developing the models deploys and tests the solution. The tool requires a bit of ongoing maintenance. It is relatively easy to do.
What's my experience with pricing, setup cost, and licensing?
The product is expensive. I rate the pricing a five or six out of ten.
What other advice do I have?
We are partners and resellers. Overall, I rate the product a nine out of ten.
Disclosure: My company has a business relationship with this vendor other than being a customer: Reseller
Buyer's Guide
Amazon SageMaker
April 2025
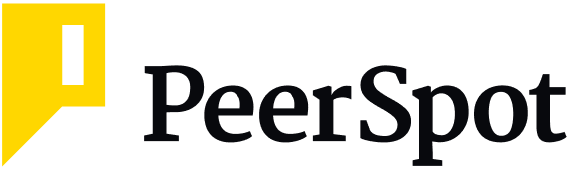
Learn what your peers think about Amazon SageMaker. Get advice and tips from experienced pros sharing their opinions. Updated: April 2025.
847,959 professionals have used our research since 2012.
Data Scientist at a computer software company with 501-1,000 employees
It’s low-price point makes it a great entry into machine learning, but it is difficult to learn to use
Pros and Cons
- "The superb thing that SageMaker brings is that it wraps everything well. It's got the deployment, the whole framework."
- "The solution is complex to use."
What is our primary use case?
I use SageMaker to use a "bring-your-own-model" setup. For SageMaker AutoML, we're fine and happy with it. It is restricted because you can't move through multiple algorithms. It seems to work only with two. One of the things I am doing is prototyping, and it's proving quite difficult to get our model working how we want it to. It's proving complex with many moving parts, and the documentation is only partially helpful. SageMaker requires a lot of work to get it working.
I spent the last four months trying to get a prototype working and exploring to bring in a model while exploring alternate models and making prototypes work. We've stepped back to AutoML for now. We might be using EKS, so we bring our containers. Within the containers, we can work with what we need to work with.
What is most valuable?
The superb thing that SageMaker brings is that it wraps everything well. It's got the deployment, the whole framework. When you couple it with step functions, you can do some very powerful things. It manages deployment, you have model monitoring, and you have model quality checks. It's got a lot of end-to-end services one needs to get a full machine-learning pipeline running. While I say that I had a struggle and blame the product partially, I am also impressed with the ecosystem. I would still use it over and above other competing products, but I don't know the Google setup. I have worked very briefly with Azure, so I can't do a proper card-to-card comparison, but I do like the ecosystems AWS brings. If a client came along and asked me to set up a machine learning ecosystem, a full machine learning production deployment, I would use Sagemaker.
What needs improvement?
The solution is complex to use.
Some additional functionality would be for them to provide sample end-to-end card formation templates and try to unify the setup. At the moment, as you move from one set of documentation to the next, some of the documentation is for bringing your model, some of the documentation is for SDK, some of it's for API, some of it's for command lines, and some of it's for step functions. None of the documentation seems to be end-to-end. There are gaps in the documentation. It proves to require a lot of digging from the user to figure it out. I did get through the AWS machine learning specialty certification, but that proved to be a bit superficial. Though it covered a lot of ground, it didn't have the detail one would need for Sagemaker. I am self-taught in a lot of the stuff. I could dive deeper into some code and take time to get examples running. But I was consulting a startup, and they needed to move quickly.
I was hoping SageMaker would be easier to work with because I was expecting there would be examples we could repurpose that were more complete.
The new functionality I'd like to see is Amazon tuning attention to the documentation sets and the templates.
For how long have I used the solution?
I've been using Amazon SageMaker for about two and a half years.
What do I think about the stability of the solution?
Stability is not a relevant metric anymore because SageMaker runs on its own underlying AWS serverless infrastructure, which is 100% reliable.
Folks better than me with more extensive resources and time have run and checked SageMaker ten times a second every second for 1,000 hours to see if they got a drop, but they haven't. It's serverless and bulletproof.
What do I think about the scalability of the solution?
I rate the scalability a ten out of ten. Part of the problem is that AWS has limited the functionality of SageMaker in many ways to make it scalable. So it's scalability first and then functionality second.
The solution works well for medium-sized businesses and up. But even for small businesses, you can do some simple and quick elastic endpoints and get going quickly. The problem is the amount of work it takes for people to know what they're doing with Sagemaker, and those people are probably rare. I've been able to get things up and running in most cases in all sorts of AWS services, but I'm struggling. Small, medium, and large enterprises could use SageMaker with an automatic model, but it depends on the people's skills doing the deployment. A small business probably couldn't afford contractors, consultants, or data scientists. It's not about AWS. It's a problem with classic data science skills.
How was the initial setup?
I rate the initial setup a three or two out of ten because it's very complex.
What's my experience with pricing, setup cost, and licensing?
You don't pay for Sagemaker. You only pay for the compute instances in your storage. SageMaker is free.
Which other solutions did I evaluate?
I've had a little bit of a look at Azure, but I didn't get into the level of detail I did with Sagemaker. I have worked reasonably intentionally with DataRobot and H20. But SageMaker is a way bigger, way more capable platform. The AutoML is very simple, and it is much, much cheaper. The cost of SageMaker is nothing. By contrast, if you're using DataRobot, you'll pay $100,000 plus for a five-year license.
What other advice do I have?
Anyone doing on-prem at the moment for anything but their core datasets or legacy systems that can't be moved is just paying useless money.
I rate Amazon SageMaker a seven out of ten. I'd recommend it to other users. It's worth syncing the time and effort into getting it running.
Which deployment model are you using for this solution?
Public Cloud
If public cloud, private cloud, or hybrid cloud, which cloud provider do you use?
Amazon Web Services (AWS)
Disclosure: I am a real user, and this review is based on my own experience and opinions.
Senior Technical Architect; Head of Platform at Blenheim Chalcot IT Services India
Allows for generating high-quality models without needing extensive coding knowledge
Pros and Cons
- "The most valuable features in Amazon SageMaker are its AutoML, feature store, and automated hyperparameter tuning capabilities."
- "Improvements are needed in terms of complexity, data security, and access policy integration in Amazon SageMaker."
What is our primary use case?
The primary use cases for Amazon SageMaker are for EDA processing, ML model building, setting up MLOps, predictive analysis, customer churn models, fraud detection, image and video analysis, as well as NLP projects. It is a versatile tool in the machine-learning landscape.
What is most valuable?
The most valuable features in Amazon SageMaker are its AutoML, feature store, and automated hyperparameter tuning capabilities. These features allow for generating high-quality models without needing extensive coding knowledge, making it accessible for non-experts. SageMaker helps in end-to-end machine learning, incorporating data preparation, model deployment, and continuous monitoring.
What needs improvement?
Improvements are needed in terms of complexity, data security, and access policy integration in Amazon SageMaker. It is considered complex to integrate these aspects, and adjustments need to be made in multiple places, which should be more user-friendly. A centralized interface for managing these configurations is desired.
For how long have I used the solution?
I have been working with Amazon SageMaker for nearly three years.
What do I think about the stability of the solution?
Amazon SageMaker's stability depends on how well-configured the entire setup is. Due to the interconnected dependencies within the system, the learning curve may be steep for new users. However, with proper configuration, the overall stability is adequate.
What do I think about the scalability of the solution?
Amazon SageMaker offers a high level of scalability. It allows dynamic resource allocation and supports large datasets through various features like multi-model endpoints and flexible instance configuration, scaling up or down according to requirements.
How are customer service and support?
Technical support for Amazon SageMaker involves communication through web chats or telephone based on the support agreement.
How would you rate customer service and support?
Positive
Which solution did I use previously and why did I switch?
In AWS, we used to build the whole pipeline model by ourselves, component by component. With Amazon SageMaker, costs have been optimized as it includes pre-configured components that reduce overall expenses.
How was the initial setup?
The initial setup of Amazon SageMaker can be achieved quickly if the default configuration is used. However, setting it up more customizable, such as for specific requirements, can make the process time-consuming, earning an eight out of ten in terms of ease.
What about the implementation team?
A single knowledgeable person with expertise in ML and cloud can handle the deployment and maintenance of Amazon SageMaker.
What was our ROI?
We have seen a significant reduction in costs using Amazon SageMaker. Building any ML lifecycle benefits from SageMaker's pre-configured components, which bring down the overall cost compared to setting up all components separately.
What's my experience with pricing, setup cost, and licensing?
While Amazon SageMaker is expensive compared to other cloud vendors, certain cost optimizations can be made with proper setup and configuration knowledge. Greater visibility from AWS regarding cost-impacting configurations would be beneficial.
Which other solutions did I evaluate?
No other solutions were evaluated outside of AWS, as we were setting everything up within AWS before opting to use Amazon SageMaker.
What other advice do I have?
I rate SageMaker eight out of ten.
New users should conduct a pilot or proof of concept with Amazon SageMaker to see if it aligns with their business use cases. Evaluate and understand the integration with other AWS services and ensure the team has adequate knowledge to handle monitoring, model performance, and managing costs efficiently. Engaging with the community to remain updated on any misconfigurations is also advisable.
Which deployment model are you using for this solution?
Public Cloud
If public cloud, private cloud, or hybrid cloud, which cloud provider do you use?
Amazon Web Services (AWS)
Disclosure: I am a real user, and this review is based on my own experience and opinions.
Last updated: Nov 26, 2024
Flag as inappropriateData Science Manager / Chapter Lead at Afya
A managed AWS service that provides the tools to build, train and deploy machine learning models and collaborate using tools like GitLab
Pros and Cons
- "Amazon SageMaker is highly valuable for managing ML workloads. It connects to AWS cloud resources, making it easy to deploy algorithms and collaborate using tools like GitLab. It offers a wide range of Python libraries and other necessary tools for modelling and algorithms."
- "Amazon SageMaker can make it simpler to manage the data flow from start to finish, such as by integrating data, usingthe machine, and deploying models. This process could be more user-friendly compared to other tools. I would also like to improve integration with Bedrock and the LLM connection for AWS."
What is our primary use case?
Amazon SageMaker is a collaborative tool for our data science projects. It allows us to integrate efficiently, write and review code, and access all the necessary project tools.
What is most valuable?
Amazon SageMaker is highly valuable for managing ML workloads. It connects to AWS cloud resources, making it easy to deploy algorithms and collaborate using tools like GitLab. It offers a wide range of Python libraries and other necessary tools for modeling and algorithms.
What needs improvement?
Amazon SageMaker can make it simpler to manage the data flow from start to finish, such as by integrating data, usingthe machine, and deploying models. This process could be more user-friendly compared to other tools. I would also like to improve integration with Bedrock and the LLM connection for AWS.
For how long have I used the solution?
I have been using Amazon SageMaker for the past two years.
What do I think about the scalability of the solution?
I've never encountered issues with SageMaker's scalability. AWS provides all the necessary resources in terms of power and capacity.
How was the initial setup?
The initial setup is straightforward. We have a team from the infrastructure department that ensures the system runs smoothly. The data science team also plays a role in monitoring the effectiveness of the models. The deployment process usually takes two to three months for the whole project, with various strategies involved. SageMaker integrates well with AWS features, and when deploying, I typically set up APIs to make the model accessible to other systems and connect it with GitLab for easier model control.
What's my experience with pricing, setup cost, and licensing?
In terms of pricing, I'd also rate it ten out of ten because it's been beneficial compared to other solutions.
What other advice do I have?
I would rate Amazon SageMaker a nine out of ten because while it has all the necessary features, there could be improvements in making the data flow more manageable.
Which deployment model are you using for this solution?
Private Cloud
Disclosure: I am a real user, and this review is based on my own experience and opinions.
Machine Learning Engineer at TechMinfy
Has hyperparameter tuning which helps to save time
Pros and Cons
- "The most tool's valuable feature, in my experience, is hyperparameter tuning. It allows us to test different parameters for the same model in parallel, which helps us quickly identify the configuration that yields the highest accuracy. This parallel computing capability saves us a lot of time."
- "One area where Amazon SageMaker could improve is its pricing. The high costs can drive companies to explore other cloud options. Additionally, while generally good, the updates sometimes come with bugs, and the documentation could be much better. More examples and clearer guidance would be helpful."
What is our primary use case?
We use Amazon SageMaker primarily for training and deploying end-to-end models for our specific use cases. We take models from the interface and deploy them to the staging environment, ensuring they are monitored 24/7. This tool is essential for deploying models.
What is most valuable?
The most tool's valuable feature, in my experience, is hyperparameter tuning. It allows us to test different parameters for the same model in parallel, which helps us quickly identify the configuration that yields the highest accuracy. This parallel computing capability saves us a lot of time.
What needs improvement?
One area where Amazon SageMaker could improve is its pricing. The high costs can drive companies to explore other cloud options. Additionally, while generally good, the updates sometimes come with bugs, and the documentation could be much better. More examples and clearer guidance would be helpful.
For how long have I used the solution?
I have been working with the product for six to seven months.
What do I think about the stability of the solution?
The solution is a stable product.
What do I think about the scalability of the solution?
My company has 300 to 400 users. The solution is scalable.
How are customer service and support?
We contacted AWS support, and we are happy with them.
Which solution did I use previously and why did I switch?
We are AWS partners and blindly go with AWS products.
How was the initial setup?
Regarding the initial installation, setup, and deployment, I would rate it as medium difficulty. Since it operates within the AWS ecosystem, you must follow specific rules and understand how AWS works. It can take around four to five months to fully deploy a model, understand its running and training processes, and get everything set up properly.
What other advice do I have?
If you want to use Amazon SageMaker for the first time, I would advise completing one of the AWS certifications and reading the documentation thoroughly. Having someone experienced with the product to guide you can also be very helpful.
Despite its high price, the tool is continually evolving, and updates are frequent and relevant. However, due to its pricing and some issues, I would rate it a seven out of ten.
If public cloud, private cloud, or hybrid cloud, which cloud provider do you use?
Amazon Web Services (AWS)
Disclosure: My company has a business relationship with this vendor other than being a customer:
Last updated: Jul 25, 2024
Flag as inappropriateData specialist at a mining and metals company with 11-50 employees
Easier and faster than manually coding everything in Python
Pros and Cons
- "The Autopilot feature is really good because it's helpful for people who don't have much experience with coding or data pipelines. When we suggest SageMaker to clients, they don't have to go through all the steps manually. They can leverage Autopilot to choose variables, run experiments, and monitor costs. The results are also pretty accurate."
- "The training modules could be enhanced. We had to take in-person training to fully understand SageMaker, and while the trainers were great, I think more comprehensive online modules would be helpful."
What is our primary use case?
I use it for modeling large amounts of production data. We don't have the time and it's a large amount of production data. So, it's not physically possible to eliminate or find the co-relations, run it through, basically setting and coding in Python. So it's much easier.
You just have your drag and drop. So if you have the Python knowledge for that, it's very good. We basically suggest that these people to use it as well.
What is most valuable?
The Autopilot feature is really good because it's helpful for people who don't have much experience with coding or data pipelines. When we suggest SageMaker to clients, they don't have to go through all the steps manually. They can leverage Autopilot to choose variables, run experiments, and monitor costs. The results are also pretty accurate.
What needs improvement?
The training modules could be enhanced. We had to take in-person training to fully understand SageMaker, and while the trainers were great, I think more comprehensive online modules would be helpful.
Additionally, the user manuals can be difficult to navigate without prior knowledge. We often test new features for clients in small groups, and I've heard feedback that the documentation could be more user-friendly.
For how long have I used the solution?
I have been using it for around nine months.
What do I think about the stability of the solution?
I would rate the stability an eight out of ten. They could add features, which would be nice.
What do I think about the scalability of the solution?
Scalability is a great point for AWS. But then again, when it comes to manufacturing, it's about people in the plant. Sometimes, they don't use the product at all.
Even though it's popular and used by many companies, people tend to stick with other solutions. However, since Arain assumed your data center should be in-country, most people are now welcoming these cloud solutions.
The suitability of this solution's usage depends on the use case and the company size. If it involves a lot of variables and is difficult to manage manually, the tool is perfect.
How are customer service and support?
We get support from the Dubai guys. They come for training and provide any technical assistance needed. It's nice to have in-house support, so I'd rate them a nine out of ten.
How would you rate customer service and support?
Positive
Which solution did I use previously and why did I switch?
Our Infotech company comes under shared services. So, technically, we provide solutions for every other department.
Plus, we are working with AWS. Plus, we have, we have in-house tools that we develop. We also use Microsoft tools like Teams, Office, and SharePoint, as well as EDMS.
We also use SageMaker and Databricks.
How was the initial setup?
The initial setup was very simple for me. But for other people, so we set up the environment for people in here. So, technically, I don't think process people would have faced much challenge about that because we usually set it up, call them, and we share a screen, and we set it up for them. So it's all there.
Initially, when someone has no knowledge about that, it would be hard. If you don't know the AWS essentials, knowing the correct kind of storage might be a challenge.
Initially, deployment will take around maybe an hour. But after that, you just get used to it, so it is pretty much easy. Like, about 15 minutes, you're done, you're explaining.
For us, it's always on on-premise things, even better be a data lake or data warehouse or modeling or anything. So, going from, like, hardcore coding every line in bit fit and embedding it on your own. Having a feature like that is just a relief. So it took a lot of time because one of the popular manufacturing companies underwent a hack. And after that, most of the manufacturing companies don't promote cloud solutions.
Our data LAKE is on-prem. So, basically, we just move the modules that are required at that point in time and pull it out.
What's my experience with pricing, setup cost, and licensing?
There is room for improvement in the pricing. The pricing could be better, especially for querying. The per-query model feels expensive. It would be better to have tiered pricing based on query sets or usage. Some services definitely need pricing adjustments.
Which other solutions did I evaluate?
We tried Azure, and their tech support wasn't great. It took a long time for them to get back, and they might not have much regional coverage. I don't know if they have it, but AWS dominates the region, and most companies use it. When we were looking for solutions, we did some research, but the feedback for Azure wasn't positive.
What other advice do I have?
Overall, I would rate the solution a nine out of ten.
Which deployment model are you using for this solution?
On-premises
Disclosure: I am a real user, and this review is based on my own experience and opinions.
One-touch deployment and monitoring with customizable insights
Pros and Cons
- "One of the most valuable features of Amazon SageMaker for me is the one-touch deployment, which simplifies the process greatly."
- "The dashboard could be improved by including more features and providing more information about deployed models, their drift, performance, scaling, and customization options."
What is our primary use case?
Our primary use case is to build machine learning models and manage them using Amazon SageMaker. We use various tools provided by SageMaker, such as the studio for machine learning, pre-processing data using Wrangler, and deploying models. Some users prefer using Jupyter notebooks for their own libraries while others use features like Jumpstart or Autopilot.
What is most valuable?
One of the most valuable features of Amazon SageMaker for me is the one-touch deployment, which simplifies the process greatly. Additionally, I appreciate the flexibility that the notebook provides, as it allows me to experiment with scripts.
The solution offers the SageMaker Model Monitor, which helps monitor deployed models for performance issues like bias and drift. Also, the high scalability of SageMaker allows us not to worry about the underlying infrastructure, as it automatically adjusts based on demands.
What needs improvement?
The dashboard could be improved by including more features and providing more information about deployed models, their drift, performance, scaling, and customization options.
For how long have I used the solution?
I have been working with Amazon SageMaker for about three years.
What do I think about the stability of the solution?
The stability of the solution is generally about an eight out of ten. Most instabilities arise from initial configuration errors rather than the infrastructure itself. Ensuring that the correct setup is chosen from the start minimizes these issues.
What do I think about the scalability of the solution?
Amazon SageMaker is highly scalable, rated ten out of ten. It can scale up according to the demands detected by CloudWatch, providing a seamless experience without needing to manage the underlying infrastructure.
How are customer service and support?
The customer service and support are rated as a five out of ten. The level of support depends on whether we are a premium AWS customer or not, with premium customers receiving better and more immediate support.
How would you rate customer service and support?
Neutral
How was the initial setup?
The initial setup of SageMaker can be challenging for beginners, rated as a six, but easier for those with a background in machine learning, rated as a nine out of ten. Experience with machine learning is crucial for a straightforward setup. Without it, understanding the roles of different features can be a stumbling block.
What's my experience with pricing, setup cost, and licensing?
Pricing is rated as a six, which is slightly more expensive compared to the budget yet adequate for the capabilities provided. On average, customers pay about $300,000 USD per month.
What other advice do I have?
On a scale from one to ten, where ten is the best, I rate Amazon SageMaker as a nine. For new users evaluating SageMaker, it is important to remember that it takes some learning, however, the solution is straightforward and beneficial. There are no special prerequisites except having an account.
Disclosure: My company has a business relationship with this vendor other than being a customer: consultant
Last updated: Nov 22, 2024
Flag as inappropriate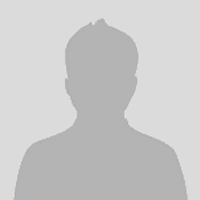
Buyer's Guide
Download our free Amazon SageMaker Report and get advice and tips from experienced pros
sharing their opinions.
Updated: April 2025
Popular Comparisons
Databricks
Microsoft Azure Machine Learning Studio
Alteryx
Dataiku
IBM SPSS Statistics
Altair RapidMiner
IBM Watson Studio
IBM SPSS Modeler
Anaconda
Domino Data Science Platform
Together Inference
Starburst Enterprise
Buyer's Guide
Download our free Amazon SageMaker Report and get advice and tips from experienced pros
sharing their opinions.
Quick Links
Learn More: Questions:
- How would you compare Databricks vs Amazon SageMaker?
- Is Microsoft Power BI capable to work with Amazon SageMaker ML models?
- What are the pros and cons of Amazon SageMaker vs Microsoft Azure Machine Learning Studio?
- Which are the best end-to-end data science platforms?
- What enterprise data analytics platform has the most powerful data visualization capabilities?
- What Data Science Platform is best suited to a large-scale enterprise?
- When evaluating Data Science Platforms, what aspect do you think is the most important to look for?
- How can ML platforms be used to improve business processes?
- Why is Data Science Platforms important for companies?