Feature Store, CodeCommit, versioning, model control, and CI/CD pipelines are the most valuable features in Amazon SageMaker.
Team lead at Assell
A fast solution that uses less code, but creating notebook instances for multiple users is pretty expensive
Pros and Cons
- "Feature Store, CodeCommit, versioning, model control, and CI/CD pipelines are the most valuable features in Amazon SageMaker."
- "Creating notebook instances for multiple users is pretty expensive in Amazon SageMaker."
What is most valuable?
What needs improvement?
Creating notebook instances for multiple users is pretty expensive in Amazon SageMaker.
For how long have I used the solution?
I have been using Amazon SageMaker for six months.
What do I think about the stability of the solution?
Amazon SageMaker is a stable solution.
Buyer's Guide
Amazon SageMaker
March 2025
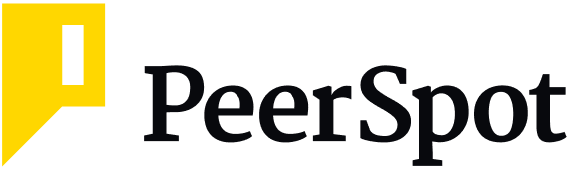
Learn what your peers think about Amazon SageMaker. Get advice and tips from experienced pros sharing their opinions. Updated: March 2025.
842,145 professionals have used our research since 2012.
What do I think about the scalability of the solution?
Amazon SageMaker is a scalable solution.
Which solution did I use previously and why did I switch?
I have previously worked with Google Cloud Platform (GCP). We first moved from GCP to Databricks because it was cost-efficient. Now, we are moving from Databricks to Amazon SageMaker to see whether it serves our purpose and is beneficial with respect to cost and time.
How was the initial setup?
The solution’s initial setup was straightforward. On a scale from one to ten, where one is difficult, and ten is easy, I rate Amazon SageMaker a six out of ten for the ease of its initial setup.
What about the implementation team?
The solution's initial deployment was a one-week job, but the final pipeline run was 12 to 15 hours.
What's my experience with pricing, setup cost, and licensing?
Creating notebook instances for multiple users is pretty expensive in Amazon SageMaker, but its storage is cheap.
On a scale from one to ten, where one is cheap, and ten is expensive, I rate the solution's pricing a six out of ten.
What other advice do I have?
I am doing a benchmarking study between Databricks and Amazon SageMaker to determine the most cost-efficient and effective for our organization.
Amazon SageMaker is a pretty good solution for users who don't have any knowledge about their data and want to try different scenarios. The solution is fast and uses less code.
Overall, I rate Amazon SageMaker a seven out of ten.
Which deployment model are you using for this solution?
Public Cloud
If public cloud, private cloud, or hybrid cloud, which cloud provider do you use?
Amazon Web Services (AWS)
Disclosure: I am a real user, and this review is based on my own experience and opinions.
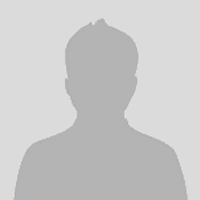
Big Data Solution Architect - Spatial Data Specialist at SCIERA, INC
A reasonably priced solution offering various models for its users to leverage from, along with an easy deployment process
Pros and Cons
- "The solution's ability to improve work at my organization stems from the ensemble model and a combination of various models it provides."
- "In general, improvements are needed on the performance side of the product's graphical user interface-related area since it consumes a lot of time for a user."
What is our primary use case?
My company uses Amazon SageMaker since we are into data analytics involved in predictions and focusing on various model executions, working with some top companies. Most of the use cases of the solution for my company stem from the fact that we need to understand various customer chain models, including customer retention or customer acquisition models, to leverage more revenue. Sometimes, the solution functions in batch mode or real-time mode. In case a customer contacts an IVR agent or the customer support team for help, we do modeling in real-time and deliver to Amazon SageMaker endpoint, ensuring how the robotics part responds to the queries of the customer.
How has it helped my organization?
The solution's ability to improve work at my organization stems from the ensemble model and a combination of various models it provides. It is important to note that since the ensemble model has limitations, it takes more time to process.
What is most valuable?
The most valuable feature of the solution is Amazon SageMaker Canvas. The training and algorithm-based XGBoost modeling make it a good product for a startup, especially for companies that want to explore something but don't have a proper model. The instrument will be helpful for those who want to explore something.
What needs improvement?
Amazon SageMaker should concentrate and get the performance of the ensemble model to be good enough for its users.
Improvements are needed in terms of performance for not all but some of the models, especially whenever we use the product for image classification or something. In general, improvements are needed on the performance side of the product's graphical user interface-related area since it consumes a lot of time for a user.
For how long have I used the solution?
I have been using Amazon SageMaker for four to five years. My company is a customer of AWS, and we have an advanced technology partnership with Amazon.
What do I think about the stability of the solution?
Stability-wise, I rate the solution a nine out of ten.
What do I think about the scalability of the solution?
Scalability-wise, I rate the solution an eight out of ten.
Around 20 to 25 people in my company use Amazon SageMaker.
How are customer service and support?
The technical support for the solution is good, but it is a paid service. The technical support for troubleshooting issues is chargeable, so ten percent of AWS billing will be the cost for technical support. I rate the solution's technical support an eight out of ten.
How would you rate customer service and support?
Positive
Which solution did I use previously and why did I switch?
Along with Amazon SageMaker, I use other services from AWS, like AWS Glue, Athena, Redshift, SQS, SNS, and Airflow.
How was the initial setup?
On a scale of one to ten, where one is a difficult setup, and ten is an easy setup, I rate the setup phase a nine.
The solution is deployed on the cloud.
The deployment phase takes around 15 to 20 minutes since the product has good integration capabilities with other platforms like Jenkins and Terraform.
Our company uses Jenkins pipeline and Bitbucket for the deployment process. Everything is moved from CodeCommit to Bitbucket, after which the Jenkins pipeline takes it from Bitbucket and deploys it to SageMaker. We can do the deployment in the cloud as well, but we do it with Bitbucket and Jenkins since they allow for good integration with Amazon SageMaker, which is also easy for us to make it move.
We have a team consisting of solution and database architects in which, most of them are AWS-certified individuals capable of carrying out troubleshooting procedures in case of issues who take care of the solution's deployment process in our company.
What's my experience with pricing, setup cost, and licensing?
I rate the pricing a five on a scale of one to ten, where one is the lowest price, and ten is the highest price. The solution is priced reasonably. There is no additional cost to be paid in excess of the standard licensing fees.
What other advice do I have?
From an exploration perspective, for people who cannot afford hardware at the physical location, it would be good to use the services from a cloud for leverage. It is easy to scale up or down when operating on an AWS Cloud. Suppose we have an on-premises or hybrid solution. In that case, we need to look at the economic structure of the organization, after which bringing everything into a physical location can get really complex. I suggest others explore using AWS before deciding on future plans.
I rate the overall solution a nine out of ten.
Disclosure: My company has a business relationship with this vendor other than being a customer:
Buyer's Guide
Amazon SageMaker
March 2025
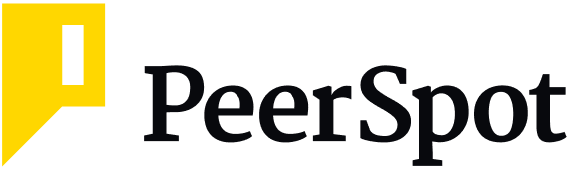
Learn what your peers think about Amazon SageMaker. Get advice and tips from experienced pros sharing their opinions. Updated: March 2025.
842,145 professionals have used our research since 2012.
Cloud Architect & Support Service Delivery Manager at Almoayyed Computers
Straightforward setup, scalable, and the technical support is good
Pros and Cons
- "The most valuable feature of Amazon SageMaker is that you don't have to do any programming in order to perform some of your use cases."
- "AI is a new area and AWS needs to have an internship training program available."
What is our primary use case?
We are a solution provider that is concentrating on migrating our customers from on-premises to the cloud, and Amazon SageMaker is one of the products that we implement for our customers.
SageMaker is an AI platform, and I have been working on creating a solution that uses SageMaker and DeepLens to recognize people for access control. It will automatically log people who are coming and leaving. The second use case that we are working on is a system that recognizes cars by reading license plates and then opening a gate automatically to let them into the parking area.
AI, in general, has not yet been heavily used in this region so I am working on three or four use cases.
What is most valuable?
The most valuable feature of Amazon SageMaker is that you don't have to do any programming in order to perform some of your use cases. As it is, we can start to use it directly.
What needs improvement?
AI is a new area and AWS needs to have an internship training program available. This is one place where I see this solution lagging. There is high-level training available, but when you consider that people have been working with Windows, Linux, and various applications for the past 20 years, they know those products inside and out. SageMaker, on the other hand, is a completely new tool. It can be very hard to digest.
AWS needs to provide more use cases for SageMaker. There are some, but not enough. They should collect or create more use cases and then distribute them free of charge to the customers.
I would like to see a more graphical, low-code interface that can be used to customize SageMaker.
For how long have I used the solution?
We have just begun to provide services using SageMaker.
What do I think about the scalability of the solution?
This solution is completely scalable.
How are customer service and technical support?
I have been in contact with Amazon technical support in the past, but not for SageMaker. I have between 50 and 70 customers and I have worked with Amazon support on multiple cases. I am quite happy with it. It is not expensive and the service is great.
The value you get for paying from Amazon support is great. They are ready to work with me to resolve my issues.
How was the initial setup?
The initial setup is straightforward. People with level-one training can start using it.
It usually takes about one hour to deploy, although the length of time and the number of people required are dependent on the complexity of the use cases and the environment.
What's my experience with pricing, setup cost, and licensing?
The business support costs 10% of the Amazon utility spend
What other advice do I have?
Myself and certain people in my team have just begun the training. There is an eight-hour training video to assist with learning how to use this solution.
I would rate this solution an eight out of ten.
Which deployment model are you using for this solution?
Public Cloud
If public cloud, private cloud, or hybrid cloud, which cloud provider do you use?
Amazon Web Services (AWS)
Disclosure: My company has a business relationship with this vendor other than being a customer: Reseller
Solutions Architect, ML Engineer, MLOps at a computer software company with 1-10 employees
Efficient experiment design through integrated infrastructure and reasonable pricing
Pros and Cons
- "The most valuable features are the ability to store artifacts and gather reports and measures from experiments."
- "The model repository is a concern as models are stored on a bucket and there's an issue with versioning."
What is our primary use case?
I use Amazon SageMaker primarily for designing and performing experiments with recommendation systems. It's mainly about classification, as most recommendation systems are based on classification.
How has it helped my organization?
It is crucial for the design and execution of multiple experiments concurrently, allowing me to concentrate on model design. I can use SageMaker for hyperparameter optimization and eventually for deploying models to production.
What is most valuable?
The most valuable features are the ability to store artifacts and gather reports and measures from experiments. Additionally, the integration with AWS infrastructure and the capability to create a hybrid grid infrastructure with scaling are important.
What needs improvement?
The model repository is a concern as models are stored on a bucket and there's an issue with versioning. Also, being unable to create routing other than based on TCP/IP protocols and HTTP poses limitations.
For how long have I used the solution?
I have been working with Amazon SageMaker for close to three years.
What do I think about the stability of the solution?
I find the performance of SageMaker stable, especially when I use it with a Kubernetes cluster. There have been no significant issues noted.
What do I think about the scalability of the solution?
It is scalable, particularly with the AWS infrastructure, including clusters and scheduling solutions like Airflow.
How are customer service and support?
I rarely use customer support since the platform is stable. Any issues, though rare, seem to be resolved well.
How would you rate customer service and support?
Positive
Which solution did I use previously and why did I switch?
I have worked with other AI development platforms, such as Kubeflow and MLflow, as separate platforms.
How was the initial setup?
The initial setup is straightforward if you have basic knowledge related to AWS Cloud and its infrastructure.
What's my experience with pricing, setup cost, and licensing?
The pricing is reasonable from my point of view, but it depends on factors like project size, potential income, data size, and user number.
Which other solutions did I evaluate?
I have experience with other AI development platforms, including Kubeflow and MLflow.
What other advice do I have?
I suggest properly sizing your project to manage potential costs effectively. If the project is small, starting with something simpler and less expensive may be better.
I'd rate the solution nine out of ten.
Which deployment model are you using for this solution?
Public Cloud
If public cloud, private cloud, or hybrid cloud, which cloud provider do you use?
Amazon Web Services (AWS)
Disclosure: I am a real user, and this review is based on my own experience and opinions.
Last updated: Oct 8, 2024
Flag as inappropriate,student at a university with 11-50 employees
With a great support team, the product's initial setup phase and configuration process are easy
Pros and Cons
- "I have contacted the solution's technical support, and they were really good. I rate the technical support a ten out of ten."
- "The payment and monitoring metrics are a bit confusing not only for Amazon SageMaker but also for the range of other products that fall under AWS, especially for a new user of the product."
What is our primary use case?
I use the solution since it is good. I have no issues with the solution as it suits my needs. Amazon SageMaker was used in our company to train an ML model. One of the trainers in our organization used Amazon SageMaker to train an ML model. I haven't had the opportunity to use products other than Amazon SageMaker. I am satisfied with Amazon SageMaker.
What needs improvement?
I feel that the area around the interface in AWS is overall confusing. The payment and monitoring metrics are a bit confusing not only for Amazon SageMaker but also for the range of other products that fall under AWS, especially for a new user of the product. The tool is not simplified enough for beginners to use. From an improvement perspective, the tool needs to be simplified enough for beginners to use.
For how long have I used the solution?
I have used Amazon SageMaker once or twice in the last six months. My company operates as a system integrator for Amazon.
What do I think about the stability of the solution?
Stability-wise, I rate the solution an eight to eight and a half out of ten.
What do I think about the scalability of the solution?
It is a scalable solution. Scalability-wise, I rate the solution an eight out of ten.
The number of uses of Amazon SageMaker varies from project to project. For most of the projects, the employees in our company depend on Azure platforms. Based on requests from our company's clients, we use Amazon SageMaker. Presently, five or six teams in our company use Amazon SageMaker.
How are customer service and support?
I have contacted the solution's technical support, and they were really good. I rate the technical support a ten out of ten.
How would you rate customer service and support?
Positive
How was the initial setup?
The product's initial setup phase and configuration were easy.
The product's installation phase requires two people.
The solution can be deployed in a few hours.
What about the implementation team?
I and one of the trainers in our company were involved in the installation process of the product.
What was our ROI?
As the product does the job for what is required in our organization, I feel that it saves time.
What's my experience with pricing, setup cost, and licensing?
Amazon SageMaker is a very expensive product. There is a need to make monthly payments towards the licensing cost attached to the solution. Even though I had initially used Amazon SageMaker's free trial version, Amazon charged me 130 USD for two to three days of usage. There are no extra charges to be paid apart from the resources that users use.
What other advice do I have?
I have not integrated Amazon SageMaker with other products in our company.
If someone plans to use the free trial version of Amazon SageMaker, then the person should be aware that it is chargeable since Amazon has not mentioned it in a written format. For enterprise-level users, there is nothing to worry about since their organization will take care of the costs attached to the solution.
I rate the overall tool an eight out of ten.
Disclosure: My company has a business relationship with this vendor other than being a customer: Integrator
Lead Technical Product Owner - AI & ML at a transportation company with 10,001+ employees
Stable solution that's worth the money but lacks reporting services
Pros and Cons
- "We've had no problems with SageMaker's stability."
- "SageMaker would be improved with the addition of reporting services."
What is our primary use case?
I mainly use SageMaker for deploying, using, and running our models.
What needs improvement?
SageMaker would be improved with the addition of reporting services. In addition, the models available in SageMaker are not enough for most of our use cases and require customization to be useful.
For how long have I used the solution?
I've been using SageMaker for six to eight months.
What do I think about the stability of the solution?
We've had no problems with SageMaker's stability.
How are customer service and support?
We are premium partners with AWS, so we have complete 24/7 support from them.
How was the initial setup?
The initial setup was very easy and quick, though deploying using cloud formation templates was difficult for us.
What about the implementation team?
We used an in-house team.
What's my experience with pricing, setup cost, and licensing?
SageMaker is worth the money for our use case.
What other advice do I have?
I would give SageMaker a rating of six out of ten.
Which deployment model are you using for this solution?
Public Cloud
Disclosure: My company has a business relationship with this vendor other than being a customer: partner
Machine Learning Specialist at Hubtel
The product enables users to build and deploy machine learning models, but the documentation must be made more user-friendly
Pros and Cons
- "The product aggregates everything we need to build and deploy machine learning models in one place."
- "The documentation must be made clearer and more user-friendly."
What is our primary use case?
I use the solution to build machine learning models and deploy them on endpoints.
How has it helped my organization?
The cost of managing our organization’s infrastructure is just too much. It’s easier to use AWS.
What is most valuable?
The product aggregates everything we need to build and deploy machine learning models in one place. We log in to the cloud and have everything we need to build and deploy models.
What needs improvement?
The product must improve its documentation. The documentation must be made clearer and more user-friendly. Sometimes, we run into issues with setup. However, it's not that often.
For how long have I used the solution?
I have been using the solution for about two years.
What do I think about the stability of the solution?
The stability is solid. I've been using the tool for a while. I haven't had major issues with it.
What do I think about the scalability of the solution?
We have 600 people in our organization. More than 300 people are developers. Everybody uses AWS. The tool is very scalable. I rate the scalability a nine out of ten.
How are customer service and support?
I had an issue logging into my AWS account. So I contacted the support persons. They were helpful.
How would you rate customer service and support?
Positive
How was the initial setup?
The solution is deployed on the cloud. We do not manually install the solution on our machine. We do have a CLI on our machine.
What's my experience with pricing, setup cost, and licensing?
The solution is relatively cheaper. Azure might be a little cheaper than AWS. However, AWS has all the services we need in one place.
What other advice do I have?
The solution works. It’s better than most of the other options available. We go through a long process to get the model in the hands of the users. SageMaker caters to all the processes involved with pre-built services. It makes the whole process very easy. Sometimes, the cost can be an issue, but it has all the right services we need. It is very smooth. Overall, I rate the solution a seven out of ten.
Disclosure: I am a real user, and this review is based on my own experience and opinions.
Solutions Architect at Emids
Helps to extract text from documents, images, and PDFs
Pros and Cons
- "The tool has made client management easier where patients need to upload their health records and we can use the tool to understand details on treatment date, amount, etc."
- "The solution needs to be cheaper since it now charges per document for extraction."
What is our primary use case?
We use the solution as an OCR to extract text from documents, images, PDFs, etc.
How has it helped my organization?
The tool has made client management easier where patients need to upload their health records and we can use the tool to understand details on treatment date, amount, etc.
What is most valuable?
I am impressed with the tool's text extraction and its accuracy.
What needs improvement?
The solution needs to be cheaper since it now charges per document for extraction.
For how long have I used the solution?
I have been using the solution for five years.
What do I think about the stability of the solution?
I would rate the tool's stability a nine out of ten.
What do I think about the scalability of the solution?
I would rate the tool's scalability a nine out of ten and we use it once a week.
How are customer service and support?
The support's response to tickets is slow.
How would you rate customer service and support?
Neutral
How was the initial setup?
I would rate the product's deployment a nine out of ten since it's straightforward. The deployment gets completed within 20 minutes. You need one IT person and a developer to handle the deployment and maintenance.
What was our ROI?
We have seen ROI with the tool's use.
What's my experience with pricing, setup cost, and licensing?
I would rate the solution's price a ten out of ten since it is very high.
What other advice do I have?
I recommend the tool for document processing and would rate it an eight out of ten.
Disclosure: I am a real user, and this review is based on my own experience and opinions.
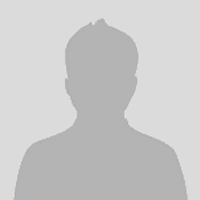
Buyer's Guide
Download our free Amazon SageMaker Report and get advice and tips from experienced pros
sharing their opinions.
Updated: March 2025
Popular Comparisons
Databricks
Microsoft Azure Machine Learning Studio
Alteryx
Dataiku
IBM SPSS Statistics
Altair RapidMiner
IBM Watson Studio
IBM SPSS Modeler
Anaconda
Domino Data Science Platform
Together Inference
Starburst Enterprise
Buyer's Guide
Download our free Amazon SageMaker Report and get advice and tips from experienced pros
sharing their opinions.
Quick Links
Learn More: Questions:
- How would you compare Databricks vs Amazon SageMaker?
- Is Microsoft Power BI capable to work with Amazon SageMaker ML models?
- What are the pros and cons of Amazon SageMaker vs Microsoft Azure Machine Learning Studio?
- Which are the best end-to-end data science platforms?
- What enterprise data analytics platform has the most powerful data visualization capabilities?
- What Data Science Platform is best suited to a large-scale enterprise?
- When evaluating Data Science Platforms, what aspect do you think is the most important to look for?
- How can ML platforms be used to improve business processes?
- Why is Data Science Platforms important for companies?